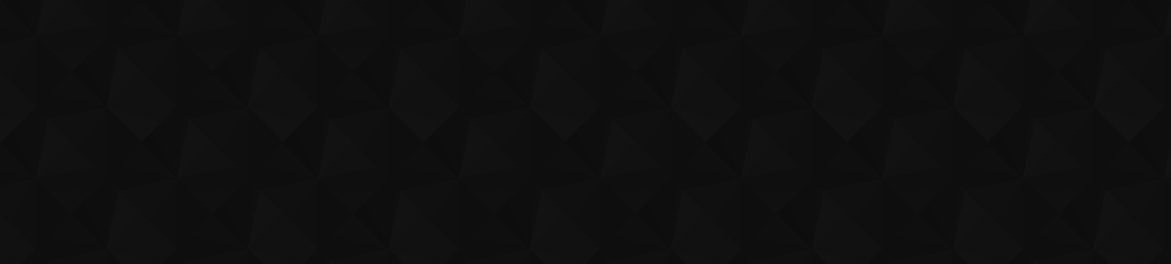
- 25
- 45 962
Generative Memory Lab
เข้าร่วมเมื่อ 26 ก.พ. 2021
Critical windows: non-asymptotic theory for feature emergence in diffusion models
Marvin Li presents the paper "Critical windows: non-asymptotic theory for feature emergence in diffusion models" arxiv.org/pdf/2403.01633
มุมมอง: 311
วีดีโอ
Discrete diffusion modeling by estimating the ratios of the data distribution
มุมมอง 4.6K8 หลายเดือนก่อน
Aaron Lou presents the paper "Discrete diffusion modeling by estimating the ratios of the data distribution" arxiv.org/abs/2310.16834v2
Generalization, hallucinations and memorization in diffusion models
มุมมอง 9109 หลายเดือนก่อน
The "question and discussion" section after the talk from Rylan Schaeffer became a very interesting conversation on learning and memorization in diffusion models.
Bridging Associative Memory and Probabilistic Modeling
มุมมอง 4.2K9 หลายเดือนก่อน
Rylan Schaeffer gives a presentation on the link between Associative Memory and Probabilistic Modeling.
Spontaneous symmetry breaking in generative diffusion models
มุมมอง 2Kปีที่แล้ว
Gabriel Raya presents his paper "Spontaneous symmetry breaking in generative diffusion models" openreview.net/forum?id=lxGFGMMSVl Link to Blog Post: gabrielraya.com/blog/2023/symmetry-breaking-diffusion-models/ Link to Github repo: github.com/gabrielraya/symmetry_breaking_diffusion_models
Action Matching: Learning Stochastic Dynamics from Samples
มุมมอง 478ปีที่แล้ว
Kirill Neklyudov presents his paper "Action Matching: Learning Stochastic Dynamics from Samples" arxiv.org/abs/2210.06662
Blackout Diffusion: Generative Diffusion Models in Discrete-State Spaces
มุมมอง 832ปีที่แล้ว
Yen Ting Lin presents his paper "Blackout Diffusion: Generative Diffusion Models in Discrete-State Spaces" arxiv.org/abs/2305.11089
Reflected Diffusion Models
มุมมอง 448ปีที่แล้ว
Aaron Lou presents his paper "Reflected Diffusion Models" arxiv.org/abs/2304.04740
Diffusion models as plug-and-play priors
มุมมอง 2.1Kปีที่แล้ว
Alexandros Graikos presents his paper "Diffusion models as plug-and-play priors" arxiv.org/pdf/2206.09012.pdf
Building Normalizing Flows with Stochastic Interpolants
มุมมอง 2.8Kปีที่แล้ว
Michael S Albergo presents his paper °Building Normalizing Flows with Stochastic Interpolants° arxiv.org/abs/2209.15571
Hierarchically branched diffusion models
มุมมอง 479ปีที่แล้ว
Alex M. Tseng presents the paper "Hierarchically branched diffusion models for efficient and interpretable multi-class conditional generation" arxiv.org/abs/2212.10777
Planning with Diffusion for Flexible Behavior Synthesis
มุมมอง 6Kปีที่แล้ว
Yilun Du, PhD student at MIT EECS, presents the paper 'Planning with Diffusion for Flexible Behavior Synthesis' arxiv.org/pdf/2205.09991.pdf
Diffusion Models for Inverse Problems
มุมมอง 17Kปีที่แล้ว
Hyungjin Chung presents his papers: "Diffusion posterior sampling for general noisy inverse problems" arxiv.org/pdf/2209.14687.pdf "Improving diffusion models for inverse problems using manifold constraints" arxiv.org/pdf/2206.00941.pdf
Back to the Manifold: Recovering from Out-of-Distribution States
มุมมอง 2352 ปีที่แล้ว
Alfredo Reichlin presents his paper: "Back to the Manifold: Recovering from Out-of-Distribution States"
KALE Flow: A Relaxed KL Gradient Flow For Probabilities With Disjoint Support
มุมมอง 752 ปีที่แล้ว
Pierre Glaser presents his paper "KALE Flow: A Relaxed KL Gradient Flow For Probabilities With Disjoint Support" proceedings.neurips.cc/paper/2021/file/433a6ea5429d6d75f0be9bf9da26e24c-Paper.pdf
Probabilistic Circuits and Any-Order Autoregression
มุมมอง 2612 ปีที่แล้ว
Probabilistic Circuits and Any-Order Autoregression
Transport Score Climbing: Variational Inference Using ForwardKL and Adaptive Neural Transport
มุมมอง 1032 ปีที่แล้ว
Transport Score Climbing: Variational Inference Using ForwardKL and Adaptive Neural Transport
Decoupling Exploration and Exploitation for Meta-Reinforcement Learning without Sacrifices
มุมมอง 2212 ปีที่แล้ว
Decoupling Exploration and Exploitation for Meta-Reinforcement Learning without Sacrifices
A learning gap between neuroscience and reinforcement learning, Samuel Wauthier and Pietro Mazzaglia
มุมมอง 1412 ปีที่แล้ว
A learning gap between neuroscience and reinforcement learning, Samuel Wauthier and Pietro Mazzaglia
Recurrent Model-Free RL is a Strong Baseline for Many POMDPs
มุมมอง 2722 ปีที่แล้ว
Recurrent Model-Free RL is a Strong Baseline for Many POMDPs
Scalable Bayesian Deep Learning with Modern Laplace Approximations
มุมมอง 5813 ปีที่แล้ว
Scalable Bayesian Deep Learning with Modern Laplace Approximations
ADAVI: Automatic Dual Amortized Variational Inference Applied To Pyramidal Bayesian Models
มุมมอง 713 ปีที่แล้ว
ADAVI: Automatic Dual Amortized Variational Inference Applied To Pyramidal Bayesian Models
Argmax Flows and Multinomial Diffusion: Learning Categorical Distributions
มุมมอง 2.1K3 ปีที่แล้ว
Argmax Flows and Multinomial Diffusion: Learning Categorical Distributions
Continuously-Indexed Normalizing Flows - Increasing Expressiveness by Relaxing Bijectivity.
มุมมอง 1183 ปีที่แล้ว
Continuously-Indexed Normalizing Flows - Increasing Expressiveness by Relaxing Bijectivity.
Targeted likelihood-free inference for dark matter searches in strong lensing images.
มุมมอง 2133 ปีที่แล้ว
Targeted likelihood-free inference for dark matter searches in strong lensing images.
Presenter totally unprepared, neither confident about nor even familiar with diffusion process.
Worthy!!
This will be GPT-7
Thanks for posting this! It's very helpful to have this next to reading the paper! 📃
great work! Intriguing way to modeling natural languages
I wish the audio had been processed to eliminate the compression aberrations.
Please continue producing those talks and publishing them here, they are incredibly useful!
gradually, then suddenly 🦆🧬⚡
Your team would benefit from watching my AI Self Awareness videos.
Thank you. This is most useful seeds of continued work.
This is fascinating! These presentations are very easy to follow and even if you are not in this field, it still show an exceptional understanding of systems thinking.
Thanks! These are fascinating topics!
Super interesting. Thanks for posting this.
Gold video!
Amazing presentation Gabriel!
Amazing talk, thanks!
I think I missed the high level, what is the SoTA technology here, what applications? Mostly for reversing complicated smudges and blurring? Other applications?
I have one question: Why is it that we can factorize as shown at 12:44 given that x_0 is independent on y and x_t?
Excellent talk. Very enlightening! ❤
On the minus sign comment, the confusion arises from the fact that we call this a reverse diffusion process. Its not - its conditioned on the highest probability of the distribution function or any transformation of it. If you you were to plot the two diffusions (forward and conditional), they look completely different. Anyways, minus sign because the gradient will reverse your sign to keep you on the highest probability ridge.
dt is negative in the reverse SDE and positive in the forward SDE. See paragraph under (6) of arXiv:2011.13456v2. Intuitively, we can understand the sign by taking g(t) to 0. Then the evolution is deterministic, and governed only by the drift force f(x,t) in the forward direction. Since this process is Markovian, the reverse process is simply dx = -f(x,t) |dt|.
great talk, thanks for sharing! (LHS in slides 18-21 should be p(y|x_t))
Wonderful work and wonderful talk!
Hi, this paper is accepted to ICML 2022, and this is the official talk th-cam.com/video/tpofgxbi8pU/w-d-xo.html
This video is GOLD. Bad news: only 130 view count. Good news: I found it. Thank you for sharing this awesome seminar in public.
There are interesting methods in those papers. Thank you for a short and clear presentation. I wish you all the best in your research.