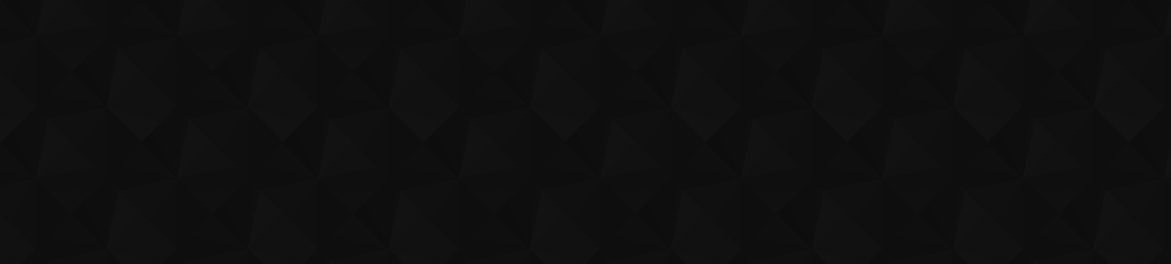
- 49
- 426 196
Andy McDonald
United Kingdom
เข้าร่วมเมื่อ 31 พ.ค. 2021
#petrophysics #python #matplotlib #geoscience
Creating Waffle Chart Subplots With Matplotlib - Easy Data Visualisation for Geoscience
This video follows my previous video where we created a basic waffle chart using PyWaffle. In this video, I share how you can quickly and easily display waffle charts as subplots in matplotlib, which can make it easier to understand the variances between different categories.
⭐️ If you haven't already, make sure you subscribe to the channel: th-cam.com/channels/n1O_4_ApzbYwrsUdRoMmOg.html
▼ ---- SUPPORT THE CHANNEL ---- ▼
☕️ BUY ME A COFFEE: www.buymeacoffee.com/andymcdonaldgeo
▼ ---- SOCIAL CHANNELS ---- ▼
Thanks for watching, if you want to connect you can find me at the links below:
andymcdonaldgeo.medium.com/
www.linkedin.com/in/andymcdonaldgeo/
www.andymcdonald.scot/
#datascience #petrophysics #python #datavisualization #eda
⭐️ If you haven't already, make sure you subscribe to the channel: th-cam.com/channels/n1O_4_ApzbYwrsUdRoMmOg.html
▼ ---- SUPPORT THE CHANNEL ---- ▼
☕️ BUY ME A COFFEE: www.buymeacoffee.com/andymcdonaldgeo
▼ ---- SOCIAL CHANNELS ---- ▼
Thanks for watching, if you want to connect you can find me at the links below:
andymcdonaldgeo.medium.com/
www.linkedin.com/in/andymcdonaldgeo/
www.andymcdonald.scot/
#datascience #petrophysics #python #datavisualization #eda
มุมมอง: 427
วีดีโอ
Creating Waffle Charts With Matplotlib and PyWaffle
มุมมอง 7677 หลายเดือนก่อน
Waffle charts are a great way to visualise categorical data, are aesthetically pleasing and easy for readers to understand - which is one of the key goals of effective data visualisations. They also provide a nicer looking alternative to pie charts. Waffle charts are square or rectangular displays made up of smaller squares in a grid pattern. Most commonly, it is a 10 x 10 grid, but they can be...
Styling Your Matplotlib Figures With a Cyberpunk Theme
มุมมอง 1.1K7 หลายเดือนก่อน
When we create infographics or posters containing data, we want to catch the reader’s attention and make it aesthetically pleasing to look at whilst telling a convincing story. Within Python, we have numerous plotting libraries that allow us to create charts - one such library is the well-known matplotlib library. However, out of the box, the plots generated by matplotlib are often seen as bori...
Displaying Maps With Plotly Express Mapbox and Streamlit
มุมมอง 2.5K7 หลายเดือนก่อน
Streamlit provides a quick and easy way to build interactive applications and dashboards for data analysis and machine learning. If we are looking to build a data analysis app within Streamlit that uses data containing location information, one of the first visualisations we may want to consider adding is a map. Having an interactive map within our app allows us to visualise where the data poin...
Creating Geospatial Heatmaps With Plotly Express MapBox and Folium in Python - Data Visualisation
มุมมอง 2.9K8 หลายเดือนก่อน
Heatmaps, also known as Density Maps, are data visualisations that display the spatial distribution of a variable across a geographic area. They can be great tools for visualising and identifying trends, supporting decision-making, detecting outliers, and creating compelling visualisations for presentations. There are several mapping Python libraries available; however, two very popular and eas...
Pandas Dataframes - Data Aggregation Using Geological Lithology Data
มุมมอง 4408 หลายเดือนก่อน
Using data aggregation techniques can help us transform an overwhelming and almost incomprehensible numeric dataset into something that is easily digestible and much more reader-friendly. The process of data aggregation involves summarising multiple data points into single metrics that can be used to provide a high-level overview of the data. One way we can apply this process within petrophysic...
How To Make Your Matplotlib Bar Charts Stand Out
มุมมอง 1.4K8 หลายเดือนก่อน
Bar charts are a commonly used data visualisation tool where categorical features are represented by bars of varying lengths/heights. The height or length of the bar corresponds to the value being represented for that category. Bar charts can easily be created in matplotlib. However, the matplotlib library is often regarded as a library that produces unexciting charts and can be challenging to ...
PyGWalker for Exploratory Data Analysis In Jupyter Notebooks
มุมมอง 12Kปีที่แล้ว
PyGWalker (Python binding of Graphic Walker) is a python library that can help speed up the data analysis and visualisation workflow directly within a Jupyter notebook. It leverages the power of interactivity by providing an interface similar to the popular data analytics software called Tableau. This video will explore some of the features of PyGWalker using one of my favourite well log data s...
Isolation Forest for Outlier Detection within Python
มุมมอง 25Kปีที่แล้ว
Isolation Forest is a popular unsupervised machine learning algorithm for detecting anomalies (outliers) within datasets. Anomaly detection is a crucial part of any machine learning and data science workflow. Erroneous values that are not identified early on can result in inaccurate predictions from machine learning models, and therefore impact the interpretation of those results. ⭐️ If you hav...
Working With Well Survey Data in Python Using wellpathpy
มุมมอง 2.3Kปีที่แล้ว
Depth is an essential measurement when working with subsurface data. It is used to tie multiple sets of data to a single reference. There are numerous depth references used to identify a position within the subsurface. These include Measured Depth (MD), True Vertical Depth (TVD), and True Vertical Depth Subsea (TVDSS). When wells are drilled, survey measurements are often taken to ensure that t...
Combining Well Log Data With Formation Tops in Python for Petrophysics
มุมมอง 2.8Kปีที่แล้ว
When working with well log data we often have to deal with different data sources and sampling rates. One area where we commonly experience this is with well log data and formation tops. Formation top data contains a formation name along with a single depth reference, whereas well log data is regularly depth sampled. In this video I will go over the process on how to create a master dataframe w...
Random Forest Regression Machine Learning - Well Log Prediction for Petrophysics
มุมมอง 3.5Kปีที่แล้ว
Random forest is a very popular machine learning algorithm that can be used for both classification and regression. Within this tutorial, we will see how we can use the Random Forest algorithm to predict a continuous output using well logs as an example. ⭐️ If you haven't already, make sure you subscribe to the channel: th-cam.com/channels/n1O_4_ApzbYwrsUdRoMmOg.html ▼ SUPPORT THE CHANNEL ▼ ☕️ ...
Porosity Permeability (Poro-Perm) Log-Linear Regression in Python - Petrophysics
มุมมอง 2.7Kปีที่แล้ว
Permeability is one of the key reservoir properties we as petrophysicists attempt to derive as part of our workflow. As well logging tools do not provide a direct measurement for permeability, we have to infer it through relationships with core data from the same field or well, from empirically derived equations or NMR data. One common method of deriving permeability is to plot core porosity (o...
Seaborn Heatmap - How to Visualise Correlations and Data With Heatmaps in Python
มุมมอง 31Kปีที่แล้ว
Heatmaps are a great way to visualise tabular data. They allow us to identify trends, spot outliers and understand the range of our data. In this week's video, we are going to see how to visualise data using a Seaborn Heatmap. ⭐️ If you haven't already, make sure you subscribe to the channel: th-cam.com/channels/n1O_4_ApzbYwrsUdRoMmOg.html ▼ SUPPORT THE CHANNEL ▼ ☕️ BUY ME A COFFEE: www.buymeac...
Seaborn Pairplot - How to Create a Pairplot for Data Visualization in Python Using Seaborn
มุมมอง 6Kปีที่แล้ว
The Seaborn pairplot allows us to easily take a dataframe and generate a single figure showing the relationships between all of the variables and their distributions. In this week's video we will see how we can quickly create a pairplot using the Seaborn library in Python ⭐️ If you haven't already, make sure you subscribe to the channel: th-cam.com/channels/n1O_4_ApzbYwrsUdRoMmOg.html I have al...
Documenting Your Code with Python - Overview of Comments, Docstrings and Type Hints
มุมมอง 2.2Kปีที่แล้ว
Documenting Your Code with Python - Overview of Comments, Docstrings and Type Hints
Random Forest Machine Learning Tutorial in Python for Lithology Prediction - Includes Overview
มุมมอง 6K2 ปีที่แล้ว
Random Forest Machine Learning Tutorial in Python for Lithology Prediction - Includes Overview
New Streamlit Multi-Page Web Apps - Converting Existing Apps
มุมมอง 11K2 ปีที่แล้ว
New Streamlit Multi-Page Web Apps - Converting Existing Apps
Creating Multiple Subplots the Easy Way - Seaborn FacetGrid Introduction
มุมมอง 5K2 ปีที่แล้ว
Creating Multiple Subplots the Easy Way - Seaborn FacetGrid Introduction
Seaborn Relplot - Create Scatter Plots and Line Plots in Python
มุมมอง 1.7K2 ปีที่แล้ว
Seaborn Relplot - Create Scatter Plots and Line Plots in Python
Create Semi Log Scatter Plots in Python - Display Data on a Logarithmic Axis in Seaborn
มุมมอง 2.2K2 ปีที่แล้ว
Create Semi Log Scatter Plots in Python - Display Data on a Logarithmic Axis in Seaborn
Adding Interactive Plotly Charts to a Streamlit App
มุมมอง 22K2 ปีที่แล้ว
Adding Interactive Plotly Charts to a Streamlit App
Creating Multi-Page Streamlit Apps | Python Streamlit Series Part 2
มุมมอง 13K2 ปีที่แล้ว
Creating Multi-Page Streamlit Apps | Python Streamlit Series Part 2
Getting Started With Streamlit in Python
มุมมอง 26K2 ปีที่แล้ว
Getting Started With Streamlit in Python
Fast and Effective Exploratory Data Analysis (EDA) With Python and Pandas Profiling for Data Science
มุมมอง 6K2 ปีที่แล้ว
Fast and Effective Exploratory Data Analysis (EDA) With Python and Pandas Profiling for Data Science
Data Quality Considerations for Petrophysical Machine Learning Models
มุมมอง 1.8K2 ปีที่แล้ว
Data Quality Considerations for Petrophysical Machine Learning Models
CSV to LAS with Python and LASIO for Well Log Data
มุมมอง 3.1K2 ปีที่แล้ว
CSV to LAS with Python and LASIO for Well Log Data
Free Well Logging & Petrophysics Datasets for Data Science and Machine Learning
มุมมอง 7K2 ปีที่แล้ว
Free Well Logging & Petrophysics Datasets for Data Science and Machine Learning
6 Essential Python Libraries for Well Log Data
มุมมอง 3K2 ปีที่แล้ว
6 Essential Python Libraries for Well Log Data
Python Functions - Application of Functions to a Petrophysical Equation
มุมมอง 1.1K2 ปีที่แล้ว
Python Functions - Application of Functions to a Petrophysical Equation
You are a hero!
Hi Andy - Can you please share this dataset ? I have not been able to find it online
Thanks Andy. Nice series of videos that certainly helped me get started with Streamlit. Have you considered a video of adding the ability to trigger events from plotly charts? For example, clicking and item on a plotly chart to filter a dataframe that powers another plotly chart? This is something I think would be useful to understand to create truly interactive dashboards with Streamlit
Thanks. It is something I have thought about and I’m hoping to share a video on it in the future
@@AndyMcDonald42 awesome thank you!
baik bgt pa membantu ujian saya
nice explanation👍
Great presentation. The clearest I've seen on TH-cam, to date. 👍
sir, how to clustering data 2d with size(512,512), please help me sir tq
Do you know how to set the x-axis range for the log plots to show a little padding? I used fig.update_xaxes(range=[min, max]) but it sets the x-axis range to the same values for all three plots even though I did this for all three subplots separately.
No worries, I figured it out right after posting. It was standard "user error" on my part. range=[min, max] works as advertised when implemented properly.
Another great video. How do you decide when to use Jupyter Notebook & when to use a more traditional code editor?
Great! Appreciate your videos. Small correction: Kansas Geological SURVEY (not Society).
sir can u plz make vedio about the data preprocessing like how to convert the dates from dat format to csv and csv to las file and how to combine the readings of the same well and different well
Amazing! Thanks ;D
Thank you!
Great
excellent content and explanation about streamlit... Gracias!!!
nicer😎
This map can work in offline environments?
Thanks for this knowledge
Thanks, Andy!!! Even knowing bar plotting pretty well, I learn at least one or two great trick in your videos. I appreciate your videos and recommend them to colleagues very often. Cheers!!!
Thank You so much Andy.
Very informative video ...just a suggestion , Subtitles should be made available as your accent is pretty strong for non english speakers to understand
A masterpiece video! makes it easy to grasp the concept
Hi Andy, this was a great tutorial as it's something I would like to try on a csv file with various metrics in the design of a pharmaceutical. I have one question though: I will be wanting to use 5-7 columns on the csv file for clustering - how do you go about visually representing this? I can't think of a good way to do it. Thanks!
Great one, how do you get the p values out?
Do you know why I get a 403 error when I try to upload the csv?
these are the best tutorials for streamlit I have found! Thanks a lot!
Hello Andy, thanks for the great tutorial. I have a question, do you know how to create/calculate the trend log such as DT Sonic trend line (Normal Compaction Trend)? And how to filter the spike log values (despike)?
Good morning sir, how can i use this random forest algorithm for LULC prediction using tiff files
please provide me, if you have any source code to do with raster files to predict future lulc classification
I can send you the data and the type of boxplot Im talkig about
thanks for the video please how can I get the Scatter Plots for two (y) axes Thank you for your time
el error es 'LASFile' object has no attribute 'add_curve'
ami me sale este error, alguien puede decirme como corregirlo
help me out alot than you!😀😀😀
Great video, Andy.. Explained so lucidly
Hi Andy. What's the difference between the CKHG and CKHL perm values? Can you shed some light? Cheers
Thank you for the video, I copied the steps and created the las file which looks the same as yours when opened in notepad. Should it not be a binary file? When I try to attach it in QGIS, i get and error'Could not load source layer for INPUT: path file not found. Am I supposed to do another step to read the file?
Lovely <3
Could you please share the link to get the dataset?
I find it very slow!
i liked it, had to hit that belllll
Where can I get the Data Xeek well file because I keep getting errors
Hello ! Is it possible to use this for corrected cover area (CCA) for overlap in an umbrella review?
These look great and I disagree, I'd definitely use these in a presentation (for physics). Are there any other good libraries like this?
Hi Andy! I have been trying to make a box plot with a simple break in y-axis and have not been able to. any tips?
Wow. I wish there's 3D visualization
Thank you very much! Extremely useful video!
Thanks for the explanation
Greetings Andy, I work in a company that won't give me an access to Python (lasio), and sometimes I face issues while dealing with wrapping las files trying to export them into excel, do you know of any way I can resolve this issue using Excel or MATLAB? Also, I can't download any additional add-ons or packages to either.
Thanks for information,The library is amazing!