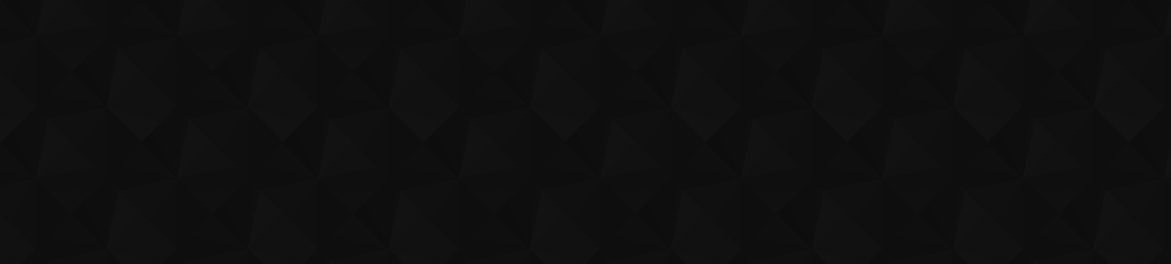
- 44
- 142 849
ML For Nerds
India
เข้าร่วมเมื่อ 10 ธ.ค. 2021
Hi guys! Welcome to My TH-cam Channel. Hope you liked a few of my videos and came here to subscribe.
I am an Advanced Data Scientist with 9+ years of experience in Machine Learning and Deep Learning. I have started this channel for teaching beginners out of passion. Kindly pardon me if there is any delay in uploading the videos as I am a full-time employee. But I will try my best to upload regularly.
Along with youtube, I am regularly blogging on medium on advanced concepts in machine learning. Mostly I do paper reviews on medium. Kindly check it out if interested. - medium.com/@SambasivaraoK
Github Profile: github.com/MLForNerds
Connect with me on LinkedIn for any help - www.linkedin.com/in/sambasivarao-k-886828113/
Discord server for help and queries - discord.com/invite/nfZCszw7Rw
Thank you! Happy Learning!
I am an Advanced Data Scientist with 9+ years of experience in Machine Learning and Deep Learning. I have started this channel for teaching beginners out of passion. Kindly pardon me if there is any delay in uploading the videos as I am a full-time employee. But I will try my best to upload regularly.
Along with youtube, I am regularly blogging on medium on advanced concepts in machine learning. Mostly I do paper reviews on medium. Kindly check it out if interested. - medium.com/@SambasivaraoK
Github Profile: github.com/MLForNerds
Connect with me on LinkedIn for any help - www.linkedin.com/in/sambasivarao-k-886828113/
Discord server for help and queries - discord.com/invite/nfZCszw7Rw
Thank you! Happy Learning!
YOLO-V4: MiWRC, CmBN, DROP BLOCK, CIOU, DIOU || YOLO OBJECT DETECTION SERIES
This video is about Yolo object detection family. This is about YoloV4 which is the most popular and widely used object detector in the industry. YoloV4 has the highest usage by industry for commercial purposes because of its optimal speed and accuracy. In this video, we discussed Multi-Input Weighted Residual Connections, Cross mini Batch Normalization, Drop Block Regularisation, types of IOU losses. These are all parts of Bag of Specials and Bag of Features in YoloV4.
YOLO Playlist:
th-cam.com/play/PL1u-h-YIOL0sZJsku-vq7cUGbqDEeDK0a.html
Neural Networks From Scratch Playlist:
th-cam.com/play/PL1u-h-YIOL0u7R6dg_d5O5M9HUj0SFjHE.html
Link to Papers:
YoloV4: arxiv.org/pdf/2004.10934.pdf
EfficientDet: arxiv.org/pdf/1911.09070.pdf
Cross Batch Norm: arxiv.org/pdf/2002.05712.pdf
DropBlock Regularization: arxiv.org/pdf/1810.12890.pdf
IOU Losses: arxiv.org/pdf/1911.08287.pdf
Chapters:
00:00 Introduction
02:00 Cross Mini-Batch Normalization
11:06 Multi-Input Weighted Residual Connections
17:50 Drop Block Regularization
25:57 IOU Loss
30:32 GIOU Loss
34:29 DIOU Loss
37:29 CIOU Loss
43:06 Conclusion
#yolo #yoloobjectdetection #objectdetection #yolov4 #yolov5 #yolov3 #yolov7 #computervision #imageclassification
YOLO Playlist:
th-cam.com/play/PL1u-h-YIOL0sZJsku-vq7cUGbqDEeDK0a.html
Neural Networks From Scratch Playlist:
th-cam.com/play/PL1u-h-YIOL0u7R6dg_d5O5M9HUj0SFjHE.html
Link to Papers:
YoloV4: arxiv.org/pdf/2004.10934.pdf
EfficientDet: arxiv.org/pdf/1911.09070.pdf
Cross Batch Norm: arxiv.org/pdf/2002.05712.pdf
DropBlock Regularization: arxiv.org/pdf/1810.12890.pdf
IOU Losses: arxiv.org/pdf/1911.08287.pdf
Chapters:
00:00 Introduction
02:00 Cross Mini-Batch Normalization
11:06 Multi-Input Weighted Residual Connections
17:50 Drop Block Regularization
25:57 IOU Loss
30:32 GIOU Loss
34:29 DIOU Loss
37:29 CIOU Loss
43:06 Conclusion
#yolo #yoloobjectdetection #objectdetection #yolov4 #yolov5 #yolov3 #yolov7 #computervision #imageclassification
มุมมอง: 2 862
วีดีโอ
Batch Normalization - Part 4: Python Implementation on MNIST dataset
มุมมอง 52711 หลายเดือนก่อน
We have been discussing Batch Normalization in detail. We have seen why do we need Batch Normalization and we have dig deeper into how Batch Normalization works and also understood the significance of learnable parameters called Gamma and Beta which are Scaling and Shifting. We also saw the Backpropagation for Batch Normalization layer and how Batch Normalization works during inference without ...
Batch Normalization - Part 3: Backpropagation & Inference
มุมมอง 1.4Kปีที่แล้ว
We have been discussing Batch Normalization in detail. We have seen why do we need Batch Normalization and we have dig deeper into how Batch Normalization works and also understood the significance of learnable parameters called Gamma and Beta which are Scaling and Shifting. In this video, we will see the Backpropagation for Batch Normalization layer and also see how Batch Normalization works d...
Batch Normalization - Part 2: How it works & Essence of Beta & Gamma
มุมมอง 1.4Kปีที่แล้ว
We have been discussing Batch Normalization in detail. We have seen why do we need Batch Normalization in the previous video. In this video, we will dig deeper into how Batch Normalization works and also understand the significance of learnable parameters called Gamma and Beta which are Scaling and Shifting. Deep Learning Projects playlist: th-cam.com/play/PL1u-h-YIOL0s2GYHiaemx7-o-iWrht_Lk.htm...
Batch Normalization - Part 1: Why BN, Internal Covariate Shift, BN Intro
มุมมอง 3.6Kปีที่แล้ว
In this video, we dig deeper into “Why do we need Batch Normalization?” And Internal Covariate Shift. Deep Learning Projects playlist: th-cam.com/play/PL1u-h-YIOL0s2GYHiaemx7-o-iWrht_Lk.html Neural Networks From Scratch in Python: th-cam.com/play/PL1u-h-YIOL0u7R6dg_d5O5M9HUj0SFjHE.html Chapters: 00:00 Introduction 02:52 Issues with NN Training w/o BN 04:00 Internal Covariate Shift 04:28 What ar...
Neural Networks From Scratch - Lec 24 - Regression Losses - Mean Square Logarithmic Error
มุมมอง 614ปีที่แล้ว
Building Neural Networks from scratch in python. This is the twenty fourth video of the course - "Neural Networks From Scratch". This video covers most commonly used loss functions we use in regression problems. We discussed the important properties of log function and its significance to regression problems. We also saw Mean Square Log Error and its advantages and drawbacks. We also saw the py...
YOLO-V4: CSPDARKNET, SPP, FPN, PANET, SAM || YOLO OBJECT DETECTION SERIES
มุมมอง 10Kปีที่แล้ว
This video is about Yolo object detection family. This is about YoloV4 which is the most popular and widely used object detector in the industry. YoloV4 has the highest usage by industry for commercial purposes because of its optimal speed and accuracy. In this video, we discussed about Backbone CSPDarknet-53, SPP, FPN, PANT and SAM modules. These are all parts of Bag of Specials in YoloV4. YOL...
YOLO-V4: Optimal Speed & Accuracy || YOLO OBJECT DETECTION SERIES
มุมมอง 7Kปีที่แล้ว
This video is about Yolo object detection family. This is about YoloV4 which is the most popular and widely used object detector in the industry. YoloV4 has the highest usage by industry for commercial purposes because of it's optimal speed and accuracy. YOLO Playlist: th-cam.com/play/PL1u-h-YIOL0sZJsku-vq7cUGbqDEeDK0a.html Neural Networks From Scratch Playlist: th-cam.com/play/PL1u-h-YIOL0u7R6...
YOLO-V3: An Incremental Improvement || YOLO OBJECT DETECTION SERIES
มุมมอง 8Kปีที่แล้ว
This video is about Yolo object detection family. In this video, we dig deeper into Yolo-v3 object detection model, which is an incremental update over YoloV2. This was the Sate of the art object detector and faster object detector back then when it was released. YOLO Playlist: th-cam.com/play/PL1u-h-YIOL0sZJsku-vq7cUGbqDEeDK0a.html Neural Networks From Scratch Playlist: th-cam.com/play/PL1u-h-...
YOLO-9000 - An Object Detector for 9000 classes || YOLO OBJECT DETECTION SERIES
มุมมอง 3.7Kปีที่แล้ว
This video is about yolo object detection family. In this video, we will dig deeper into yolo9000 model which can detect objects from 9000 categories. This is an extension of YOLOv2 for detecting objects at largest scale. This uses Darknet 19 backbone for yolo. YOLO Playlist: th-cam.com/play/PL1u-h-YIOL0sZJsku-vq7cUGbqDEeDK0a.html Neural Networks From Scratch Playlist: th-cam.com/play/PL1u-h-YI...
YOLO V2 - Better, Faster & Stronger || YOLO OBJECT DETECTION SERIES || YOLO9000
มุมมอง 12Kปีที่แล้ว
This is the second video of the series about YOLO object detection model family. This video digs deeper into YOLO-V2 paper which is an improvement over Yolo-V1. This version of yolo object detector is much more accurate and faster than yolo v1. They have made an new architecture named darknet 19 for backbone which is more accurate and less complex. YOLO Playlist: th-cam.com/play/PL1u-h-YIOL0sZJ...
YOLO V1 - YOU ONLY LOOK ONCE || YOLO OBJECT DETECTION SERIES
มุมมอง 35Kปีที่แล้ว
Hi Guys, I am starting a new series about YOLO object detection model family. This is not an overview series, we will dig deeper into every detail of these yolo object detectors. Everyone uses YOLO models, they are the state of the art models for object detection. Hope you learn something from these videos. YOLO Object Detection Series: th-cam.com/play/PL1u-h-YIOL0sZJsku-vq7cUGbqDEeDK0a.html PD...
Neural Networks From Scratch - Lec 23 - Regression Losses - Smooth L1 Loss and Huber Loss Functions
มุมมอง 1.5Kปีที่แล้ว
Building Neural Networks from scratch in python. This is the twenty third video of the course - "Neural Networks From Scratch". This video covers most commonly used loss functions we use in regression problems. We discussed Smooth L1 loss and Huber loss and their differences. Neural Networks From Scratch Playlist: th-cam.com/users/playlist?list... Please like and subscribe to the channel for mo...
Remove the confusion once for all! Cost Function vs Loss Function vs Objective Function
มุมมอง 1.1Kปีที่แล้ว
In this video, we have resolved the confusion between the most commonly used loss terms in machine learning. What is loss function? What is cost function? Are they same? Time stamps: 00:00 Introduction 00:22 What do you think? 00:35 Answer! Difference between them 02:02 Illustration with Example 03:10 One more difference 03:45 What is Objective Function then? 04:59 Conclusion #loss #objective #...
Neural Networks From Scratch - Lec 22 - MAE vs RMSE, Comparison with an Example
มุมมอง 979ปีที่แล้ว
Building Neural Networks from scratch in python. This is the twenty second video of the course - "Neural Networks From Scratch". This video covers the similarities an important differences between MAE loss and RMSE loss functions, How do we interpret them and which one to prefer. Code Link: github.com/MLForNerds/Neural_network_from_scratch/blob/main/MAE_RMSE.ipynb Neural Networks From Scratch P...
What is Numpy? Why Numpy arrays are faster than python lists?
มุมมอง 584ปีที่แล้ว
What is Numpy? Why Numpy arrays are faster than python lists?
MNIST Classification: Hands-on Project in PyTorch 1.12
มุมมอง 529ปีที่แล้ว
MNIST Classification: Hands-on Project in PyTorch 1.12
PyTorch Vs Tensorflow: Jobs, Research and Industries. Who is the winner in 2022?
มุมมอง 1.6Kปีที่แล้ว
PyTorch Vs Tensorflow: Jobs, Research and Industries. Who is the winner in 2022?
MNIST Classification: Hands-on Project in Tensorflow 2.8
มุมมอง 4352 ปีที่แล้ว
MNIST Classification: Hands-on Project in Tensorflow 2.8
Building a Neural Network from scratch: MNIST Project (No Tensorflow/Pytorch, Just Numpy)
มุมมอง 13K2 ปีที่แล้ว
Building a Neural Network from scratch: MNIST Project (No Tensorflow/Pytorch, Just Numpy)
Neural Networks From Scratch - Lec 21 - Regression Losses - MSE & RMSE
มุมมอง 9112 ปีที่แล้ว
Neural Networks From Scratch - Lec 21 - Regression Losses - MSE & RMSE
Neural Networks From Scratch - Lec 20 - Regression Losses - MAE, MAPE & MBE
มุมมอง 1.1K2 ปีที่แล้ว
Neural Networks From Scratch - Lec 20 - Regression Losses - MAE, MAPE & MBE
Neural Networks From Scratch - Lec 19 - Approaching Regression Problem with Neural Networks
มุมมอง 8192 ปีที่แล้ว
Neural Networks From Scratch - Lec 19 - Approaching Regression Problem with Neural Networks
Neural Networks From Scratch - Lec 18 - Typical Neural Network Training Setup
มุมมอง 6992 ปีที่แล้ว
Neural Networks From Scratch - Lec 18 - Typical Neural Network Training Setup
Neural Networks From Scratch - Lec 17 - Python Implementations of all Activation functions
มุมมอง 1K2 ปีที่แล้ว
Neural Networks From Scratch - Lec 17 - Python Implementations of all Activation functions
Neural Networks From Scratch - Lec 16 - Summary of all Activation functions in 10 mins
มุมมอง 9812 ปีที่แล้ว
Neural Networks From Scratch - Lec 16 - Summary of all Activation functions in 10 mins
Neural Networks From Scratch - Lec 15 - GeLU Activation Function
มุมมอง 6K2 ปีที่แล้ว
Neural Networks From Scratch - Lec 15 - GeLU Activation Function
Neural Networks From Scratch - Lec 14 - Mish Activation Function
มุมมอง 1.2K2 ปีที่แล้ว
Neural Networks From Scratch - Lec 14 - Mish Activation Function
Neural Networks From Scratch - Lec 13 - Swish Activation Function
มุมมอง 1.3K2 ปีที่แล้ว
Neural Networks From Scratch - Lec 13 - Swish Activation Function
Neural Networks From Scratch - Lec 12 - Softplus Activation Function
มุมมอง 8892 ปีที่แล้ว
Neural Networks From Scratch - Lec 12 - Softplus Activation Function
for tx ty they are relative to grid cell why we are not multiplying with 64 like in yolov1
This implementation has overflow-error with very small or large values for x
bro start making videos once again, your explanataions are very good
Thanks a lot for this video, this was really helpful
Thanks a lot for your video, this helped me a lot to understand its working
Also, how is it possible that beta/gamma make a non-symmetric, non-normal distribution like in your picture? It can only give a different normal distribution that may not have mean 0 and std 1. But it's a linear transformation of a std normal, so it must still be normally distributed. Right?
I'm a little confused...you say at around 10:21 that in each mini batch we normalize the x's, which usually denotes the inputs and this doesn't seem like what I think of as batch normalization. But then you go on to say in the visual explanation afterwards what I expected and what I think is correct which is that we normalize the Zs in layers after the input layer before sending them to the activations. So, to be be clear: we don't normalize the batch inputs themselves, just the Zs, right? Or is it both? By the way, this is excellent material--it's just that this one point was confusing me a little
woah, i am happy i found this!
This is amazing could you do a transformer series!
Thanks
Glad! i found this legendary explanation
Best
Bro ❤ that's so better than 200 usdt lectures
hiii will u post yolo v5 ? this is the best playlist
thank you! clear and direct.
Thank you very much for the best explaination of yolo papers on youtube. I have a question of loss calculation on multiscale training. This affects the number of output grid (WxHXS) used in loss calculation when input image(WxH) size changes. How does the loss calculation maintain consistency for this training scheme?
could you please mention source of the mathematical explanations it would be great help.
The best explanations of YOLO on TH-cam. Period. Thank you!🙏
really great job sir! waiting for more lately versions
You rock!!! It was very detailed. Clearly, you have out a lot of work into this. Thank you so much🙏🙏🙏🙏🙏🙏
Just wow!
The best explanation for this concept, kindly keep making such content. Thanks a lot.
thanks for giving us perfect video, love you bro
thanks for helping us a lot in learning, truly appreciate your work
very detailed explanation, Thanks for making it more clear. I believe i didn't find any such video with the way you explained the things in deep. I have a doubt when you said total loss = obj loss+no obj loss, In the example you considered only 2 grid cells has an object which means obj loss is calculated for those 2 grid cells and remaining 47 grid cells falls under no obj loss right?
no
Underrated. Keep going man!
thank you i could have nerver learn and understand any better then your video
i still want to know how to code this stuff up? theory can be found everywhere but how to dive into doing it ourselves?
this is such a great vid
great explanation! thanks!
Very cool project! I have only one question: based on the video, you achieve 71.6% accuracy for 10 epochs, but I can't go above 52-54%. However, you also only have 56.94% accuracy in your github project. What is the reason of this? I'm very new to DL/MI, that's why I'm asking. I tried so much that I went up to 20 epochs, but the end result was almost exactly the same as that of 10 epochs. However, the accuracy increased by half per epoch... Thank you very much for any suggestions!
u can try increase learning rate like (0.1,0.5).. it will gradually increase the accuracy , but also note that extreme Learning rate also lead to skiping ..and dont run entire program every time if you run entire program it will reset the all the values so run only part of training part alone jupiter nb and no of times running the training part = reducing the loss, and a=increases the accuracy if accuracy is there might be logic error
Just like sir said the higher level feature map or smalle feature map are mainly for bigger object and lower level feature map or bigger feature map are for smaller object,due to this various scale are neded for differenet feature map , can anyone tell me in simple words what is 'scale' here
One of the best I have come across so far, Could you please continue the series.
Great work on the explanation, very well summarized!
nice video maboy, the best theorical explanation of why numpy is that good
great video, now I finally understand it :) could you just please clarify why in 22:32 only 2 grid cells contain objects? the woman appears in a few other cells as well, so why only two?
Wherever the object centroid falls, only those cells are considered
@@MLForNerds thank you!! just to make sure that I understand correctly - in this example, one cell has a centroid for the horse and one has a centroid for the person? also, are you planning on making a video on Yolo v7? :)
Yes, you are right regarding object centers. I will continue this series and finish all yolo versions
IM EATING THIS UP THANK YOU
Hello Sir First upon thanks for your all videos, Sir i request you please upload Video on YOLOv8 architecture and How to improve accuracy in yolov8 model.
Fantastic explaination. Super clear
underrated af
WOW what a clear and simple explanation
please make video soon on remaining yolo variants
thanks!
what a great material - so well structured. thx for your efforts - beautiful!!!
Dear Author, thx for your great work! you are awesome!
Best YoloV3 video!
Excellent Explanation . Will not forget concept now till going to grave
Hi! thank you for your wonderfull explanation! Unfortunately in the original paper there are many unclear moments. Your video helped me a lot. But i still have some questions. 1) "Grid cell is "responsible" if the center of bbox falls into it." In training data we have annotated bboxes. But in test data there are no annotated bboxes and therefore centers. So which grid cell will be "responsible" in that case? 2) if c < threshold, then we simply nullify all the values in the vector or we should train the model to nullify the vector on its own? 3) if only 2 grid cells (in your case) predict the coordinates of bboxes, what is the use of the other 47 grid cells (are the useless at all or not?) 4) How one small grid cell (64x64) predicts a box for an object that is a way bigger than this cell (450x450)? 5) Why you are telling that there are only 2 object cells, if the woman overlap at least 6 cells? Maybe you mean only 2 "responsible" cells?
And, I have a question for the "Not 0 centered function" section : What if you use the activation function only up to the last hidden layer. You just don't put any to get the final result.. The weights between the hidden layer and the output might be negative and the backpropagation won't be changing the sign of it.. will it ?
Yes it's aaawesome !! I was searching a beginner video for weeks but they are all only "beginner friendly" by name.. It comes really fast into complicated maths subjects and you don't get to understand the compution process behing it.. You explain everything :) it's really cool ! TY