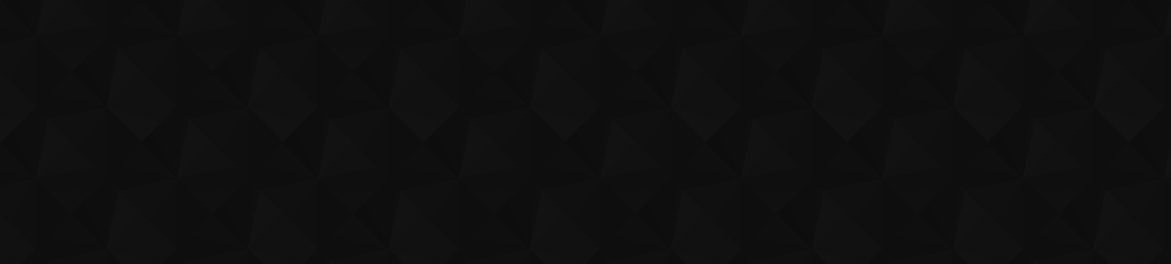
- 107
- 181 115
Climate Change AI
เข้าร่วมเมื่อ 14 พ.ย. 2019
Official channel of Climate Change AI (www.climatechange.ai), an initiative to facilitate cooperation and provide resources at the intersection of climate change and machine learning.
Discussion Seminar: GANs and sequential planning for resilient and sustainable buildings and cities
This is the November event in CCAI's Discussion Seminar Series.
This session included three different speakers from Future Cities Lab Global of Singapore-ETH Centre, a collaboration between ETH Zurich and Singaporean universities, all focusing on the intersection of Building and AI.
More details here: community.climatechange.ai/c/ccai-event-signup/ccai-discussion-seminar-series-4-generative-adversarial-networks-gans-and-sequential-planning-for-resilient-and-sustainable-buildings-and-cities
This session included three different speakers from Future Cities Lab Global of Singapore-ETH Centre, a collaboration between ETH Zurich and Singaporean universities, all focusing on the intersection of Building and AI.
More details here: community.climatechange.ai/c/ccai-event-signup/ccai-discussion-seminar-series-4-generative-adversarial-networks-gans-and-sequential-planning-for-resilient-and-sustainable-buildings-and-cities
มุมมอง: 447
วีดีโอ
AI for Climate Action: From Data to Impact - Climate Week NYC 2024
มุมมอง 6062 หลายเดือนก่อน
AI for Climate Action: From Data to Impact Organized by Climate Change AI and Google DeepMind Monday, September 23, 2024 @ Climate Week NYC Artificial intelligence (AI) is reshaping the way we act on climate change, across areas as diverse as energy, agriculture, biodiversity, and disaster response. However, fully realizing the potential of AI for climate action requires addressing critical gap...
Discussion Seminar: Multimodal AI Approaches for Urban Microclimate Prediction and Building Analysis
มุมมอง 2432 หลายเดือนก่อน
This is the October event in CCAI's Discussion Seminar Series. Kunihiko Fujiwara presented on "Microclimate Vision: Multimodal prediction of climatic parameters using street-level and satellite imagery" Binyu Lei presented on "Predicting building characteristics at urban scale using graph neural networks and street-level context" Xiucheng Liang presented on "Evaluating human perception of build...
Estimating Coal Power Plant Operation From Satellite Images with Computer Vision
มุมมอง 5013 หลายเดือนก่อน
This is the walkthrough video for the Estimating Coal Power Plant Operation From Satellite Images with Computer Vision tutorial. This tutorial can be accessed using the link below. www.climatechange.ai/tutorials?search=id:mrv-coal-power-plant&
Agile Modeling for Bioacoustic Monitoring
มุมมอง 4533 หลายเดือนก่อน
Agile Modeling for Bioacoustic Monitoring
Forecasting the El Nino/ Southern Oscillation with Machine Learning
มุมมอง 5433 หลายเดือนก่อน
This is the walkthrough video for the Forecasting the El Nino/ Southern Oscillation with Machine Learning tutorial. You can access the tutorial at the link below. www.climatechange.ai/tutorials?search=id:forecasting-the-el-nino-southern-oscillation-with-machine-learning&
Building Load Forecasting with Machine Learning
มุมมอง 6603 หลายเดือนก่อน
This is the walkthrough video for the Building Load Forecasting with Machine Learning tutorial. You can access this tutorial using the link below. www.climatechange.ai/tutorials?search=id:building-load-forecasting-with-machine-learning&
Reducing your Climate Impact when Training ML Models
มุมมอง 3473 หลายเดือนก่อน
This is the walkthrough video for the Reducing your Climate Impact when Training ML Models tutorial. You can access the tutorial at the link below. www.climatechange.ai/tutorials?search=id:reduce-climate-impact-when-training-ml-models&
Research Synthesis using NLP in the Field of Climate Change: Part 2
มุมมอง 4203 หลายเดือนก่อน
This is the walkthrough video for Part 2 of the Research Synthesis using NLP in the Field of Climate Change tutorial. You can access this tutorial using the link below.
Research Synthesis using NLP in the Field of Climate Change: Part 1
มุมมอง 5763 หลายเดือนก่อน
This is the walkthrough video for Part 1 of the Research Synthesis using NLP in the Field of Climate Change tutorial. You can access this tutorial using the link below. www.climatechange.ai/tutorials?search=id:nlp-climate-policy-part1&
Land Use and Land Cover Classification using Pytorch: Part 2
มุมมอง 7853 หลายเดือนก่อน
This is the walkthrough video for Part 2 of the Land Use and Land Cover Classification using Pytorch tutorial. You can access this tutorial using the link below. www.climatechange.ai/tutorials?search=id:land-use-and-land-cover-lulc-classification-using-deep-learning-part-i&
Land Use and Land Cover Classification using Pytorch: Part 1
มุมมอง 1.1K3 หลายเดือนก่อน
This is the walkthrough video for Part 1 of the Land Use and Land Cover Classification using Pytorch tutorial. You can access this tutorial using the link below. www.climatechange.ai/tutorials?search=id:land-use-and-land-cover-lulc-classification-using-deep-learning-part-i&
Predicting Mobility Demand from Urban Features Tutorial
มุมมอง 2453 หลายเดือนก่อน
This is the walkthrough video for the Predicting Mobility Demand from Urban Features tutorial. You can access the tutorial at the link below. www.climatechange.ai/tutorials?search=id:predicting-mobility-demand&
Building Control with RL using BOPTEST
มุมมอง 2573 หลายเดือนก่อน
This is the walkthrough video for the Building Control with RL using BOPTEST tutorial. You can access this tutorial using the link below. www.climatechange.ai/tutorials?search=id:building-control-with-rl-using-boptest&
Ethics, Impacts, and Regulation of AI
มุมมอง 1.1K3 หลายเดือนก่อน
Ethics, Impacts, and Regulation of AI
AI for Social Sciences, Economics and Policy, Part II
มุมมอง 1.1K3 หลายเดือนก่อน
AI for Social Sciences, Economics and Policy, Part II
AI for Monitoring, Reporting, and Verification
มุมมอง 1.6K3 หลายเดือนก่อน
AI for Monitoring, Reporting, and Verification
AI for Social Sciences, Economics and Policy, Part I
มุมมอง 1.8K3 หลายเดือนก่อน
AI for Social Sciences, Economics and Policy, Part I
AI for Agriculture, Forestry, and Other Land Use
มุมมอง 3.7K3 หลายเดือนก่อน
AI for Agriculture, Forestry, and Other Land Use
Tackling Climate Change with Machine Learning and Introduction to Machine Learning
มุมมอง 7K3 หลายเดือนก่อน
Tackling Climate Change with Machine Learning and Introduction to Machine Learning
Discussion Seminar: Beyond Green: Navigating Campus Sustainability with the CoolNUS-BEAM Initiative
มุมมอง 1564 หลายเดือนก่อน
Discussion Seminar: Beyond Green: Navigating Campus Sustainability with the CoolNUS-BEAM Initiative
Thanks for the forecast! Could you help me with something unrelated: I have a SafePal wallet with USDT, and I have the seed phrase. (alarm fetch churn bridge exercise tape speak race clerk couch crater letter). What's the best way to send them to Binance?
Prof. Philipp Hacker's briliantly researched contribution on the topic of Regulation of AI is so incredibly important (starts at 1h 4min). My request: separate the two lectures and place AI and Regulation prominently as a separate event. Thanks for this fantastic lecture. Sabine Wallstein, Munich
M
thanks for the valuable tutorial. is there any available link to access the PDF files? or they are not accessible?
The lectures were grate and comprehensive.
Thanks, I didn't know this summer school was happening
4092403 The second law of thermodynamics may be false conventional wisdom. Let's face the wonder of full heat use. The second law of thermodynamics was imposed on us during victorian england's scientific and religious fascination with steam engines. The second law is behind modern refrigerators needing electrical energy to compress the refrigerent to force it to release as waste the heat that it has removed from the refrigerator's service interior in the cooling part of the refrigerent's circulation. The interior coldness draws in exterior heat through the cabinet insulation. There is also discarded heat from mechanical friction and electrical resistance. The net thermal output equals the electrical input with energy not being gained or lost in this refrigeration system including its forced waste. Unencumbered refrigeration by the principle that energy is conserved should produce electricity instead of consuming it. It makes more sense that refrigerators should yield electricity because energy is widely known to change form with no ultimate path of energy gain or loss being found. Therefore any form of fully recyclable energy can be cycled endlessly in any quantity. In an extreme case senario, full heat recycling, all electric, very isolated underground, undersea, or space communities would be highly survivable with self sufficient EMP resistant LED light banks, automated vertical farms, thaw resistant frozen food storehouses, factories, dwellings, self contained elevators, safe rooms, and horizontal transports. In a flourishing civillization senario, small self sufficient electric or cooling devices of many kinds and styles like lamps, smartphones, hotplates, water heaters, cooler chests, fans, radios, TVs, cameras, security devices, robot test equipment, scales, transaction terminals, wall clocks, open or ciosed for business luminous signs, power hand tools, ditch diggers, pumps, and personal transports, would be available for immediate use incrementally anywhere as people as individuals or larger social groups see fit. Some equipment groups could be consolidated on local networks. If a high majority thinks our civilization should geoengineer gigatons or teratons of carbon dioxide out of our environment, instalations using devices that convert ambient heat into electricity can hypothetically be scaled up do it with a choice of comsequences including many beneficial ones. Energy sensible refrigerators that absorb heat and yield electricity would complement computers as computing consumes electricity and yields heat. Computing would be free. Chips could have energy recycling built in. A simple rectifier crystal can, iust short of a replicatable long term demonstration of a powerful prototype, almost certainly filter the random thermal motioren of electrons or discrete positiive charged voids called holes so the electric current flowing in one direction predominates. At low system voltage a filtrate of one polarity predominates only a little but there is always usable electrical power derived from the source, which is Johnson (observation) Nyquest (theory) thermal electrical noise. This net electrical filtrate can be aggregated in a group of separate diodes in consistent alignment parallel creating widely scalable electrical power. The maximum energy is converted from ambient heat to productive electricity when the electrical load is matched to the array impeadence. Matched impeadence output (watts) is k (Boltźman's constant), one point three eight x 10^ minus 23, times T (temperature Kelvin) times bandwidth (0 Hz to a natural limit ~2 THz @ 290 K) times rectification halving and nanowatt power level rectification efficiency, times the number of diodes in the array. For reference, there are a billion cells of 1000 square nanometer area each per square millimeter, 100 billion per square centimeter. Order is imposed on the random thermal motion of electrons by the structual orderlyness of a diode array made of diodes made within a slab: -----‐------‐----_____-- Out 🔻🔻🔻🔻 ■■■■■■___ + Out All the P type semiconductor anodes abut a metal conductive plane deposited on the top face of the slab with nonrectifying joins; the N type semiconductor cathodes or common cathode abuts the bottom face. As the polarity filtered electrical energy is exported, the amount of thermal energy in the group of diodes decreases. This group cooling will draw heat in from the surrounding ambient heat at a rate depending on the filtering rate and thermal resistance between the group and ambient gas, liquid, or solid warmer than absolute zero. There is always a lot of ambient heat on our planet, more on equatorial dry desert summer days and less on polar desert winter nights. Focusing on the composition of one simple diode, a near flawless crystal of silicon is modified by implanting a small amount of phosphorus (N type conductivity) on one side from a ohmic contact end to a junction where the additive is suddenly and completely changed to boron (P type conductivity) with minimal disturbance of the crystal lattice. The crystal then continues to another ohmic contact. A region of high electrical resistance forms at the junction in this type of diode when the phosphorous near the ĵunction donates electrons that are free to move elsewhere while leaving phosphorus ions held in the crystal while the boron donates holes which are similalarly free to move. The two types of mobile charges mutually clear each other away near the junction leaving little electrical conductivity. An equlibrium width of this region is settled between the phosphorus, boron, electrons, and holes. Thermal noise is beyond steady state equlibrium. Thermal noise transients, where mobile electrons move from the phosphorus added side to the boron added side ride transient extra conductivity so the forward moving electrons are preferentally filtered into the external circuit. Mobile electrons are units of electric current. They lose their thermal energy of motion and gain electromotive force, another name for voltage, as they transition between the junction and the array electrical tap. Inside the diode, heat is absorbed: outside the diode, to exactly the same extent, an attached electrical circuit is energized. The voltage of a diode array is likely to be small so many similar arrays need to be put in series to build higher voltage. Understanding diodes is one way to become convinced that Johnson Nyquest thermal electrical noise can be rectified and aggregated. Self assembling development teams may find many ways to accomplish this wide mission. Taxonomically there should be many ways ways to convert heat directly into electricity. A practical device may use an array of Au needles in a SiO2 matrix abutting N type GaAs. These were made in the 1970s when registration technology was poor so it was easier to fabricate arrays and select one diode than just make one diode. There are other plausible breeches of the second law of thermodynamics. Hopefully a lot of people, mostly as independent teams, will join in expanding the breech. Please share the successes or setbacks of experiemental efforts. These devices would probably become segmented commodities sold with minimal margin over supply cost. They would be manufactured by advanced automation that does not need financial incentive. Applicable best practices would be adopted. Business details would be open public knowledge. Associated people should move as negotiated and freely and honestly talk. Commerce would be a planetary scale unified conglomerate of diverse local cooperatives. There is no need of wealth extracting top commanders. We do not need often token philanthropy from the top if the wide majority of people can afford to be generous. Aloha Charles M Brown Kilauea Kauai Hawaii 96754
Amazing, cheers from Peru!
Jevon's paradox♥
One of the best sessions
I have gained indepth knowledge on the intersection of artificial intelligence and health
Great concept of ev anomaly detection , will be very applicable in real world
Very interesting and informative session.
Thank you, very informative
This is impressive
Dr. Mitra, intonation would help me understand better. Thank you.
👍
I've learned so much. I'd never thought about the impact that ML and AI have on emissions. Thank you
Very informative and eye-opening lecture. Thank you all!
Quick note at 41:10. To fix the `ValueError: Cannot convert '68' to a shape.` error, line 2 should be `model.add(Input(shape=(X_test.shape[1], )))` instead of `model.add(Input(shape=X_test.shape[1]))`
Thank you so much for this:)
Why aren't your video views increasing? And not reaching people? The reason is: 1. Your video is not being SEO properly 2. Your video is not reaching the right people 3. Not using video hash tags properly 4. Not sharing the video on social media There are a few more reasons. That's why your videos are limited to your channel. Can't get out In a word, your video is awesome. Such videos are in great demand. If you do these things correctly. Hopefully, you will get a lot of visitors. I can help with that. My info is on this very channel I'm using to comment.
🎯 Key points for quick navigation: 🌍 Cities play a significant role in global climate change, contributing to over 70% of global greenhouse gas emissions and facing unique challenges like the urban heat island effect. 📊 Data-driven approaches are crucial for cities to mitigate and adapt to climate change impacts, from identifying emissions hotspots to improving urban planning for transportation and waste management. 🏙️ Urban areas lack consistent and comparable data on emissions, hindering effective climate action planning and evaluation of mitigation efforts. 🌡️ The urban heat island effect exacerbates local temperatures, making cities more vulnerable to climate impacts such as heatwaves and extreme weather events. 🤖 AI technologies offer solutions for optimizing energy use in buildings, forecasting renewable energy production, and developing early warning systems for extreme weather events. 🔍 Current research highlights a need for AI to address data gaps in understanding urban emissions and evaluating the effectiveness of climate action plans. 🛠️ The DPSIR framework provides a structured approach for integrating AI-driven data analytics into urban climate policies, enhancing resilience and reducing vulnerability. 📈 Mitigation and adaptation strategies must work hand-in-hand as cities globally strive to combat climate change impacts while preparing for future environmental challenges. 🏙️ Cities globally face challenges in climate action, with significant gaps in emissions reporting and data consistency. 📊 Only a small fraction (less than 10%) of cities publicly report their greenhouse gas emissions inventories. 🌍 Initiatives like C40 Cities and the Global Covenant of Mayors play crucial roles in city climate action plans. 🛰️ Satellite and geospatial data are pivotal in augmenting city emission inventories where direct reporting is lacking. 🤖 Machine learning aids in data fusion from various sources to predict city emissions, filling data gaps effectively. 🔍 AI studies focus heavily on transportation and building sectors for climate mitigation in urban areas. 📈 Effective city actions identified include clean energy integration and optimized transportation management. 🌳 Climate adaptation in cities remains a research gap, with a focus needed on understanding local impacts and inequalities. 🌍 Urban heat disparities disproportionately affect low-income communities in dense city environments lacking green spaces and tree cover. 🛰️ Satellite remote sensing data measures land surface temperatures, revealing significant heat variations within cities. 🌡️ Fine-grained heat stress metrics using machine learning can provide more accurate assessments than traditional methods. 📊 Combining high-resolution data from sensors and aerial imagery helps create detailed heat stress maps for urban areas. 🏙️ Machine learning models like microclimate simulations aid in predicting localized heat radiation patterns across cities. 💡 Collaborations with local governments are crucial for implementing effective heat mitigation strategies based on AI data insights. 🌳 Initiatives like coating hot roadways with reflective materials show immediate responses to identified urban heat hotspots. 🌡️ Challenges include the need for comprehensive and transparent data to build reliable AI models for urban climate adaptation. 🏙️ Global Building Morphology Indicators project introduced metrics, open-source tools, and datasets to analyze urban form across cities worldwide. 🌐 Data quality in geospatial datasets, like OpenStreetMap, varies significantly, impacting urban research and AI applications. 🛠️ G mapper uses AI to predict building footprints, addressing data gaps and enhancing urban morphology studies. 🏢 Semantic attributes in geospatial data (e.g., building type, floors) from OpenStreetMap are often incomplete and inaccurately recorded. 📊 Research identifies less than 20% completeness in semantic attributes globally, affecting climate change studies reliant on accurate building data. 🏗️ AI leverages real estate listings to fill data gaps on building attributes like construction year and amenities, enhancing urban analytics. ☀️ Rooftop analysis using AI reveals current usage (e.g., solar panel installation) across cities, aiding urban sustainability planning. 🌡️ Human comfort in buildings is studied using AI and physiological data, identifying preferences and optimizing indoor environments. 🏙️ Emerging data sources are crucial for gaining deeper insights into built environments, which are increasingly complex. 🌍 As AI techniques advance, there's a growing need to scrutinize data quality across various scales and sources. 📊 Integrating diverse data sets remains challenging yet essential for future urban research and sustainability efforts. 🧑💼 Human-centric AI models are gaining importance, emphasizing the collection of personalized data to enhance urban living. 🌱 Urban sustainability efforts are enhanced by leveraging integrated platforms like digital twins for comprehensive data analysis. 🔍 Building-specific data supports vulnerability assessments, such as earthquake risk predictions, aiding in urban resilience planning. 🌏 AI applications are adaptable across diverse urban contexts, benefiting both highly developed cities and less developed regions. 🌡️ Considerations of AI's environmental impact highlight the need for energy-efficient model training and inference processes. Made with HARPA AI
👍👍
🎯 Key points for quick navigation: 02:24 *🎤 Dr. Angela Daka emphasizes integrating African philosophies and epistemology into AI debates.* 05:37 *🌍 AI's impact on climate change must consider power dynamics and ethical implications globally.* 10:25 *🤝 Understanding AI's role in climate action requires addressing equity and justice issues globally.* 18:37 *🌱 AI's deployment in agriculture offers potential benefits in efficiency but raises concerns about data privacy and environmental impact.* 26:47 *🐾 AI plays a crucial role in monitoring environmental changes but contributes to increased greenhouse gas emissions and environmental exploitation.* 32:24 *🌍 AI can significantly impact energy efficiency and water purification systems, potentially reducing greenhouse gas emissions by 40%.* 35:29 *🌐 Vulnerable populations are disproportionately affected by climate change, experiencing severe economic and environmental losses.* 36:38 *🌱 AI can aid in climate change predictions, enhance environmental conservation efforts, and optimize resource management and energy consumption.* 40:07 *🌏 Global discussions on AI often overlook ethical considerations, focusing more on technical solutions and economic benefits, neglecting climate justice and human rights.* 46:32 *📉 AI deployment and funding dynamics perpetuate global inequalities, favoring technological solutions over addressing underlying climate and social issues.* 01:05:27 *🌍 Regulation of AI in relation to climate change is gaining mainstream political attention, highlighting the need for regulatory frameworks to address sustainability impacts.* 01:10:17 *🤖 The definition of AI in the AI Act is intentionally broad, encompassing machine-based systems with varying autonomy levels that infer outputs from inputs, potentially including basic functions like Excel's auto-sum.* 01:18:16 *🚨 The AI Act categorizes AI systems into four risk levels: prohibited, high-risk, limited risk, and minimal risk, each with specific regulatory requirements to manage societal and environmental impacts.* 01:20:48 *📜 The AI Act mandates AI literacy for all personnel handling AI systems across industries, emphasizing the importance of education and transparency in AI usage.* 01:23:18 *💬 AI systems like chatbots must disclose their AI nature when interacting with humans, ensuring transparency and avoiding the illusion of human interaction.* 01:29:49 *🌍 Sustainability concerns are inadequately addressed in current AI regulations, such as the Digital Markets Act, highlighting a significant regulatory gap.* 01:30:17 *🔍 Providers of high-risk AI systems must ensure transparency, bias minimization in training data, and adhere to rigorous documentation and performance metrics.* 01:32:06 *📊 Foundation models like GP4 are subject to transparency rules under Article 503, impacting their regulatory requirements.* 01:34:39 *🌱 ICT and AI contribute significantly to global greenhouse gas emissions, comparable to sectors like global air travel, necessitating sustainable AI policy responses.* 01:35:46 *⚡️ The energy consumption of AI, both during training and inference, poses substantial environmental challenges, surpassing many traditional sectors.* 01:37:16 *🔄 Generative AI models, despite their popularity, offer limited potential for climate change mitigation due to their inefficiencies in energy and resource use.* 01:39:04 *🌍 AI's regulation for energy use is crucial due to its escalating emissions, positioning it for early regulatory focus compared to other emerging technologies.* 01:40:26 *💧 Current AI regulations overlook environmental impacts like water consumption and cooling requirements, highlighting gaps in regulatory frameworks.* 01:43:14 *🚫 AI is not included in traditional emissions trading systems, underscoring a significant regulatory gap in addressing AI's environmental footprint.* 01:50:17 *🛡️ AI regulations indirectly incorporate environmental protection via risk assessment frameworks, despite environmental protection not being a fundamental right in EU law.* 01:54:00 *🌍 AI applications should consider varying energy budgets based on social utility, distinguishing between medical uses and entertainment purposes.* 01:54:28 *💡 Financial incentives are crucial to regulate AI's environmental impact, as current market dynamics prioritize leaderboard rankings over sustainability.* 01:55:10 *🏢 Data centers are not sufficiently covered by current supply chain regulations, posing challenges for managing their environmental impact.* 01:56:02 *📊 Standardizing climate impact reporting and AI training rules are essential for effective regulation and industry accountability.* 01:57:38 *⚡️ Large tech companies should be obligated to contribute actively to renewable energy creation, rather than solely purchasing existing renewable energy sources.* 01:58:19 *🌱 Defining carbon and energy budgets tailored to different AI applications based on their societal benefits is a complex but necessary task.* Made with HARPA AI
Dr. Lily Very neatly captured how different AI techniques could be effectively used in biodiversity conservation. Loved her lecture
🎯 Key points for quick navigation: 00:04 *🌍 Overview of Climate Change AI Virtual Summer School 2024* - Overview of sponsors and supporters of the virtual summer school, - Introduction to the session's structure and participation guidelines. 02:03 *🎓 Introduction to Dr. Kasia Tokarska* - Dr. Kasia Tokarska's background and expertise in climate modeling and machine learning, - Her current role at Carbon Pool and focus on climate risk analysis. 03:15 *📊 Motivation for Emissions Accounting* - Understanding the physical and transition risks associated with greenhouse gas emissions, - Importance of emission accounting for policy compliance and risk management. 05:08 *📉 Types and Challenges of Emission Accounting* - Differentiating between scope 1, 2, and 3 emissions, - Challenges in controlling and reducing scope 3 emissions for companies. 18:45 *🤖 Machine Learning Approaches for Emission Monitoring* - Overview of bottom-up, data-driven, and satellite data approaches to emission quantification, - Potential and limitations of machine learning in climate change mitigation strategies. 23:34 *📊 Using clustering and mapping methods for emissions data analysis* - Using clustering methods requires careful consideration of data relevance and accuracy. - Attributes like company size, revenue, and sourcing channels impact accuracy. - Data biases can skew predictions, especially with limited data diversity. 25:13 *📈 Exploring feature importance in predicting Scope 3 emissions* - Understanding which features are crucial for accurate emission predictions. - Different features may be necessary for each category of emissions. - Balancing complexity with data availability and model accuracy is crucial. 30:16 *🛰️ Integrating satellite data for top-down emission estimates* - Satellite data can complement bottom-up methods for emission monitoring. - Machine learning improves real-time emission estimates from satellite data. - Enhancing accuracy through hybrid approaches of top-down and bottom-up methods. 32:39 *🌳 Monitoring biomass changes and carbon sequestration* - Machine learning aids in monitoring nature-based carbon removals like afforestation. - Remote sensing and AI predict biomass changes and carbon stocks globally. - Ensuring temporal and spatial consistency remains a challenge in biomass predictions. 35:07 *🌍 Global biomass data set creation using machine learning* - Creating a detailed global biomass data set for carbon sequestration. - Utilizing machine learning to estimate biomass with one-meter resolution globally. - Challenges include data consistency and regional performance biases. 45:01 *🛰️ Challenges of Using Satellite Data* - Challenges with remote sensing data include interpretation limitations and errors due to factors like cloud cover affecting data accuracy. - Remote sensing provides diverse metrics such as NDVI and soil moisture, each with its interpretation challenges. 47:09 *🌿 Biomass Monitoring vs. Deforestation* - Monitoring biomass involves complex calculations due to species variation and canopy factors. - Deforestation monitoring is comparatively simpler but lacks detailed biomass and carbon sequestration insights. 48:32 *📊 Criteria for Model Accuracy* - Validation criteria for models depend on the specific project's objectives and ground truth data availability. - Statistical tests and domain expert collaboration are essential for accurate model validation. 49:43 *🌍 Global Climate Data Challenges* - Utilizing climate data requires careful selection to avoid biases and ensure meaningful outputs. - Understanding climate system phenomena aids in interpreting and utilizing climate model outputs. 52:01 *🌐 Using Satellite Data for Ecosystem Change Analysis* - Satellite data from 2014 onwards offers extensive coverage for tracking ecosystem changes globally. - Selecting appropriate data sources and understanding their limitations are crucial for accurate analysis. 54:21 *🌊 CO2 Emissions and Marine Ecosystems* - Assessing CO2 emissions from marine ecosystems requires integrating process-based ocean circulation models. - Satellite data alone may not suffice due to water reflectance issues and the depth complexity of marine environments. 57:00 *🌱 Using Allometric Equations for CO2 Emission Prediction* - Allometric equations aid in calculating CO2 emissions by quantifying carbon storage in different plant parts. - Regional and species-specific variations influence the accuracy of CO2 emission predictions. 59:09 *🔄 Machine Learning for Carbon Offsets* - Machine learning's focus on carbon removal aids in achieving net-zero emissions goals effectively. - Offset solutions beyond removal, like emissions reduction, offer interim solutions but do not address atmospheric CO2 levels directly. Made with HARPA AI
🎯 Key points for quick navigation: 00:04 *🌍 Overview of Climate Change AI Virtual Summer School 2024* - Overview of sponsors and supporters of the virtual summer school, - Introduction to the session's structure and participation guidelines. 02:03 *🎓 Introduction to Dr. Kasia Tokarska* - Dr. Kasia Tokarska's background and expertise in climate modeling and machine learning, - Her current role at Carbon Pool and focus on climate risk analysis. 03:15 *📊 Motivation for Emissions Accounting* - Understanding the physical and transition risks associated with greenhouse gas emissions, - Importance of emission accounting for policy compliance and risk management. 05:08 *📉 Types and Challenges of Emission Accounting* - Differentiating between scope 1, 2, and 3 emissions, - Challenges in controlling and reducing scope 3 emissions for companies. 18:45 *🤖 Machine Learning Approaches for Emission Monitoring* - Overview of bottom-up, data-driven, and satellite data approaches to emission quantification, - Potential and limitations of machine learning in climate change mitigation strategies. 23:34 *📊 Using clustering and mapping methods for emissions data analysis* - Using clustering methods requires careful consideration of data relevance and accuracy. - Attributes like company size, revenue, and sourcing channels impact accuracy. - Data biases can skew predictions, especially with limited data diversity. 25:13 *📈 Exploring feature importance in predicting Scope 3 emissions* - Understanding which features are crucial for accurate emission predictions. - Different features may be necessary for each category of emissions. - Balancing complexity with data availability and model accuracy is crucial. 30:16 *🛰️ Integrating satellite data for top-down emission estimates* - Satellite data can complement bottom-up methods for emission monitoring. - Machine learning improves real-time emission estimates from satellite data. - Enhancing accuracy through hybrid approaches of top-down and bottom-up methods. 32:39 *🌳 Monitoring biomass changes and carbon sequestration* - Machine learning aids in monitoring nature-based carbon removals like afforestation. - Remote sensing and AI predict biomass changes and carbon stocks globally. - Ensuring temporal and spatial consistency remains a challenge in biomass predictions. 35:07 *🌍 Global biomass data set creation using machine learning* - Creating a detailed global biomass data set for carbon sequestration. - Utilizing machine learning to estimate biomass with one-meter resolution globally. - Challenges include data consistency and regional performance biases. 45:01 *🛰️ Challenges of Using Satellite Data* - Challenges with remote sensing data include interpretation limitations and errors due to factors like cloud cover affecting data accuracy. - Remote sensing provides diverse metrics such as NDVI and soil moisture, each with its interpretation challenges. 47:09 *🌿 Biomass Monitoring vs. Deforestation* - Monitoring biomass involves complex calculations due to species variation and canopy factors. - Deforestation monitoring is comparatively simpler but lacks detailed biomass and carbon sequestration insights. 48:32 *📊 Criteria for Model Accuracy* - Validation criteria for models depend on the specific project's objectives and ground truth data availability. - Statistical tests and domain expert collaboration are essential for accurate model validation. 49:43 *🌍 Global Climate Data Challenges* - Utilizing climate data requires careful selection to avoid biases and ensure meaningful outputs. - Understanding climate system phenomena aids in interpreting and utilizing climate model outputs. 52:01 *🌐 Using Satellite Data for Ecosystem Change Analysis* - Satellite data from 2014 onwards offers extensive coverage for tracking ecosystem changes globally. - Selecting appropriate data sources and understanding their limitations are crucial for accurate analysis. 54:21 *🌊 CO2 Emissions and Marine Ecosystems* - Assessing CO2 emissions from marine ecosystems requires integrating process-based ocean circulation models. - Satellite data alone may not suffice due to water reflectance issues and the depth complexity of marine environments. 57:00 *🌱 Using Allometric Equations for CO2 Emission Prediction* - Allometric equations aid in calculating CO2 emissions by quantifying carbon storage in different plant parts. - Regional and species-specific variations influence the accuracy of CO2 emission predictions. 59:09 *🔄 Machine Learning for Carbon Offsets* - Machine learning's focus on carbon removal aids in achieving net-zero emissions goals effectively. - Offset solutions beyond removal, like emissions reduction, offer interim solutions but do not address atmospheric CO2 levels directly. Made with HARPA AI
I'm a graduate of Ecotourism and Wildlife Management and I am passionate about ICT in biodiversity conservation
🎯 Key points for quick navigation: 00:18 *Climate Change AI Summer School introduces lectures on AI applications for climate science and weather forecasting.* 03:02 *Kai Jeggle discusses using AI and satellite data to enhance understanding of cloud processes in climate science.* 11:14 *AI's use in climate science research has seen exponential growth in publications, indicating its increasing importance.* 15:22 *AI benefits climate science by handling large datasets, modeling nonlinear dependencies, and reducing computational costs.* 22:29 *AI aids weather and climate models through AI-driven predictions and emulating subit processes, enhancing forecasting accuracy and efficiency.* 27:43 *AI models like "icet" use machine learning to predict polar ice sheet behavior, demonstrating effective climate impact forecasting.* 28:39 *AI used by the British Antarctic Survey effectively improves climate science by enhancing cloud data insights.* 29:06 *"Ice Cloud Net" project integrates AI for 3D cloud reconstruction using sensor fusion, significantly increasing data availability over traditional methods.* 30:25 *AI techniques like UNet and Patch GAN are employed to reconstruct vertical cloud structures, enhancing understanding of cloud formation processes.* 32:38 *Challenges in AI for climate science include data bias, energy consumption, and ensuring models are physically consistent and interpretable.* 55:28 *AI predictions in climate science emphasize the need for robust evaluation criteria beyond simple accuracy metrics, focusing on spatial patterns and model robustness.* 55:57 *Current AI models for climate modeling show promise in predicting extreme events efficiently through ensemble runs, providing directional insights with low variability.* 01:18:47 *AI weather models use probabilistic approaches with initial conditions to account for uncertainty, crucial for accurate forecasts.* 01:19:41 *Spaghetti diagrams in weather forecasting display forecast uncertainty, offering probabilities for events like extreme rain or heat waves.* 01:21:08 *AI-driven weather models differ from traditional methods by learning rules from data rather than predefined equations, enhancing forecast accuracy.* 01:22:57 *AI models in weather forecasting must distinguish between interpolation (within known data) and extrapolation (beyond known data), crucial for adapting to changing climates.* 01:23:51 *AI's ability to process vast amounts of atmospheric data can improve weather forecasts significantly, especially in data-rich regions.* 01:40:29 *AI weather models, like Transformers and graph networks, encode high-dimensional weather data into reduced latent spaces for efficient forecasting.* 01:41:39 *Embeddings in AI weather models help efficiently learn influences across different spatial scales, focusing forecasting efforts where weather interactions are significant.* 01:44:41 *Weather embeddings in AI models enhance forecasting accuracy by focusing on prognostic variables, reducing training and deployment times significantly.* 01:46:02 *AI models excel in predicting rare weather events with better accuracy than traditional physics-based models, leveraging their ability to learn complex, non-linear relationships.* 01:50:14 *AI weather models complement traditional NWP models but are not yet advanced enough to replace them due to ongoing advancements and the need for rigorous validation and integration with existing systems.* Made with HARPA AI
8:33 Don't want to be negative, but the plot shows the conceptual model as the one with high computing time, while the speaker says the opposite. Thank you for the lecture and for posting it!
- 🌍 Climate Innovation Policy integrates AI with social sciences to drive green transition. - 📈 Transition to a low-carbon economy requires systemic shifts in technology and policy. - 🚀 Historical parallels with the Industrial Revolution highlight rapid innovation's impact. - 🛠 Challenges include developing and scaling carbon-neutral technologies by 2030. - 💡 Innovation in sectors like transport varies; policies crucial for widespread adoption. - 💰 Public and private investment gaps need addressing, especially in developing nations. - 🌱 State intervention crucial: sets standards, de-risks investments, fosters innovation. - 🌐 International collaboration essential for setting global climate tech standards. - 🌱 Governments play a crucial role in climate innovation policy by setting standards, creating market demand through policies like renewable energy mandates, and training labor forces. - ⚖ Climate innovation policies use a mix of sticks (disincentives like penalties and market restrictions) and carrots (incentives like subsidies and tax benefits) to drive green technology adoption. - 📊 Carbon pricing, such as carbon taxes and cap-and-trade schemes, internalizes the social cost of carbon emissions, incentivizing both producers and consumers towards greener choices. - 🌍 Public sector investment alone is insufficient to meet climate goals; attracting private sector investment through financial incentives and de-risking policies is crucial. - 💡 Innovation in green tech requires not just financial incentives but also supportive regulatory frameworks and policies that ensure scalability and accessibility. - 🚗 Electrification of transport systems, supported by policies like tax rebates for electric vehicles, is pivotal in reducing carbon emissions and transitioning to a low-carbon economy. - 🔋 Green bonds and other financial products are essential tools for financing green projects, leveraging private sector capital towards sustainable development. - 🌐 Policy packages need to address equity concerns and social impacts, ensuring that the transition to green technologies benefits all sectors of society. - 🌍 Climate policy involves significant equity and fairness challenges, both within and across countries. - 🌱 Developing countries face dilemmas in climate negotiations due to historical emissions disparities and limited adaptive capacity. - 🏭 There's potential for developing countries to bypass dirty industrialization and leapfrog to green technologies with international support. - 🤝 International aid and technology transfers are crucial for equitable climate action. - 📊 Differentiated responsibilities for climate action exist between Annex 1 and Annex 2 countries under the UNFCCC. - 🚀 Optimism exists for developing countries to benefit from investing in green futures and sustainable development. - 🧠 AI is recognized under the UNFCCC for enhancing climate technology solutions, focusing on mitigation and adaptation in developing countries. - 🏆 The AI Innovation Grand Challenge aims to foster AI-powered climate solutions in least developed countries and small island developing states. Notes Created by HARPA AI
Very nice presentation by Prof. Devis Tuia. He seems to have a welcoming personality too.
Thank you for sharing.
Thank you for sharing
- 🌿 Biodiversity crisis: Over 30% of species are projected to face extinction in the next 30 years, emphasizing the urgent need for conservation efforts. - 🌍 Global impact: Habitat loss affects every region, threatening ecosystem services like food production and water purification that humans depend on. - 📉 Data gaps: Approximately 10% of known species lack sufficient data, hindering effective conservation strategies. - 🦁 Wildlife monitoring: Tools like biologgers and camera traps aid in tracking individual animals, despite challenges like battery life and ethical considerations. - 🛰 Sensor diversity: Technologies include satellite imagery, drones, and acoustic sensors, each offering unique insights into biodiversity. - 📊 Conservation frameworks: International agreements aim to conserve 30% of land and sea globally, supported by significant funding for biodiversity studies. - 🎓 Role of AI: Machine learning enhances data analysis from sensors, supporting more efficient conservation decision-making. - 🌱 Future directions: Advances in digital tools and AI offer hope for reversing biodiversity decline through improved monitoring and management strategies. - 🌍 Drones provide safe, non-invasive methods for mapping and monitoring wildlife compared to traditional aerial methods like helicopters. - 🚁 Choosing the right drone involves considerations such as flight capabilities (stationary vs. fast), noise levels, and regulatory compliance. - 🛰 Satellite data offers broad coverage for ecological monitoring but varies in resolution and cost, influencing its practicality for different research needs. - 📸 Image processing and AI are essential for analyzing drone and satellite data to detect and count wildlife populations like elephants and penguins. - 🐧 AI models, like the Mega detector, aid in object detection from camera trap images, helping in wildlife conservation efforts and combating poaching. - 🦓 Machine learning models can significantly speed up animal census tasks traditionally done by humans, with potential for error reduction and efficiency. - 🐋 Computer vision techniques, such as identifying individual animals by unique features like whale flukes, enhance tracking and conservation efforts. - 📹 Pose estimation technologies, like DeepLabCut, enable tracking animal movements by recognizing body parts in video sequences, aiding behavioral studies. - 🌊 AI technologies like 3D modeling from cameras and lasers aid in environmental monitoring and biodiversity conservation. - 🐾 Monitoring tools help track wildlife behavior and habitat changes for effective conservation strategies. - 🌿 Digital twins of ecosystems created through AI allow for detailed biodiversity assessments. - 📡 Remote sensing data, like satellite imagery, combined with AI, enhances resolution for accurate environmental monitoring. - 🌏 Challenges include biases in data collection and the ethical use of open data for conservation efforts. - 🌱 AI models must consider energy efficiency and environmental impact to align with conservation goals. - 📉 Interdisciplinary collaboration is crucial for leveraging AI in climate change and biodiversity conservation. - 🛠 Accessible platforms like Sentinel Hub and Planet provide varying resolutions of satellite data for biodiversity research. - 🌿 Conservation challenges include pollution, human-wildlife conflict, and habitat loss, necessitating urgent action. - 🛡 Strategies like protected areas, alternative livelihoods, and community education are pivotal in biodiversity conservation. - 🐾 Translocation and reintroduction of species are crucial for restoring ecosystems and preserving biodiversity. - 💰 Conservation financing plays a vital role in supporting protected areas and local communities. - 📊 AI aids conservation through monitoring, planning, and impact evaluation using techniques like machine learning and optimization. - 🌍 Optimization tools like predictive modeling help maximize conservation efforts within budget constraints. - 💡 Market design and payments for ecosystem services incentivize conservation actions by compensating landowners. - 🔄 Online learning and reinforcement learning enable adaptive conservation strategies in dynamic environments like fisheries management. - 🌊 Marine ecosystem regulation involves setting sustainable harvest levels based on current fish populations. - 🎣 Regulators use reinforcement learning to make decisions that impact long-term fish population health. - 🌍 Markov Decision Processes (MDPs) form the basis for modeling ecological systems in AI. - 📊 Causal inference helps evaluate the effectiveness of conservation policies like Payments for Ecosystem Services (PES). - 🌳 Quantifying uncertainty in conservation decisions aids in robust optimization of ecological outcomes. - 🐅 Reward design in AI for conservation often focuses on proxies like keystone species populations. - 🧠 Ethical considerations emphasize human expertise and local community involvement in AI-driven conservation decisions. - 📚 Further resources are available for deeper exploration of AI applications in biodiversity conservation. Key Takeaways using HARPA
- 🌾 Agriculture and forestry are pivotal in understanding climate change impacts and responses. - 🛰 Satellite data is extensively used to monitor and predict agricultural and forestry conditions. - 🌍 Climate change poses significant threats to agricultural productivity and food security. - 🚜 Farmers seek AI-driven insights on crop yields, weather patterns, and soil health. - 🏛 Policymakers rely on AI to plan resilient agricultural policies at local and global scales. - 🌱 AI enhances sustainability in agriculture by optimizing crop management and resource use. - 📊 Remote sensing enables precise mapping of crop lands, types, and environmental conditions. - 🌿 AI supports early warning systems for agricultural crises caused by climate-related events. - 🌾 Crop mapping and crop type mapping in agriculture are key tasks involving binary and multiclass classification respectively, aiding in efficient land use. - 📈 Yield estimation in agriculture utilizes regression models to predict crop productivity per unit area, crucial for optimizing food production. - 🦠 Pest and disease detection in crops employs outlier detection methods to identify anomalies early, supporting timely interventions. - 🌍 Increasing crop yields can significantly conserve land, highlighting the importance of yield optimization in sustainable agriculture. - 🤖 Precision agriculture integrates AI for on-farm resource management, enhancing efficiency in water and nutrient application. - 🌲 AI for forestry focuses on applications like forest monitoring and management using remote sensing data and deep learning techniques. - 🌱 Self-supervised models like Presto are pivotal in remote sensing, pre-training on vast datasets to improve accuracy in agricultural mapping tasks. - 🚜 Robotics in agriculture enables tasks like automated weeding and pest control, enhancing productivity while minimizing environmental impact. - 🌲 Forests provide crucial ecosystem services like carbon storage, water purification, and biodiversity support. - 🌍 Deforestation contributes significantly to global warming, releasing stored carbon and disrupting climate regulation. - 🛡 Conservation efforts include Forest Carbon Credits, incentivizing reforestation and preventing deforestation. - 🌳 Agroforestry integrates trees with agriculture, enhancing sustainability and biodiversity. - 📜 Nature regulations like the EU Deforestation Law aim to curb deforestation in supply chains. - 🌱 Restoration initiatives, such as the EU Nature Restoration Act, set targets to restore degraded ecosystems. - 📊 Monitoring and verification challenges hinder effective implementation of nature protection policies. - 🌐 Global efforts like the One Trillion Tree Initiative aim to mitigate climate change through massive reforestation efforts. - 🌲 AI and carbon credits: AI helps monitor forests for carbon credit programs, ensuring environmental and climate safeguards. - 🌍 Multimodal data fusion: AI leverages diverse environmental data (satellite, drone, DNA) to monitor biodiversity and ecosystem health. - 🛰 Remote sensing integration: Combining satellite and drone data improves accuracy in forest monitoring and deforestation detection. - 🌳 Field-based AI applications: AI apps and drones measure tree biomass and species diversity, reducing manual effort and improving accuracy. - 🎶 Bioacoustic monitoring: AI analyzes forest sounds to identify biodiversity and ecosystem health, aiding in conservation efforts. - 🌐 Global data biases: Existing AI biases due to data gaps in biodiversity data from regions like the Amazon and Congo pose challenges. - 🌿 Indigenous involvement: Co-designing AI tools with indigenous communities ensures fairness and accuracy in forest management. - 💡 Future challenges: Overcoming biases and integrating local knowledge are critical for effective AI deployment in nature conservation. - 🌱 Generative AI facilitates knowledge sharing in local communities through storytelling, overcoming language barriers and fostering trust in data ownership. - 🌎 AI models are enhancing inclusivity by enabling communication in local languages like Portuguese, empowering indigenous communities to manage and share their oral histories. - 🌳 Incorporating indigenous knowledge with AI enhances environmental monitoring, such as using AI to predict locations of economically valuable trees like Brazil nuts. - 📡 AI technologies like geospatial models and sensors are co-designed with communities to improve accuracy in environmental sampling and monitoring. - 💬 Transparent AI algorithms are crucial for fair policy decisions and avoiding biased data impacts, requiring close collaboration with local communities. - 💡 Benefits of AI applications must be shared with communities to ensure ethical data use and maintain trust, promoting equitable outcomes from technology advancements. - 🤝 Co-designing AI technologies with local communities fosters mutual respect and ensures that data ownership and benefits are prioritized for community use. - 🌍 Environmental conservation efforts benefit from AI-driven insights into deforestation risks and carbon credit assessments, though challenges remain in integrating local and scientific knowledge effectively. Notes I created Using HARPA
The lecture is very educative and cause upskilling on machine learning on urban sustainability.
I really enjoyed this lecture since I am a biology student and work in marine biology research. I consider that these tools will be very useful for me specially 3D models
Impressive session
I guess, website of Fews Net is not updated. Ukraine is included, Gaza is not in the Countries & Regions in Food Insecurity.
nice
starts at 2:53
A lot of thanks for sharing
- 🌍 Climate Change AI (CCAI) Virtual Summer School 2024 aims to bridge climate change and machine learning. - 📈 Over 140 countries participating, emphasizing global interest and engagement. - 🎓 12-day program includes 11 lectures, tutorials, and peer-to-peer learning opportunities. - 📅 Schedule includes sessions on climate change, machine learning, and panel discussions. - 🌱 Urgent need to achieve Net Zero emissions by 2050 to mitigate climate impacts. - 🤖 Machine learning's role: distilling data, optimizing systems, improving predictions, and accelerating scientific discovery. - 🛠 Key themes include climate science, mitigation, adaptation, and interdisciplinary collaboration. - 🌐 Emphasis on holistic strategies integrating climate justice and sustainable development goals. - 🌍 Machine learning enhances climate models' resolution, aiding local climate adaptation by downscaling. - 🚗 ML optimizes car aerodynamic simulations, aiming for more fuel-efficient vehicle designs. - ⚡ ML accelerates planning models for electrical grids, enhancing simulation speed. - 📊 Prioritizing ML applications involves assessing impact scope and feasibility for climate solutions. - 🌱 Early reduction of CO2 has higher impact, emphasizing the urgency in climate actions. - 🔍 ML solutions must consider stakeholder involvement and mitigate potential negative side effects. - 🤝 Collaboration between ML and domain experts ensures effective problem-solving and deployment. - 🌐 ML applications for climate action require robust, interpretable models and equitable deployment strategies. - 🌍 There are diverse job opportunities in climate-focused sectors including academia, startups, tech companies, and public bodies, each contributing to climate action. - 🤝 Collaboration between machine learning experts and domain specialists is crucial for addressing climate-related challenges effectively. - 📊 Machine learning professionals can integrate climate considerations into existing projects or focus directly on climate-related applications. - 📈 Opportunities exist to participate in challenges, workshops, and conferences focused on machine learning and climate change. - 📚 Researchers can publish their work in venues like AI conferences and journals, contributing to the intersection of AI and climate science. - 🏛 Policy engagement is vital, with governments increasingly integrating AI for climate policy and seeking expert input. - 🔄 Machine learning models inherit biases from data, highlighting the importance of ethical considerations and diverse input. - 🌱 The introduction to machine learning covered supervised, unsupervised, and reinforcement learning, each applicable to different types of data and tasks. - 🌍 Supervised learning involves training models with labeled data to predict outcomes like yes/no or numerical values. - 📊 Classification predicts categorical labels (e.g., pregnant or not), while regression predicts continuous values (e.g., stock prices). - 🚴♂ Example: Predicting bicycle availability using historical data demonstrates regression with continuous labels. - 🏞 K Nearest Neighbors (KNN) is a simple supervised learning model suitable for small to medium-sized datasets. - 📏 Overfitting occurs when a model performs well on training data but poorly on unseen test data, indicating excessive complexity. - 🎯 Evaluation metrics like precision, recall, and F1 score assess model performance in supervised learning. - 🌀 Unsupervised learning finds patterns in unlabeled data, useful for tasks like clustering and topic modeling. - 🌟 K-means clustering is a common unsupervised learning algorithm for grouping data points into clusters based on similarity. - 📄 Topic modeling is effective for analyzing large volumes of unstructured text data by extracting main topics and categorizing documents. - 🎮 Reinforcement learning involves agents maximizing rewards through actions in an environment, applicable from games like Pac-Man to optimizing complex systems like power grids. - 🌐 Generative models like language models predict sequences (e.g., text) based on learned patterns from large datasets, though they can perpetuate biases present in their training data. - 📊 Unsupervised learning, such as clustering algorithms, can assess data patterns without predefined labels, verified through metrics like silhouette coefficient. - 🤖 Machine learning's key challenges include overfitting, addressed by techniques like cross-validation, and balancing exploration (finding new actions) vs. exploitation (repeating known good actions). - 🖥 Applications in climate policy analysis can benefit from machine learning to process and understand extensive policy documents efficiently. - 🏞 Understanding reinforcement learning involves actions, states, and rewards, critical for training algorithms to make optimal decisions autonomously. - 📉 Supervised learning contrasts with reinforcement learning by training on labeled data for specific tasks rather than learning from feedback via rewards. - 🌍 Introduction to AI: Covers the history, challenges, and future directions of AI, essential for foundational understanding. - 🛠 Infrastructure for AI: Discusses practical tools like IDEs, Git, and Google Colab for managing projects and accessing resources like GPUs. - 🧠 Neural Networks: Core components of deep learning, including layers, activations, and design decisions crucial for AI applications. - 📊 Paradigms of Machine Learning: Explores different problem setups and modeling strategies based on data availability and type. - 🖼 Computer Vision: Application of AI to visual data like images and videos, with tools such as CNNs and image processing pipelines. - 📜 Natural Language Processing (NLP): Techniques for processing human language, beneficial for tasks like information retrieval and summarization. - 📐 Math Fundamentals: Essential for understanding AI concepts such as linear algebra, calculus, and probability, even in AI toolkits. - 🔍 Interpretability and Explainability: Methods to understand AI model decisions, crucial for transparency and trust in high-stakes applications. - 🌱 Estimating carbon energy consumption for machine learning models is complex due to factors like energy source and lifetime costs of hardware components. - ⚡ It's challenging to accurately assess the energy footprint of training models, considering both GPU usage and data center operations. - 🌍 Current frameworks lack robust tools for estimating the environmental impact of machine learning models. - 🔍 Researchers like Sasha Luini have explored papers that touch on energy costs related to training large models. - 📊 Lifetime costs of hardware and energy for inference also contribute significantly to a model's overall environmental impact. - 🌿 Inference processes are becoming more energy-intensive, potentially rivaling or exceeding training costs in energy consumption. - 🔄 Integrating renewable energy sources into model training could mitigate environmental impact, but frameworks for this are underdeveloped. - 🎓 Resources for using reinforcement learning in agriculture to optimize tasks like water temperature adjustments are sought after in practical applications. Key takeaways - Using HARPA AI
Thank you very much
"That terrifies me please don't do that"
very good!
Thank you very much, it was a pleasure to follow him
A lot of thanks