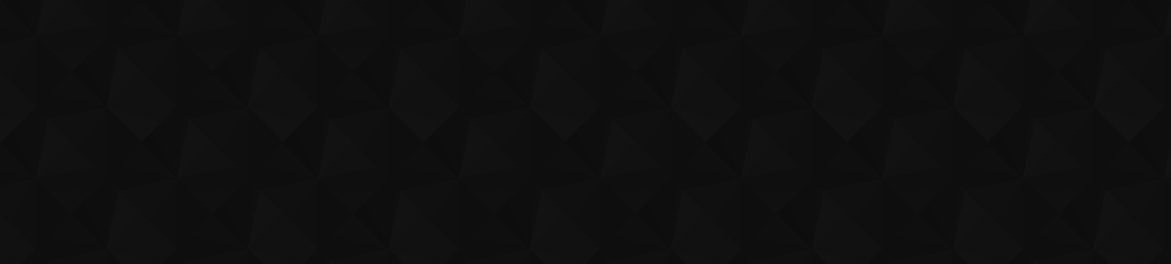
- 52
- 126 918
ICML IJCAI ECAI 2018 Conference Videos
United States
เข้าร่วมเมื่อ 12 ก.ค. 2018
AAAI 20 / AAAI 2020 Keynotes Turing Award Winners Event / Geoff Hinton, Yann Le Cunn, Yoshua Bengio
**Highlighted Topics**
02:52 [Talk: Stacked Capsule Autoencoders by Geoffrey Hinton]
36:04 [Talk: Self-Supervised Learning by Yann LeCun]
1:09:37 [Talk: Deep Learning for System 2 Processing by Yoshua Bengio]
1:41:06 [Panel Discussion]
Auto-chaptering powered by VideoKen (videoken.com/)
For indexed video, conftube.com/video/vimeo-390347111
**All Topics**
03:09 Two approaches to object recognition
03:53 Problems with CNNs: Dealing with viewpoint changes
04:42 Equivariance vs Invariance
05:25 Problems with CNNs
10:04 Computer vision as inverse computer graphics
11:55 Capsules 2019: Stacked Capsule Auto-Encoders
13:21 What is a capsule?
14:58 Capturing intrinsic geometry
15:37 The generative model of a capsule auto-encoder
20:28 The inference problem: Inferring wholes from parts
21:44 A multi-level capsule auto-encoder
22:30 How the set transformer is trained
23:14 Standard convolutional neural network for refining word representations based on their context
23:41 How transformers work
24:43 Some difficult examples of MNIST digits
25:20 Modelling the parts of MNIST digits
27:03 How some of the individual part capsules contribute to the reconstructions
28:37 Unsupervised clustering of MNIST digits using stacked capsule autoencoders
31:25 The outer loop of vision
31:36 Dealing with real 3-D images
32:51 Conclusion
36:04 *[Talk: Self-Supervised Learning by Yann LeCun]*
36:25 What is Deep Learning?
38:37 Supervised Learning works but requires many labeled samples
39:25 Supervised DL works amazingly well, when you have data
40:05 Supervised Symbol Manipulation
41:50 Deep Learning Saves Lives
43:40 Reinforcement Learning: works great for games and simulations.
45:12 Three challenges for Deep Learning
47:39 How do humans and animals learn so quickly?
47:43 Babies learn how the world works by observation
48:43 Early Conceptual Acquisition in Infants [from Emmanuel Dupoux]
49:33 Prediction is the essence of Intelligence
50:28 Self-Supervised Learning = Filling in the Blanks
50:53 Natural Language Processing: works great!
51:55 Self-Supervised Learning for Video Prediction
52:09 The world is stochastic
52:43 Solution: latent variable energy-based models
53:55 Self-supervised Adversarial Learning for Video Prediction
54:12 Three Types of Learning
55:30 How Much Information is the Machine Given during Learning?
55:54 The Next Al Revolution
56:23 Energy-Based Models
56:32 Seven Strategies to Shape the Energy Function
57:02 Denoising AE: discrete
58:44 Contrastive Embedding
1:00:39 MoCo on ImageNet
1:00:52 Latent-Variable EBM for inference & multimodal prediction
1:02:07 Learning a (stochastic) Forward Model for Autonomous Driving
1:02:26 A Forward Model of the World
1:04:42 Overhead camera on highway. Vehicles are tracked
1:05:00 Video Prediction: inference
1:05:15 Video Prediction: training
1:05:30 Actual, Deterministic, VAE+Dropout Predictor/encoder
1:05:57 Adding an Uncertainty Cost (doesn't work without it)
1:06:01 Driving an Invisible Car in "Real" Traffic
1:06:51 Conclusions
1:09:37 *[Talk: Deep Learning for System 2 Processing by Yoshua Bengio]*
1:10:10 No-Free-Lunch Theorem, Inductive Biases Human-Level AI
1:15:03 Missing to Extend Deep Learning to Reach Human-Level AI
1:16:48 Hypotheses for Conscious Processing by Agents, Systematic Generalization
1:22:02 Dealing with Changes in Distribution
1:25:13 Contrast with the Symbolic AI Program
1:28:07 System 2 Basics: Attention and Conscious Processing
1:28:19 Core Ingredient for Conscious Processing: Attention
1:29:16 From Attention to Indirection
1:30:35 From Attention to Consciousness
1:31:59 Why a Consciousness Bottleneck?
1:33:07 Meta-Learning: End-to-End OOD Generalization, Sparse Change Prior
1:33:21 What Causes Changes in Distribution?
1:34:56 Meta-Learning Knowledge Representation for Good OOD Performance
1:35:14 Example: Discovering Cause and Effect
1:36:49 Operating on Sets of Pointable Objects with Dynamically Recombined
1:37:36 RIMS: Modularize Computation and Operate on Sets of Named and Typed Objects
1:39:42 Results with Recurrent Independent Mechanisms
1:40:17 Hypotheses for Conscious Processing by Agents, Systematic Generalization
1:40:46 Conclusions
1:41:06 *[Panel Discussion]*
1:41:59 Connection between Neural Networks as a Computer Science and a Machine Learning Concept - Natural Competition
1:45:35 Idea of Differentiation: Representation and Listening
1:49:36 Alternate to Gradient Based Learning
1:51:04 What is the role of university when Facebook, Google can manage these enormous projects
1:53:50 What do you think students to read?
1:54:50 Mechanisms for Human Level AI
1:57:59 Where do new ideas come from? How do you decide which one works out?
1:59:54 How should I proceed when people writes me reviews and doesn't like my research?
2:01:53 Publications effect on the field
2:05:36 Can we code during AI doing science
2:06:52 What is not General Intelligence, how to measure? and Neural Architecture
2:08:44 Disagreements
02:52 [Talk: Stacked Capsule Autoencoders by Geoffrey Hinton]
36:04 [Talk: Self-Supervised Learning by Yann LeCun]
1:09:37 [Talk: Deep Learning for System 2 Processing by Yoshua Bengio]
1:41:06 [Panel Discussion]
Auto-chaptering powered by VideoKen (videoken.com/)
For indexed video, conftube.com/video/vimeo-390347111
**All Topics**
03:09 Two approaches to object recognition
03:53 Problems with CNNs: Dealing with viewpoint changes
04:42 Equivariance vs Invariance
05:25 Problems with CNNs
10:04 Computer vision as inverse computer graphics
11:55 Capsules 2019: Stacked Capsule Auto-Encoders
13:21 What is a capsule?
14:58 Capturing intrinsic geometry
15:37 The generative model of a capsule auto-encoder
20:28 The inference problem: Inferring wholes from parts
21:44 A multi-level capsule auto-encoder
22:30 How the set transformer is trained
23:14 Standard convolutional neural network for refining word representations based on their context
23:41 How transformers work
24:43 Some difficult examples of MNIST digits
25:20 Modelling the parts of MNIST digits
27:03 How some of the individual part capsules contribute to the reconstructions
28:37 Unsupervised clustering of MNIST digits using stacked capsule autoencoders
31:25 The outer loop of vision
31:36 Dealing with real 3-D images
32:51 Conclusion
36:04 *[Talk: Self-Supervised Learning by Yann LeCun]*
36:25 What is Deep Learning?
38:37 Supervised Learning works but requires many labeled samples
39:25 Supervised DL works amazingly well, when you have data
40:05 Supervised Symbol Manipulation
41:50 Deep Learning Saves Lives
43:40 Reinforcement Learning: works great for games and simulations.
45:12 Three challenges for Deep Learning
47:39 How do humans and animals learn so quickly?
47:43 Babies learn how the world works by observation
48:43 Early Conceptual Acquisition in Infants [from Emmanuel Dupoux]
49:33 Prediction is the essence of Intelligence
50:28 Self-Supervised Learning = Filling in the Blanks
50:53 Natural Language Processing: works great!
51:55 Self-Supervised Learning for Video Prediction
52:09 The world is stochastic
52:43 Solution: latent variable energy-based models
53:55 Self-supervised Adversarial Learning for Video Prediction
54:12 Three Types of Learning
55:30 How Much Information is the Machine Given during Learning?
55:54 The Next Al Revolution
56:23 Energy-Based Models
56:32 Seven Strategies to Shape the Energy Function
57:02 Denoising AE: discrete
58:44 Contrastive Embedding
1:00:39 MoCo on ImageNet
1:00:52 Latent-Variable EBM for inference & multimodal prediction
1:02:07 Learning a (stochastic) Forward Model for Autonomous Driving
1:02:26 A Forward Model of the World
1:04:42 Overhead camera on highway. Vehicles are tracked
1:05:00 Video Prediction: inference
1:05:15 Video Prediction: training
1:05:30 Actual, Deterministic, VAE+Dropout Predictor/encoder
1:05:57 Adding an Uncertainty Cost (doesn't work without it)
1:06:01 Driving an Invisible Car in "Real" Traffic
1:06:51 Conclusions
1:09:37 *[Talk: Deep Learning for System 2 Processing by Yoshua Bengio]*
1:10:10 No-Free-Lunch Theorem, Inductive Biases Human-Level AI
1:15:03 Missing to Extend Deep Learning to Reach Human-Level AI
1:16:48 Hypotheses for Conscious Processing by Agents, Systematic Generalization
1:22:02 Dealing with Changes in Distribution
1:25:13 Contrast with the Symbolic AI Program
1:28:07 System 2 Basics: Attention and Conscious Processing
1:28:19 Core Ingredient for Conscious Processing: Attention
1:29:16 From Attention to Indirection
1:30:35 From Attention to Consciousness
1:31:59 Why a Consciousness Bottleneck?
1:33:07 Meta-Learning: End-to-End OOD Generalization, Sparse Change Prior
1:33:21 What Causes Changes in Distribution?
1:34:56 Meta-Learning Knowledge Representation for Good OOD Performance
1:35:14 Example: Discovering Cause and Effect
1:36:49 Operating on Sets of Pointable Objects with Dynamically Recombined
1:37:36 RIMS: Modularize Computation and Operate on Sets of Named and Typed Objects
1:39:42 Results with Recurrent Independent Mechanisms
1:40:17 Hypotheses for Conscious Processing by Agents, Systematic Generalization
1:40:46 Conclusions
1:41:06 *[Panel Discussion]*
1:41:59 Connection between Neural Networks as a Computer Science and a Machine Learning Concept - Natural Competition
1:45:35 Idea of Differentiation: Representation and Listening
1:49:36 Alternate to Gradient Based Learning
1:51:04 What is the role of university when Facebook, Google can manage these enormous projects
1:53:50 What do you think students to read?
1:54:50 Mechanisms for Human Level AI
1:57:59 Where do new ideas come from? How do you decide which one works out?
1:59:54 How should I proceed when people writes me reviews and doesn't like my research?
2:01:53 Publications effect on the field
2:05:36 Can we code during AI doing science
2:06:52 What is not General Intelligence, how to measure? and Neural Architecture
2:08:44 Disagreements
มุมมอง: 42 741
วีดีโอ
Approximate Bayesian Computation - Part 2
มุมมอง 7995 ปีที่แล้ว
Wednesday, 24th July Time: 09:00 - 11:00 (BST) Table of Contents (powered by videoken.com) 0:03:17 Announcements 0:04:10 About Babylon 0:04:35 Together we can put accessible and affordable healthcare in the hands of every person on earth 0:05:15 We have 3 services 0:06:59 Transforming Healthcare in Rwanda 0:07:23 Babylon is expanding around the world 0:07:49 Areas of research 0:08:52 Some of Ba...
Approximate Bayesian Computation - Part 1
มุมมอง 1.9K5 ปีที่แล้ว
Tuesday, 23rd July Time: 17:30 - 19:30 (BST)
Welcome to IJCAI ECAI 2018 live stream from Stockholm In this keynote Nicole 2184677755006532
มุมมอง 3606 ปีที่แล้ว
Welcome to IJCAI ECAI 2018 live stream from Stockholm In this keynote Nicole 2184677755006532
Welcome to IJCAI ECAI 2018 live stream from Stockholm In this keynote Hector 2184676848339956
มุมมอง 7996 ปีที่แล้ว
Welcome to IJCAI ECAI 2018 live stream from Stockholm In this keynote Hector 2184676848339956
Welcome to IJCAI ECAI 2018 live stream from Stockholm In this keynote Jean F 2184675968340044
มุมมอง 1556 ปีที่แล้ว
Welcome to IJCAI ECAI 2018 live stream from Stockholm In this keynote Jean F 2184675968340044
Awards from IJCAI, the International Joint Conference on Artificial Intellige 2192356910905283
มุมมอง 1.2K6 ปีที่แล้ว
Awards from IJCAI, the International Joint Conference on Artificial Intellige 2192356910905283
The AI Strategy for Europe This session will present the AI strategy announce 2188129301328044
มุมมอง 1696 ปีที่แล้ว
The AI Strategy for Europe This session will present the AI strategy announce 2188129301328044
Welcome to IJCAI ECAI 2018 live stream from Stockholm In this keynote Max Te 2184678991673075
มุมมอง 2916 ปีที่แล้ว
Welcome to IJCAI ECAI 2018 live stream from Stockholm In this keynote Max Te 2184678991673075
IJCAI-ECAI 2018 | Danica Kragic Jensfelt | Collaborative Robots: Challenges and Opportunities.
มุมมอง 5416 ปีที่แล้ว
Watch this video with AI-generated Table of Content (ToC), Phrase Cloud and In-video Search here:
IJCAI-ECAI 2018 and ICML 2018 joint keynote sessions
มุมมอง 9976 ปีที่แล้ว
Watch this video with AI-generated Table of Content (ToC), Phrase Cloud and In-video Search here:
IJCAI-ECAI 2018 | Yann LeCun | Learning World Models: the Next Step Towards AI.
มุมมอง 4K6 ปีที่แล้ว
Watch this video with AI-generated Table of Content (ToC), Phrase Cloud and In-video Search here: videos.videoken.com/index.php/videos/ijcai-ecai-2018-yann-lecun-learning-world-models-the-next-step-towards-ai/
Session 3: Optimization Convex
มุมมอง 6756 ปีที่แล้ว
Watch this video with AI-generated Table of Content (ToC), Phrase Cloud and In-video Search here: videos.videoken.com/index.php/videos/icml-2018-session-3-optimization-non-convex/
Session 3: Deep Learning Neural Network Architectures
มุมมอง 7486 ปีที่แล้ว
Session 3: Deep Learning Neural Network Architectures
Session 3: Deep Learning Neural Network Architectures
มุมมอง 3656 ปีที่แล้ว
Session 3: Deep Learning Neural Network Architectures
Session 2A & 2B: Deep Learning Neural Network Architectures
มุมมอง 5086 ปีที่แล้ว
Session 2A & 2B: Deep Learning Neural Network Architectures
Session 2A & 2B: Reinforcement Learning
มุมมอง 2546 ปีที่แล้ว
Session 2A & 2B: Reinforcement Learning
Session 2A & 2B: Optimization Non Convex
มุมมอง 3286 ปีที่แล้ว
Session 2A & 2B: Optimization Non Convex
Session 1: Parallel and Distributed Learning
มุมมอง 2536 ปีที่แล้ว
Session 1: Parallel and Distributed Learning
Session 1: Deep Learning Neural Network Architectures
มุมมอง 3816 ปีที่แล้ว
Session 1: Deep Learning Neural Network Architectures
thank you for much for this amazing lecture
What is the meaning of no closed form in step 2 of Bayesian inference?
it means that it's impossible to write down on paper a derived solution to the integral. Instead you need to use approximations
Thank you
Wonderful explanation about ABC!
Somehow glucose is more efficient than electricity. 😂
Nice conference
I think i have bettered the understanding on Yashua Bengio on consciousness and meta learning and why deep learning depedendce on bits for computing and inference search algorithm of gradient descent are its limitations to what it can do.
Yesterday i had given a comment on twitter on contrastive learning, i would like to correct my statement that there is dimensionlity redcution but it is in the form of a concept. So, basically its just another way of looking at transfer from an entropy perspective. Comparable entropic systems are similar, hence, i don't see many conceptual different between what transformer q, k, v do and energy function do. Its just another good name.
Hinton' view of vision as hierarchical is not true. In perception, hierarchy does play a role but hardly in vision. Vision is what a 3D mapping by eyes of an external environment which is used as data for infinite dimensional decision making. World exists in infinite dimesnional space of physical planes. Eyes are evolved to create a 3d physical mapping of these planes and passed to human perception engine which makes sense of this using physical and meta physical spaces where human perception wander, although in a very optimized manner(much much better than computing) to find optimized paths or decision making. Those who excel in finding paths which are best are often people excelling in those dimensions whether its chess, football, occult, porn, movies or whatever. However, enhancing a dimesnion is like writing the best function for this nuance. We live in a world of recursion as well as non-recursion of several functions which are either a composition, association or hierarchical. All at the same time.
Thats why i believe colloborating systems of AI will be the best restricting functional space for each subsystem. Pretty much how humans are. However, computer vision best approach is not thinking of a universal computer vision but area restricted and our expectation of things we highly expect in that area, rare events are rare for humans too and then colloborating computer vision systems to form the universal computer vision. Pretty much like a local driver for each area transferring driving to next local expert.
The biggest problem in computer vision is not specific to machine learning and neural networks, as opposed to the inherent way images are encoded for computer processing. This starts with the way most camera sensors work to convert light waves into pixels using the bayer filter. And the output of that is simply a file with a bunch of pixels which are nothing more than 3 numeric values representing red, green and blue color values for each pixel. These numbers are not light waves that are converted by the eye into bio chemical electrical changes as they move from the retina into the visual cortex. And when humans see images on a computer they don't see numbers they see the light waves projected by the monitor or display device. All of that is a big problem in computer vision in many ways, from color perception, to pixel processing, segmentation and so on. For colors the primary problem is that when your eye looks at an image it is very easy to pick out specific color regions, such as a red apple in a bowl of fruit, based on light spectrum values received in the retina. A computer cannot do this because each pixel has a unique r,g,b value and there is no simple way to define what groups of pixels represent a specific color and every pixel has "red" in it. Which means that for computer vision most of the processing involves coming up with some way of determining the relationship between each pixel and the pixel next to it. This is generally called a convolution where you go through a region of pixels and apply a mathematical operation in order to "simplify" it for purposes of generating a statistical relationship over a group of pixels. But that still isn't the same as seeing a "red" apple in the bowl of fruit like the human eye. And that is because the human eye can easily group various light signals together as part of coherent shapes and objects. This grouping is called segmentation in computers and is a difficult task that depends on using some kind of convolution algorithm to try and assign mathematical relationships for this kind of grouping based on large volumes of data. And this is where CNNs come into play for computer vision, but these CNNs do not provide the same ability to process visual signals as humans do because the human visual system has no concept of pixels or convolutions.
Brilliant. Great teacher.
It's should rationally be obvious that to understand artificial neural nets you should understand the dot product. However apparently the weighted sum is a summie thingie. And this is the state of neural network research in 2021.🎃 AI462 neural networks.
Need a better mic...
10:31 what is it you do with the coordinate frame??
"Stacked Capsule Autoencoders" paper has got 37 citations so far. I think Geoff might be onto something here hahaha (not being sarcastic!). Nice presentation! I especially liked the Q/A part. Their presentations were way too abstract for a broad audience as you'd have to have some more context about their particular research area to get something super valuable out of it. Coming from a guy who does this for living in Microsoft and also in his free time. So pretty much all the time I'm awake. We need more fireside chats with AI minds on TH-cam. As for the keynotes I got from this talk: attention and self-supervision will play a significant role in the future of ML research. Also, "crazy" ideas like capsule nets or more generally questioning the current methods is super important - we need researchers who are working without overfitting to h-index as their main metric and instead focus on the long-term goals of improving ML for the greater good.
Thank you for posting this. I loved the Q&A session and even took some notes, it's very intimate and inspiring!!
Can someone use machine learning to clear the damn audio
I like his way of explaining things....truly intuitive
Africa is a country
The introduction starts @3:47 Speaker starts @4:41
12:05 Control theory need not concern itself only with continuous dyn. systems. When you talk about continuous vs. discrete time, for example you're just changing the 'time' set you work with, i.e. a countable infinite set (set of integers, lattices, etc.), and an uncountable dense set (the reals). When you talk about the state-space being continuous or discrete, you talk about whether its a uncountable or countable set, respectively, and even finite countable (state machines, Markov chains, etc). All of these concepts are covered in standard graduate textbooks about dynamical systems and control theory. To claim that control theory does not cover discrete, and RL does is just false.
To Yann Le Cunn: learning to plan complex action sequences actually are very easy to solve. I have a solution
Where's your working notebook?
Bodyguard: How tough are you? Geoffrey Hinton: I classified MNIST digits with 98.7% accuracy. Bodyguard: Yeah? That's not tough. Geoffrey Hinton: Without using the labels. Bodyguard: Sir, here you go, sir. Sorry, sir.
Did they decide that all of them will hit the mic?
I found the better HD resolution of the same video:- facebook.com/icml.imls/videos/432574700590384/
Thanks!
Are the slides available?
what a historical moment. and I was there. what a magic moment to be alive in history of AI
I had to download the video and watch it in VLC, 'cause I couldn't hear a thing
1:56:39 Le Cunn dodged that like Neo, but it came back haha
Hinton's explanation of transformer is the best I've seen so far
What a time to be alive. This is inspiring :) I really wish they had answered the question at the end about The Measure of Intelligence paper. We need better ways to measure AGI and better datasets to train it on.
Pure joy to watch
have the video of them receiving the Turing award come out yet?
Thank you for sharing!!!
CNNs are rubbish! lol
If Hinton says their rubbish, they are rubbish!
Capsule for 2020. Btw for first comment.
According to the definition of this guy of the local optimum, x = 0 is a local optimum of the function y=x^3 !!! 15:15
Slides and Bibliography unsupervised.cs.princeton.edu/deeplearningtutorial.html
26:03 SBEED Convergent RL with Nonlinear Function Approximation.
Good explanation, but why is this method called "variational" Bayes ?
it comes from calculus of variation
Because we are approximating the real but intractable distribution p from distribution family P, by optimizing a simpler but tractable distribution q from family Q. We a therefore varying the distribution from P to Q for the purpose of approximation.