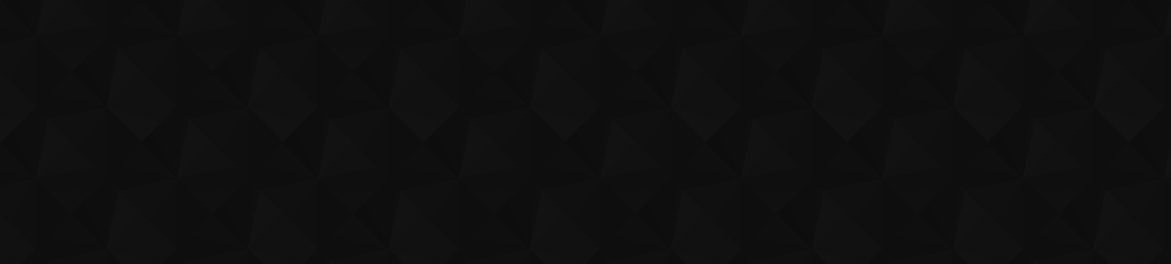
- 9
- 2 112
David Loc
เข้าร่วมเมื่อ 1 มิ.ย. 2019
What's Next
A Short 3DMODT Demonstration in a Crowded, Complex Environment
A Short Demo of 3DMODT on Phenikaa University Campus, You can see its hard to track pedestrians, as they can change direction unpredictably.
Method: PHE3D-MODT - ieeexplore.ieee.org/document/10738131
Dataset: PHE3D-X ~ complex dataset, up to 200 objects / frame
Tracking Targets: Index, 3D Box, Class, Frame Lost, Velocity, Yaw Angle
Motion Estimation: Next 10 seconds
Note: Please select 1920x1080 video quality for better visualization
Method: PHE3D-MODT - ieeexplore.ieee.org/document/10738131
Dataset: PHE3D-X ~ complex dataset, up to 200 objects / frame
Tracking Targets: Index, 3D Box, Class, Frame Lost, Velocity, Yaw Angle
Motion Estimation: Next 10 seconds
Note: Please select 1920x1080 video quality for better visualization
มุมมอง: 32
วีดีโอ
3D MODT via VLP 16 LIDAR on Vietnamese Streets
มุมมอง 3314 หลายเดือนก่อน
Qualitative Result of "Accurate 3D Multi-Object Detection and Tracking on Vietnamese Street Scenes Based on Sparse Point Cloud Data" Paper. ieeexplore.ieee.org/document/10738131 Method: PHE3D-MODT Sensor: Single LIDAR VLP-16 channel Inference Time: Up to 100 FPS for detection and up to 2000 FPS for tracking Hardware: GPU - RTX 3090, CPU - Intel i7-12K Dataset: Phenikaa-X (Vietnamese Street) Obj...
3DMODT + Clustering via VLP16 LIDAR
มุมมอง 1564 หลายเดือนก่อน
DEMO 3DMODT & Clustering on Phenikaa University Campus Method: Combined Phenikaa-X 3DMODT 3D Clustering Sensor: Using LIDAR VLP 16 channel only Range: [-300m, 300m]x[-300m, 300m]x[-3m, 4m]; effect range depend on sensor ranges. Inference Time: ±60 FPS for detection; ±2000 FPS for tracking; ±200 FPS for clustering Hardware: GPU - RTX 3090, and CPU - Intel i7-12K Objects: Vehicles, Pedestrians, R...
DEMO 3DMODT on Difficult Environment (Snow, and Heavy Rain)
มุมมอง 2328 หลายเดือนก่อน
DEMO 3DMODT on Difficult Environment (Snow, and Heavy Rain) - Method: Phenikaa-X 3DMODT - Sensor: LIDAR only - Range: [ -300m, 300m]*[ -300m, 300m]*[ -3m, 4m], effect ranges depend on sensor ranges. - Inference Time: 60 FPS on a single 3090 GPU Card. - Dataset: CADC - Objects: Vehicles, Pedestrians, and Riders - Tracking Targets ( Index, 3D Box, Class, Frame Lost, Velocity, and Yaw Angle)
How to deal 3DMODT with the WAYMO dataset
มุมมอง 33711 หลายเดือนก่อน
Demo 3D MODT on WAYMO dataset - Method: Phenikaa-X 3DMODT - Range: [ -75.2m, 75.2m]*[ -75.2m, 75.2m]*[ -3m, 4m] - Inference Time: 45 FPS on a single 3090 GPU Card. - Dataset: WAYMO - Objects: Vehicles, Pedestrians, Riders - Tracking Targets ( Index, 3D Box, Class, Frame Lost, Velocity, and Yaw Angle)
PHE-3D 3DMODT
มุมมอง 82ปีที่แล้ว
Demo 3D MODT on Phenikaa University Campus - Method: Phenikaa-X 3DMODT - Objects: Vehicles, Pedestrians, Riders - Tracking Targets ( Index, 3D Box, Class, Frame Lost, Velocity, and Yaw Angle)
Demo 3D MODT on Vietnamese Street
มุมมอง 377ปีที่แล้ว
Demo 3D MODT on Vietnamese Street - Method: Phenikaa-X 3DMODT - Inference Time: 100 FPS for only detection, 600 FPS for only tracking in a single 3090 GPU Card. - Dataset: Phenikaa-X (Vietnamese Street) - Objects: Vehicles, Pedestrians, Riders - Tracking Targets ( Index, 3D Box, Class, Frame Lost, Velocity, and Yaw Angle)
AEC3D - An Efficient and Compact Single-Stage 3D Multi-Object Detector For Autonomous Driving
มุมมอง 181ปีที่แล้ว
The implementation of AEC3D - An Efficient and Compact Single-Stage 3D Multi-Object Detector For Autonomous Driving ) Paper: ieeexplore.ieee.org/document/9861730 ) Method: AEC3D ) Dataset: Kitti Raw Dataset. ) Investigation Range: 360 view range (real driving scenarios), front view range (KITTI benchmark). ) Inference in x2 range calculate for the only front view (FPS)
Realtime 3D Multi-Object Detection and Tracking based on VLP16 LIDAR
มุมมอง 386ปีที่แล้ว
ROS Implementation of Realtime 3D Multi-Object Detection and Tracking based on VLP16 LIDAR - Method: Phenikaa-X 3DMODT - Inference Time: 100 FPS for only detection, 600 FPS (Initial), 2000 FPS (Now) for only tracking in a single 3090 GPU Card, and Intel I7 CPU, respectively - Dataset: Phenikaa-X (Concatination of 2 Velodyne 16 Channels) - Objects: Vehicles, Pedestrians, Riders - Tracking Target...
would you please share a medium such as GitHub, or LinkedIn so I can contact you?
Hi you can contact me via: lochd@phenikaa-x.com or www.linkedin.com/in/loc-hoang-duy-8ba054243/
Could you please share the code and implementation?
Hi, please take a look at the comment in this video: th-cam.com/video/ABwdtjL4v-U/w-d-xo.html
Awesome! Is the Phenikaa-X 3DMODT based on "3DMODT: Attention-Guided Affinities for Joint Detection & Tracking in 3D Point Clouds"?
Hi, thanks for being interested in my work, in this work, detection, and tracking work separately, so it is not based on the paper you mentioned above. I used a lightweight 3D voxel detection model + a mathematical base tracking model
@@loc_research If possible, could you please tell us about the method or paper on which it was based? Or is there any source code available?
@@yukihirosaito7783 Hi, actually in this work I researched and designed my own method based on the requirement of the company, so I can't tell you more details about that. But you can use 3D OD (PVRCNN, CasA, Centerpoint, SE-SSD,...) + 3D OT (Castrack, AB3MODT, CenterTrack, Mathematical based) to make the 3D MODT framework like the video above. (Centerpoint + AB3MODT should work, I tried it before).