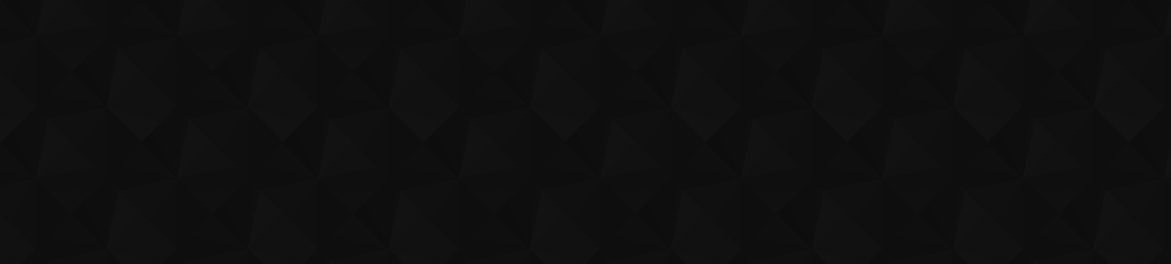
- 66
- 56 444
IARAI Research
Austria
เข้าร่วมเมื่อ 23 ม.ค. 2020
The Institute advances basic and applied research in Artificial Intelligence
We are building a unique environment of world class researchers and industrial-scale real-world data openly shared with the scientific community. We take a proudly interdisciplinary approach to bring together top talent from complementary fields, combining in-depth domain knowledge in AI, simulation, and geospatial analysis from academia and industry. All our research is open and published.
Contact us to contribute and join us.
We organize and sponsor scientific visits, research exchanges, multi-month thematic focus programmes, and academic competitions such as Traffic4cast at NeurIPS
Institute of Advanced Research in Artificial Intelligence
www.iarai.org
We are building a unique environment of world class researchers and industrial-scale real-world data openly shared with the scientific community. We take a proudly interdisciplinary approach to bring together top talent from complementary fields, combining in-depth domain knowledge in AI, simulation, and geospatial analysis from academia and industry. All our research is open and published.
Contact us to contribute and join us.
We organize and sponsor scientific visits, research exchanges, multi-month thematic focus programmes, and academic competitions such as Traffic4cast at NeurIPS
Institute of Advanced Research in Artificial Intelligence
www.iarai.org
NeurIPS 2022 - Weather4cast Special Session
1st prize CORE: FIT-CTU
Petr Šimánek, Jirí Pihrt, Rudolf Raevskiy, Matej Choma
WeatherFusionNet: Predicting Precipitation from Satellite Data
arxiv.org/pdf/2211.16824.pdf
2nd prize CORE: meteoai
Haiyu Dong, Yang Li, Zuliang Fang, Jonathan Weyn, Pete Luferenko
Super-resolution Probabilistic Rain Prediction from Satellite Data Using 3D U-Nets and EarthFormers
zenodo.org/record/7405710
3rd prize CORE: TEAM-NAME
Brian Pulfer, Yury Belousov, Sergey PolezhaevSolving the Weather4cast Challenge via Visual
Transformers for 3D Images
arxiv.org/abs/2212.02456
3rd prize CORE: SI Analytics
Minseok Seo, Doyi Kim, Seungheon Shin, Eunbin Kim, Sewoong Ahn, Yeji Choi
Simple Baseline for Weather Forecasting Using Spatiotemporal Context Aggregation Network
arxiv.org/abs/2212.02952
Transfer Learning: SI Analytics
Doyi Kim, Minseok Seo, Seungheon Shin, Eunbin Kim, Sewoong Ahn, Yeji ChoiDomain
Generalization Strategy to Train Classifiers Robust to Spatial-Temporal Shift
arxiv.org/abs/2212.02968
____
KAIST-CILAB
Jinyoung Park, Minseok Son, Seungju Cho, Inyoung Lee, Changick Kim
RainUNet for Super-Resolution Rain Movie Prediction under Spatio-temporal Shifts
arxiv.org/abs/2212.04005
KAIST_AI
Taehyeon Kim, Shinhwan Kang, Hyeonjeong Shin, Deukryeol Yoon, Seongha Eom, Kijung Shin, Se-Young Yun
Conditioned Orthogonal 3D U-Net for Weather4Cast Competition
arxiv.org/abs/2212.02059
Learn more about the Weather4cast Competitions:
www.iarai.ac.at/weather4cast
___________________________________________________________________
IARAI | Institute of Advanced Research in Artificial Intelligence
www.iarai.ac.at
Petr Šimánek, Jirí Pihrt, Rudolf Raevskiy, Matej Choma
WeatherFusionNet: Predicting Precipitation from Satellite Data
arxiv.org/pdf/2211.16824.pdf
2nd prize CORE: meteoai
Haiyu Dong, Yang Li, Zuliang Fang, Jonathan Weyn, Pete Luferenko
Super-resolution Probabilistic Rain Prediction from Satellite Data Using 3D U-Nets and EarthFormers
zenodo.org/record/7405710
3rd prize CORE: TEAM-NAME
Brian Pulfer, Yury Belousov, Sergey PolezhaevSolving the Weather4cast Challenge via Visual
Transformers for 3D Images
arxiv.org/abs/2212.02456
3rd prize CORE: SI Analytics
Minseok Seo, Doyi Kim, Seungheon Shin, Eunbin Kim, Sewoong Ahn, Yeji Choi
Simple Baseline for Weather Forecasting Using Spatiotemporal Context Aggregation Network
arxiv.org/abs/2212.02952
Transfer Learning: SI Analytics
Doyi Kim, Minseok Seo, Seungheon Shin, Eunbin Kim, Sewoong Ahn, Yeji ChoiDomain
Generalization Strategy to Train Classifiers Robust to Spatial-Temporal Shift
arxiv.org/abs/2212.02968
____
KAIST-CILAB
Jinyoung Park, Minseok Son, Seungju Cho, Inyoung Lee, Changick Kim
RainUNet for Super-Resolution Rain Movie Prediction under Spatio-temporal Shifts
arxiv.org/abs/2212.04005
KAIST_AI
Taehyeon Kim, Shinhwan Kang, Hyeonjeong Shin, Deukryeol Yoon, Seongha Eom, Kijung Shin, Se-Young Yun
Conditioned Orthogonal 3D U-Net for Weather4Cast Competition
arxiv.org/abs/2212.02059
Learn more about the Weather4cast Competitions:
www.iarai.ac.at/weather4cast
___________________________________________________________________
IARAI | Institute of Advanced Research in Artificial Intelligence
www.iarai.ac.at
มุมมอง: 509
วีดีโอ
CDCEO 22: Session III - Invited talk by Nebojsa Jojic
มุมมอง 2462 ปีที่แล้ว
Session III - Chaired by Vipin Kumar - METER-ML: A Multi-sensor Earth Observation Benchmark for Automated Methane Source Mapping by Bryan Zhu and Nicholas Lui from Stanford University, USA - A Center-masked Convolutional Transformer for Hyperspectral Image Classification by Yifan Wang from Shenzhen University, China - Task-guided Denoising Network for Adversarial Defense of Remote Sensing Scene...
CDCEO22: Session II - Invited talk by Nantheera Anantrasirichai
มุมมอง 1882 ปีที่แล้ว
Session II - Chaired by Nebojsa Jojic - Invited talk | Machine Learning for Monitoring Ground Deformation with InSAR Data by Nantheera Anantrasirichai from University of Bristol, England - Mapping Slums with Deep Learning Feature Extraction by Agatha Mattos from University College Dublin, Ireland - Weak-supervision Based on Label Proportions for Earth Observation Applications from Optical and H...
CDCEO22: Landslide4sense Special Session
มุมมอง 4032 ปีที่แล้ว
Special LandSlide4Sense Session - Chaired by Omid Ghorbanzadeh - LandSlide4Sense Competition Design and Dataset Description by Omid Ghorbanzadeh from Institute of Advanced Research in Artificial Intelligence (IARAI) - On the Generalization of the Semantic Segmentation Model for Landslide Detection (Team Tanmlh) by Qingsong Xu from Technical University of Munich, Germany - SwinLS: Adapting Swin ...
CDCEO 22: Session I - Invited talk by Vipin Kumar
มุมมอง 4302 ปีที่แล้ว
Session I - Chaired by Pedram Ghamisi - Introduction by Pedram Ghamisi from Institute of Advanced Research in Artificial Intelligence (IARAI) - Keynote | Big Data in Climate and Earth Sciences: Challenges and Opportunities for Machine Learning by Vipin Kumar from University of Minnesota, USA - Causal Inference with Bayesian Modeling and Field Tests as an Instrument by Lily Xu from Harvard Unive...
Abstraction and Analogy are the Keys to Robust AI - Melanie Mitchell
มุมมอง 1.5K2 ปีที่แล้ว
In 1955, John McCarthy and colleagues proposed an AI summer research project with the following aim: “An attempt will be made to find how to make machines use language, form abstractions and concepts, solve kinds of problems now reserved for humans, and improve themselves.” More than six decades later, all of these research topics remain open and actively investigated in the AI community. While...
Exploring Qualitative Representations in Natural Language Semantics - Kenneth D. Forbus
มุมมอง 3142 ปีที่แล้ว
People use qualitative representations to reason and learn about the continuous world. This suggests that qualitative representations have a role to play in natural language semantics. This talk will summarize my group's work in this area, including how QP theory constructs manifest in English, type-level versus instance-level qualitative models, analogical Q/A training, and work in progress to...
A Number Sense as an Emergent Property of the Manipulating Brain - Pietro Perona
มุมมอง 2312 ปีที่แล้ว
The ability to understand and manipulate numbers and quantities emerges during childhood, but the mechanism through which humans acquire and develop this ability is still poorly understood. In particular, it is not known whether for a child, or a machine, acquiring such a number sense, as well as other abstract concepts, is possible without supervision from a teacher. This question is explored ...
Learned data augmentation in natural language processing - Kyunghyun Cho
มุมมอง 6532 ปีที่แล้ว
Data augmentation has been found as a key aspect in modern machine learning. Especially in domains and problems in which there is no knowledge of important invariances and equivariances, a data augmentation procedure can be designed to encourage a machine learning model to encode those invariances and equivairances. In the case of natural language processing, it is unfortunately difficult to co...
Protein structure prediction with AlphaFold - Andrew Senior
มุมมอง 3.7K2 ปีที่แล้ว
Proteins are are large complex molecules, essential to practically all life’s processes. What a protein does largely depends on its unique 3D structure. Figuring out what shapes proteins fold into is known as the “protein folding problem”, and has stood as a grand challenge in biology for the past 50 years. The machine learning system AlphaFold has been recognised as a solution to this challeng...
How GNNs and Symmetries can help to solve PDEs - Max Welling
มุมมอง 3.5K2 ปีที่แล้ว
Joint work with Johannes Brandstetter and Daniel Worrall. Deep learning has seen amazing advances over the past years, completely replacing traditional methods in fields such as speech recognition, natural language processing, image and video analysis and so on. A particularly versatile deep architecture that has gained much traction lately is the graph neural network (GNN), of which transforme...
Neural diffusion PDEs, differential geometry, and graph neural networks - Michael Bronstein
มุมมอง 4.2K2 ปีที่แล้ว
In this talk, Michael will make connections between Graph Neural Networks (GNNs) and non-Euclidean diffusion equations. He will show that drawing on methods from the domain of differential geometry, it is possible to provide a principled view on such GNN architectural choices as positional encoding and graph rewiring as well as explain and remedy the phenomena of oversquashing and bottlenecks. ...
Towards General and Robust AI at Scale - Irina Rish
มุมมอง 5032 ปีที่แล้ว
Modern AI systems have achieved impressive results in many specific domains, from image and speech recognition to natural language processing and mastering complex games such as chess and Go. However, they often remain inflexible, fragile and narrow, unable to continually adapt to a wide range of changing environments and novel tasks without “catastrophically forgetting” what they have learned ...
Weather4cast 2021 Special Session - Part 2
มุมมอง 1212 ปีที่แล้ว
This session features an in-depth discussion of the Weather4cast 2021 competition results with the CORE and TRANSFER challenge winners as well as selected participants from the leaderboard. The goal of the competition was a short-term prediction of selected weather products based on meteorological satellites data obtained in collaboration with AEMET/ NWC SAF. The competition presented weather f...
Weather4cast 2021 Special Session - Part 1
มุมมอง 4162 ปีที่แล้ว
Weather4cast 2021 Special Session - Part 1
Science4cast Special Session - 3rd Place: Milad Aghajohari
มุมมอง 1092 ปีที่แล้ว
Science4cast Special Session - 3rd Place: Milad Aghajohari
Science4cast Special Session - Special Prize: João Moutinho
มุมมอง 422 ปีที่แล้ว
Science4cast Special Session - Special Prize: João Moutinho
Science4cast Special Session - Invited speaker: Jacob Foster
มุมมอง 1122 ปีที่แล้ว
Science4cast Special Session - Invited speaker: Jacob Foster
Science4cast Special Session - Special Prize: Nima Sanjabi
มุมมอง 822 ปีที่แล้ว
Science4cast Special Session - Special Prize: Nima Sanjabi
Science4cast Special Session - Special Prize: Francisco Andrades
มุมมอง 972 ปีที่แล้ว
Science4cast Special Session - Special Prize: Francisco Andrades
Science4cast Special Session - 2nd Place: Ngoc Tran
มุมมอง 522 ปีที่แล้ว
Science4cast Special Session - 2nd Place: Ngoc Tran
Science4cast Special Session - Special Prize: Harlin Lee and Rishi Sonthalia
มุมมอง 1042 ปีที่แล้ว
Science4cast Special Session - Special Prize: Harlin Lee and Rishi Sonthalia
Science4cast Special Session - Invited Speaker: Rose Yu
มุมมอง 812 ปีที่แล้ว
Science4cast Special Session - Invited Speaker: Rose Yu
Science4cast Special Session - Special Prize: Francisco Valente (Team mondegoscroc)
มุมมอง 732 ปีที่แล้ว
Science4cast Special Session - Special Prize: Francisco Valente (Team mondegoscroc)
Science4cast Special Session - 1st Place: Yichao Lu
มุมมอง 2272 ปีที่แล้ว
Science4cast Special Session - 1st Place: Yichao Lu
Science4cast Special Session - Intro: Mario Krenn
มุมมอง 2912 ปีที่แล้ว
Science4cast Special Session - Intro: Mario Krenn
Traffic4cast 2021 Special Session Part 2
มุมมอง 772 ปีที่แล้ว
Traffic4cast 2021 Special Session Part 2
Traffic4cast 2021 Special Session - Part 1
มุมมอง 1802 ปีที่แล้ว
Traffic4cast 2021 Special Session - Part 1
Neural Implicit Representations for 3D Vision - Prof. Andreas Geiger
มุมมอง 4.2K3 ปีที่แล้ว
Neural Implicit Representations for 3D Vision - Prof. Andreas Geiger
Practical Theory and Neural Network Models - Prof. Michael W. Mahoney
มุมมอง 5653 ปีที่แล้ว
Practical Theory and Neural Network Models - Prof. Michael W. Mahoney
So, two years on ... how's it all working out?
Good discussion.
This is more about simulating large associative networks and their dynamics, it has nothing to do with the brain.
Interesting, where can one find open implementations?
@31:19 "Reverse direction of a string" would also be a good answer.
Great talk!
If tic-tac-toe has around 4500 positions, along with a value for each position (d = 10), does this mean we can store all (positions, value) pairs in 100 (d^2) floats (i.e. 400 bytes) and retrieve them with exponential hopfield network? (exp 10) => 22026.465 ... as for chess, with some funny usage of equivalence classes, store the values of all legal positions? (exp 66) 4.6071865e28 in (expt 66 2) => 4356 floats (i.e. 17424 bytes)? If that is true, chess is as good as solved.
You have to have all the patterns stored somewhere in order to retrieve them. This is nothing like a Hopfield network that stores the images inside the weights. This idea of exponential storage only applies to the mask that is created that is multiplied by ALL the stored images to get the result. The stored images do not have the exponential quality. This is very misleading. Someone explain why I am wrong. See the graph in 48.02 to see what I am talking about. All the images are stored already as separate files/vectors.
Exactly...
@@chibrax54I wish they had provided a Python program example. The only GitHub I’ve seen is a full PyTorch pipeline with the Hopfield already embedded.
I watched this seminar and it was very useful for me. Thanks.
Extremely interesting. This shed a lot of light on the PointConv-related papers. Thank you for sharing!
Glad you enjoyed it!
Thank you this is very clear and informative! I do care about neurobiology so I appreciate the attention to biological plausibility.
You're very welcome!
Thanks a lot for this video. For me, the highlights were the explainability aspects and the connection between size-dependent in-context learning capabilities (from the GPT-3 paper) and Modern Hopfield Networks (th-cam.com/video/k3YmWrK6wxo/w-d-xo.html).
Is there a tensorflow implementation of your Hopfield layer? It would be awesome to do some experiments with it and read the code to understand the details.
At th-cam.com/video/bsdPZJKOlQs/w-d-xo.html, I do not understand the significance of the connection between the Continuous Modern Hopfield update and the attention mechanism: yes, on a very abstract level, both use scaled softmax of a matrix multiplication. But the transformers derive queries, keys, and values from the data vector by learned linear transformations, whereas the Hopfield update does not specify where the queries and keys come from, and always uses the keys as values. So at th-cam.com/video/bsdPZJKOlQs/w-d-xo.html, point 1 (transformer attention), the transformer queries are in fact Y * W_Q for self-attention, so you could leave out R as input, leading to the same layer architecture as point 2 (Hopfield Pooling). Thus I find it confusing to make such strong connections (Hopfield update equals attention) on such an abstract level. For instance, if you allow arbitrary learned or fixed arguments in your Hopfield layer, shouldn't you allow the same flexibility for the attention in a transformer block, and thus the attention in a transformer block could just as well perform pooling or k-nearest neighbor!?
Thanks a lot for the talk, that clarifies a lot from your paper. About the visualizations at th-cam.com/video/bsdPZJKOlQs/w-d-xo.html: Shouldn't there be local minima for metastable states that are in the middle of pattern clusters, e.g. for the bottom right picture at least one local minimum somewhere in the middle of the picture (close to the middle of a cluster of patterns)?
Posed differently: for sufficiently higher beta, a cluster should contain a metastable state in the middle of that cluster? The complex update dynamics shown at th-cam.com/video/bsdPZJKOlQs/w-d-xo.html are caused by the projections to 2d? For instance in the third picture on the top, the patterns sucked into the global minimum in the middle are in fact closer to the global minimum than the patterns that stay, i.e. are not sucked in?
I have been looking for someone out there in A. I. who is putting some good consideration into Douglas Hofstadters work. Thank you
Deep Learning looks to be a mile deep and an inch wide. AI needs to find the right balance between exploitation and exploration to make progress, and the directions Mitchell points to not only have the pioneers' endorsement, but also make that very elusive common sense. A tour de force of a presentation!
Hi, thank you so much for the great talk. I was wondering if we could be accessible to the slides. Look so great.
Super interesting content, thanks for uploading. Are they slides available somewhere?
Thank you Prof!
It's becoming clear that with all the brain and consciousness theories out there, the proof will be in the pudding. By this I mean, can any particular theory be used to create a human adult level conscious machine. My bet is on the late Gerald Edelman's Extended Theory of Neuronal Group Selection. The lead group in robotics based on this theory is the Neurorobotics Lab at UC at Irvine. Dr. Edelman distinguished between primary consciousness, which came first in evolution, and that humans share with other conscious animals, and higher order consciousness, which came to only humans with the acquisition of language. A machine with primary consciousness will probably have to come first. What I find special about the TNGS is the Darwin series of automata created at the Neurosciences Institute by Dr. Edelman and his colleagues in the 1990's and 2000's. These machines perform in the real world, not in a restricted simulated world, and display convincing physical behavior indicative of higher psychological functions necessary for consciousness, such as perceptual categorization, memory, and learning. They are based on realistic models of the parts of the biological brain that the theory claims subserve these functions. The extended TNGS allows for the emergence of consciousness based only on further evolutionary development of the brain areas responsible for these functions, in a parsimonious way. No other research I've encountered is anywhere near as convincing. I post because on almost every video and article about the brain and consciousness that I encounter, the attitude seems to be that we still know next to nothing about how the brain and consciousness work; that there's lots of data but no unifying theory. I believe the extended TNGS is that theory. My motivation is to keep that theory in front of the public. And obviously, I consider it the route to a truly conscious machine, primary and higher-order. My advice to people who want to create a conscious machine is to seriously ground themselves in the extended TNGS and the Darwin automata first, and proceed from there, by applying to Jeff Krichmar's lab at UC Irvine, possibly. Dr. Edelman's roadmap to a conscious machine is at arxiv.org/abs/2105.10461
Melanie is asking the questions everyone should be asking. There is a lack of metacognitive abilities in science and the focus on solving metrics without actually making progress has become so prevalent that it's becoming damaging. There are a lot of lessons in the perspective Melanie provides.
Thanks for sharing this wonderful talk!
Thanks for posting this. However, I'm finding it very hard to understand. For example, at 5:52 I hear "if mewk size is equal to oik size". If you don't speak English well, please speak slowly. Also, if you do speak English well, please speak slowly. This information is too complex and subtle for a hurried presentation.
settings/playback speed/0.5
That is probably because you're not very found of greek letters used in hopfield material. He says: new xi is equal old xi. If you do not understand the subject well just go to study simpler subjects instead criticize this amazing material. Or go to read the paper. He is doing a lot of work speaking someone else language, you should be grateful instead just trolling over the internet.
Fantastic talk!
congratulations Francisco!
🙌🙌🙌👏👏👏
14:50 restricted boltzman machines are far better options at this point.
Very interesting
Glad you enjoyed it! Thank you for watching :)
fascinating, gold mine in here. love the Q&A part most
Glad you enjoyed it! Thank you for watching :)
thank you very much! This is very insightful!
Thank you for watching :)
Great! Than you for uploading it online! 💙
Thank you for watching :)
If you look at the variance equation for linear combinations of random variables as it applies to dot products you see that fully distributed inputs are prefered. Also especially for high storage density non-linear behavior is needed. Thus to use the dot product as associative memory first you do a vector to vector random projection, then apply non-linear behavior, then do the dot product. You can use a random fixed pattern of sign flipping followed by the fast Walsh Hadamard transform as a quick distributing random projection.
The sound quality could be a bit better.