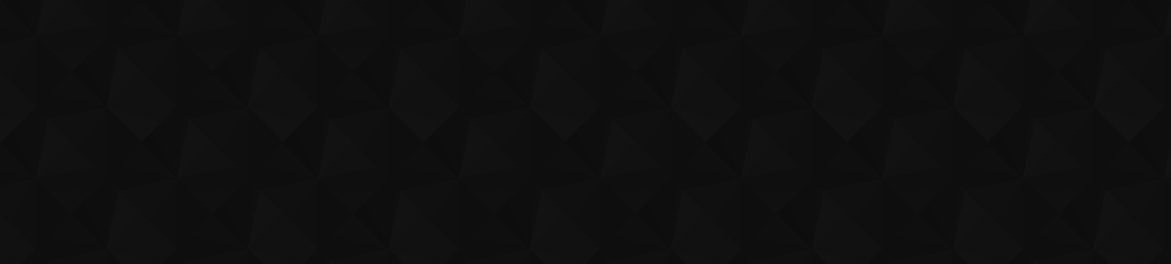
- 56
- 42 627
Formal Languages and Neural Networks Seminar
เข้าร่วมเมื่อ 12 ก.พ. 2022
Yash Sarrof: The Expressive Capacity of State Space Models: A Formal Language Perspective
Talk given by Yash Sarrof to the Formal Languages and Neural Networks discord on June 10, 2024. Thank you, Yash!
Please find the link to their paper here:
arxiv.org/abs/2405.17394
Please find the link to their paper here:
arxiv.org/abs/2405.17394
มุมมอง: 253
วีดีโอ
Anton Xue: Logicbreaks: A Framework for Understanding Subversion of Rule-based Inference
มุมมอง 44หลายเดือนก่อน
Talk given by Anton Xue to the Formal Languages and Neural Networks discord on June 10, 2024. Thank you, Anton! Please find the link to their paper here: arxiv.org/abs/2407.00075
Zhiyuan Li: Chain Of Thought Empowers Transformers To Solve Inherently Serial Problems
มุมมอง 413หลายเดือนก่อน
Talk given by Zhiyuan Li to the Formal Languages and Neural Networks discord on August 19, 2024. Thank you, Zhiyuan! Please find the link to their paper here: arxiv.org/abs/2402.12875
Yingshan Chang: Language Models Need Inductive Biases to Count Inductively
มุมมอง 74หลายเดือนก่อน
Talk given by Yingshan Chang to the Formal Languages and Neural Networks discord on July 15, 2024. Thank you, Yingshan! Please find the link to their paper here: arxiv.org/abs/2310.07923
Alessandro Ronca: On the Expressivity of Recurrent Neural Cascades
มุมมอง 145หลายเดือนก่อน
Talk given by Alessandro Ronca to the Formal Languages and Neural Networks discord on June 24, 2024. Thank you, Alessandro! Please find the link to their paper here: ojs.aaai.org/index.php/AAAI/article/view/28929
Martin Berger: Fast grammar inference on GPUs
มุมมอง 1673 หลายเดือนก่อน
Talk given by Martin Berger to the Formal Languages and Neural Networks discord on June 17, 2024. Thank you, Martin! Please find the link to their papers here: dl.acm.org/doi/10.1145/3591274 arxiv.org/abs/2402.12373
Will Merrill: The Expressive Power of Transformers with Chain of Though
มุมมอง 1863 หลายเดือนก่อน
Talk given by Will Merrill to the Formal Languages and Neural Networks discord on June 10, 2024. Thank you, Will! Please find the link to their paper here: arxiv.org/abs/2310.07923
Daniel Hsu: Transformers, parallel computation and logarithmic depth
มุมมอง 2854 หลายเดือนก่อน
Talk given by Daniel Hsu to the Formal Languages and Neural Networks discord on May 27, 2024. Thank you, Danuel! Please find the link to their paper here: arxiv.org/abs/2402.09268
Michaël Rizvi: Simulating Weighted Automata over Sequences and Trees with Transformers
มุมมอง 1215 หลายเดือนก่อน
Talk given by Michaël Rizvi to the Formal Languages and Neural Networks discord on May 13, 2024. Thank you, Michaël! Please find the link to their paper here: arxiv.org/abs/2403.09728
Mark Rofin: Why are Sensitive Functions Hard for Transformers?
มุมมอง 3255 หลายเดือนก่อน
Talk given by Mark Rofin to the Formal Languages and Neural Networks discord on April 29, 2024. Thank you, Mark! Please find the link to their paper here: arxiv.org/abs/2402.09963
Brian DuSell: Stack Attention
มุมมอง 1775 หลายเดือนก่อน
Talk given by Brian DuSell to the Formal Languages and Neural Networks discord on April 22, 2024. Thank you, Brian! Please find the link to their paper here: arxiv.org/abs/2310.01749
Will Merrill: The Illusion of State in State-Space Models
มุมมอง 1.5K6 หลายเดือนก่อน
Talk given by Will Merrill to the Formal Languages and Neural Networks discord on April 1st 2024. Thank you, Will! Their paper will be available on ArXiv soon and will be updated here once online!
Nur Lan: Bridging the Empirical-Theoretical Gap in Neural Network Formal Language Learning
มุมมอง 3296 หลายเดือนก่อน
Talk given by Nur Lan to the Formal Languages and Neural Networks discord on March 25, 2024. Thank you, Nur! Full Title: Bridging the Empirical-Theoretical Gap in Neural Network Formal Language Learning Using Minimum Description Length Please find the link to their paper here: arxiv.org/abs/2402.10013
Dylan Zhang: Transformer-Based Models Are Not Yet Perfect At Learning 2 Emulate Structural Recursion
มุมมอง 2467 หลายเดือนก่อน
Talk given by Dylan Zhang to the Formal Languages and Neural Networks Discord on March 11, 2024. Thank you, Dylan! Please find the link to their paper here: arxiv.org/abs/2401.12947
Giuseppe De Giacomo
มุมมอง 1087 หลายเดือนก่อน
Talk given by Giuseppe De Giacomo to the Formal Languages and Neural Networks Discord on March 4, 2024. Thank you, Giuseppe! Please find the link to their paper here: arxiv.org/abs/2310.13897
Hattie Zhou: What Algorithms can Transformers Learn? A Study in Length Generalization
มุมมอง 5457 หลายเดือนก่อน
Hattie Zhou: What Algorithms can Transformers Learn? A Study in Length Generalization
Alexander Kozachinskiy: Logical Languages Accepted by Transformer Encoders with Hard Attention
มุมมอง 1337 หลายเดือนก่อน
Alexander Kozachinskiy: Logical Languages Accepted by Transformer Encoders with Hard Attention
Satwik Bhattamishra: Simplicity Bias in Transformers & their Ability to Learn S. Boolean Functions
มุมมอง 1687 หลายเดือนก่อน
Satwik Bhattamishra: Simplicity Bias in Transformers & their Ability to Learn S. Boolean Functions
Andy Yang: Masked Hard-Attention Transformers and B-RASP Recognize Exactly the Star-Free Languages
มุมมอง 907 หลายเดือนก่อน
Andy Yang: Masked Hard-Attention Transformers and B-RASP Recognize Exactly the Star-Free Languages
Bohang Zhang: Towards Revealing the Mystery behind Chain of Thought: A Theoretical Perspective
มุมมอง 1487 หลายเดือนก่อน
Bohang Zhang: Towards Revealing the Mystery behind Chain of Thought: A Theoretical Perspective
Clayton Sanford: Representational Strengths and Limitations of Transformers
มุมมอง 31911 หลายเดือนก่อน
Clayton Sanford: Representational Strengths and Limitations of Transformers
Nouha Dziri: Faith and Fate: Limits of Transformers on Compositionality
มุมมอง 1.4Kปีที่แล้ว
Nouha Dziri: Faith and Fate: Limits of Transformers on Compositionality
Dan Friedman: Learning Transformer Programs
มุมมอง 1.5Kปีที่แล้ว
Dan Friedman: Learning Transformer Programs
Jenny Kunz: Where Does Linguistic Information Emerge in Neural Language Models?
มุมมอง 124ปีที่แล้ว
Jenny Kunz: Where Does Linguistic Information Emerge in Neural Language Models?
Alexandra Butoi: Convergence and Diversity in the Control Hierarchy
มุมมอง 68ปีที่แล้ว
Alexandra Butoi: Convergence and Diversity in the Control Hierarchy
David Chiang: Tighter Bounds on the Expressivity of Transformer Encoders
มุมมอง 148ปีที่แล้ว
David Chiang: Tighter Bounds on the Expressivity of Transformer Encoders
Martin Grohe: The Descriptive Complexity of Graph Neural Networks
มุมมอง 248ปีที่แล้ว
Martin Grohe: The Descriptive Complexity of Graph Neural Networks
Ryan Cotterell: Optimally encoding PFSAs as RNNs
มุมมอง 149ปีที่แล้ว
Ryan Cotterell: Optimally encoding PFSAs as RNNs
Justin DeBenedetto: Representing Unordered Data Using Complex-Weighted Multiset Automata
มุมมอง 26ปีที่แล้ว
Justin DeBenedetto: Representing Unordered Data Using Complex-Weighted Multiset Automata
Thank you for uploading this! So informative!
Interesting talk Yash 🎉
Interesting!
Good going. ❤❤ keep it up 👍
10:50 It's a bit unfair to say that (aa)* is parity + neutral symbol, especially when you tie things with circuit complexity: the regular languages of AC0 include (aa)* but not parity, so there's a quantifiable gap in computing power needed to express one and not the other. In general, in the classical study, there's not much of a difference in treatment between L[<] and L[<, mod, +1] - we have generic techniques to translates results (e.g., decidability) from one to the other. Is there something like this that can be artificially added to SSM so that they can do (aa)* but not parity? Oh and second question, what about bounded Dyck with 2 sets of parentheses?
Yep I agree, Calling Parity : (aa)* + neutral symbol was just a way to refer to the definition of Parity. I didn't mean to imply that Parity is as simple as (aa)*. Rather in our proof we treat (aa)* as a subset of Parity (solving Parity would require solving (aa)*) as well. However SSMs are unable to solve (aa)* and thus by extension Parity as well. Regarding something that can be artificially added to SSMs for them to be able to do (aa)* and not Parity. I am not sure, that's a trivial question to answer. We tried to increase the expressivity of SSMs by removing the non-negative assumption in Mamba (replacing the exponentiation with other choices), however, that didn't turn out to be helpful, as breaking the Non-negative assumption broke training, and we weren't able to converge on anything. However, we haven't explored this problem in as much detail, and there could be other ways of doing the same, but that would require breaking either of the Non-negative or Time invariant assumptions as per our findings. Exactly how one does that, without losing out on the advantages offered by SSMs is an open question. Regarding Bounded Dyck with 2 sets of parantheses, the definition of Bounded Dyck(k, m) implies 'k' different kinds of brackets (closing, opening considered different as well) and a depth of m. So, our results and theorems apply to multiple sets of Paranthesis, provided the sets of paranthesis is finite. However, the construction shown in the video was only for 1 set of parentheses. For keeping track of multiple brackets, we again would need flip flop state tracking. The 1st layer would keep track of the depth of the bracket as well as the identity of the bracket. Therefore the space of activations is - {0, ... m} X {all bracket types}. For the 2nd layer, we need to have for each depth, a separate set-reset automaton. With 'm' such automatons, at each depth, we will be able to identify the last bracket at that depth. We can thus deduce the maximum depth at which the last bracket is an opening one, and accordingly be able to predict the next set of valid characters. Thus, we need 'm' automatons that can be simulated by a width of logK , so in total a dimension of O(m logk). I hope I was able to answer your questions !
I don't agree with the slide presented at 21:35 about the input of each head. Actually, each head receives the same output from the previous embedding and positional layer.
Awesome analogies for really understanding what is happening under the hood. Thanks!
characters concepts causality context keywords phrases tasks themes topic narrative cumulative compounding coherent combinatorial continuity monosemanticity polysemanticity recombination permutation question asking answering tasks activities routines relational role charactetistics interpreting translating quantify define describe classify catagories characteristics data information knowledge learning understanding symbolic representation of quantities of information density depth level of details syntax semantics lingo dialects interpreting translating accurately effectively bullets of keywords concpets their relational role within an enviroment with objects entities events sequence of events with periodic cyclical recurrent loops with variation on a theme and recombination values vectors features activation polysamantic superposition architecture scale of focus scale of summaries domain of focus coherently compatable combination dictionary learning values vectors index coordinates concepts connection theme narrative
This is some really interesting work. Love to see people peel back the black box.
Hey this presentation is really cool and I want to understand more of it. My problem is that I have no Idea where those things get taught. I study in my masters degree. What is the best way to teach something like that to myself?
read papers run experiments
what about feedback transformers?
Talks about parity in transformer encoders, waste of time.
Great talk!
This is a really great presentation. I love the data visualizations as I am a visual thinker.
'prude score' = 1/'toxicity score'
wow I had the exact same view about transformers, I even explained it in the comment section of one of yannic kilchers videos. it's really exciting to see other people also came to the same conclusion!
this is the comment: I always thought of MLP modules in Transformers as soft key-value memories, where the keys are learned/memorized patterns (contexts, questions), and the values are memorized predictions (groundtruths, answers) that correspond to each learned pattern, assuming we ignore residual connections. If we have to consider residual connections, then the values are probably the updates/corrections to the predictions of the previous layers. so in my intuitive understanding, Transformers are doing the following steps (vaguely): 1. highlighting specific features of the embeddings (by QKV projections) 2. finding & highlighting temporal patterns (by Q @ K.T) 3. representing the highlighted patterns (by AttentionMap @ V) 4. searching for keys (learned patterns) from key-value memory, that are similar to the pattern representations from 3. (by dot product with FFN1 + ReLU) 5. updating predictions using the retrieved values from the key-value memory (by dot product with FFN2 + residual connection) because residual connections exist, patterns and predictions (or input and output) will become inseparable, making it difficult to precisely describe what's happening in each stage.
this was an excellent presentation! thank you!
This was a great talk. Thanks to Mor Geva and all who helped get this valuable presentation onto youtube.
So, it's easy to remove toxic outputs quickly by destroying the prediction process by weighting something to replace it, but it has a price in reasoning ability. The question that exists in the minds of rational humans: who decides what is "toxic" and what that is? This process can be readily used to align the LLM to produce a particular morality and political viewpoint or the lack thereof, greatly reducing the real and perceived value of the output from them, all in the name of alignment. In a Microsoft video "A Spark of AGI" (part of the Title) they ran the Unicorn benchmark on GPT-4 before and after alignment, and it fared worse post-alignment in generating a viable unicorn. Clearly, brainwashing an LLM in the name of detoxifying the output has a price. Have other options been considered towards handling such things that result from the training data that don't weaken the language processing and therefore reasoning ability, such as tagging such output so you get it if you desire?
Sebastien Bubeck's talk was from MIT and published to TH-cam by himself not Microsoft. I think you might be making an unjustified leap assuming what was done to GPT-4 and that it applies generally. It would seem to me that what Bubeck describes as having been lost was trained out of the model as it was able to use such to create very offensive utterances potentially, like creating vectors that might say spell out hatespeech if asked. It seems like Bing's public beta still had shades of this, with users finding and for example posting on Reddit various ways they had found to trick the model into say swearing at a user. If that were the case the case, than it is perhaps better thought of as a business decision rather than something fundamental to how these models can be fine tuned / trained.
I had exactly the same thought. After describing this fascinating pure research, she then proceeded to use it to pervert the LLM in the way you so articulately described. My thought was that only the final answer should be cleaned up, if that is deemed necessary, in a separate step, like maybe with a small language network specialized in such a task, and only for those who request a sanitized answer.
This is great
I'll bet this reply will not be read, but... isn't the "subject" = "I" and the "object" = "dog" ?
Yes, that's correct. The terminology is confusing though (IF one knows Latin): the 'subject' literally is 'that which is (thrown) UNDER' while the 'object' is 'that which is (thrown) on top' . Everyday sensibilities thus would expect that the object is the one who does sth. and the subject the one which has sth. done TO it. The standard convention is the OPPOSITE however.
@@LGcommaI object generally refers to inert things and the 'subject' is used as English word for persons (King asked his subjects to pay more tax during the drought years...). This could be the reason for English grammar using subject for the actor and object for the acted upon (victim).
fantastic presentation!
Very good. Takeaway as related ideas -- Principle of Maximum Entropy gives Zipf's law scaling w/ density of states set by uncertainty of statistical moments such as mean & variance; idea of parsimony=Occam's; study Gell-Mann's complexity arguments; non-linear functions such as sinusoidal contain much fitting power as they are simply described but have a massive Taylor series expansion -- like layers of NN.
Could I please have the slides? They’re partially obscured by the listeners here. I’d like to use them for a reading group.
hey, not managing to respond from my own account so positing from here - the slides are on my website, which is hosted on github - gailweiss dot github dot io
please provide experiments with datasets
This is such a wonderful series for someone like me with home-bound IBS. I can't go many places or even use Zoom but I feel like I'm right there with you all. God Bless!
Is the last point about Transformers being able to following instructions written ad constant-depth threshold circuits in a paper yet? I didn't see it in the main paper shared, but maybe I'm looking at the wrong one.
That construction is not discussed in the published paper, but we will be releasing an arxiv draft with it soon! I will post a link once it exists :)
@@vikingarnirw Thank you! Very cool work.
"Tree adjoining" seems relevant to philosophy of mind too (as an actual cognitive process that plays a part in generating higher-order thoughts)