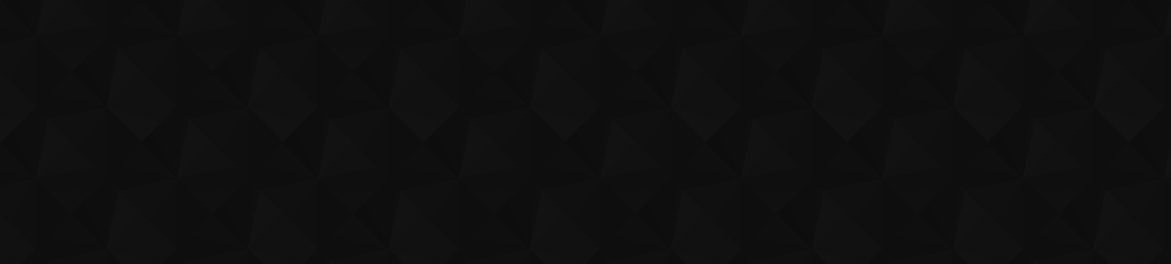
- 59
- 218 625
Computer Science Engineering
เข้าร่วมเมื่อ 22 มี.ค. 2016
This channel will bring you topics on computers, softwares and tech tutorials. Hope this channel will be able to provide you with important piece of information.
If you find this channel helpful, you can also help me by subscribing. It would really help me a lot.
Thank you! :)
If you find this channel helpful, you can also help me by subscribing. It would really help me a lot.
Thank you! :)
5.1.3 Backpropagation Intuition by Andrew Ng
Neural Networks : Learning
Machine Learning - Stanford University | Coursera
by Andrew Ng
Please visit Coursera site:
www.coursera.org
Machine Learning - Stanford University | Coursera
by Andrew Ng
Please visit Coursera site:
www.coursera.org
มุมมอง: 2 370
วีดีโอ
5.1.2 Backpropagation Algorithm by Andrew Ng
มุมมอง 9K5 ปีที่แล้ว
Neural Networks : Learning Machine Learning - Stanford University | Coursera by Andrew Ng Please visit Coursera site: www.coursera.org
5.1.1 Cost Function by Andrew Ng
มุมมอง 1.8K5 ปีที่แล้ว
Neural Networks : Learning Machine Learning - Stanford University | Coursera by Andrew Ng Please visit Coursera site: www.coursera.org
Reactions from HIM IT-BPM Stakeholders and Industry Players: HIMSCon Philippines 2017
มุมมอง 377 ปีที่แล้ว
Reactions from HIM IT-BPM Stakeholders and Industry Players: HIMSCon Philippines 2017 Healthcare embracing change that comes with mobility and intelligence such as IoT, deep learning and other emerging technologies to provide more quality healthcare to people.
4.3.3 Neural Networks Multiclass Classification by Andrew Ng
มุมมอง 1.6K7 ปีที่แล้ว
Neural Networks : Representation Machine Learning - Stanford University | Coursera by Andrew Ng Please visit Coursera site: www.coursera.org Learn Machine Learning for free.
4.3.2 Neural Networks Examples and Intuitions II by Andrew Ng
มุมมอง 1K7 ปีที่แล้ว
Neural Networks : Representation Machine Learning - Stanford University | Coursera by Andrew Ng Please visit Coursera site: www.coursera.org Learn Machine Learning for free.
4.3.1 Neural Networks Examples and Intuitions I by Andrew Ng
มุมมอง 2.2K7 ปีที่แล้ว
Neural Networks : Representation Machine Learning - Stanford University | Coursera by Andrew Ng Please visit Coursera site: www.coursera.org Learn Machine Learning for free.
4.2.2 Model Representation II by Andrew Ng
มุมมอง 9937 ปีที่แล้ว
Neural Networks : Representation Machine Learning - Stanford University | Coursera by Andrew Ng Please visit Coursera site: www.coursera.org Learn Machine Learning for free.
4.2.1 Model Representation I by Andrew Ng
มุมมอง 1.3K7 ปีที่แล้ว
Neural Networks : Representation Machine Learning - Stanford University | Coursera by Andrew Ng Please visit Coursera site: www.coursera.org Learn Machine Learning for free.
4.1.2 Neurons and the Brain by Andrew Ng
มุมมอง 1.2K7 ปีที่แล้ว
Neural Networks : Representation Machine Learning - Stanford University | Coursera by Andrew Ng Please visit Coursera site: www.coursera.org Learn Machine Learning for free.
4.1.1 Non linear Hypotheses by Andrew Ng
มุมมอง 1.3K7 ปีที่แล้ว
Neural Networks : Representation Machine Learning - Stanford University | Coursera by Andrew Ng Please visit Coursera site: www.coursera.org Learn Machine Learning for free.
3.4.4 Regularized Logistic Regression by Andrew Ng
มุมมอง 2K7 ปีที่แล้ว
Solving the Problem of Overfitting Machine Learning - Stanford University | Coursera by Andrew Ng Please visit Coursera site: www.coursera.org Learn Machine Learning for free.
3.4.3 Regularized Linear Regression by Andrew Ng
มุมมอง 2.3K7 ปีที่แล้ว
Solving the Problem of Overfitting Machine Learning - Stanford University | Coursera by Andrew Ng Please visit Coursera site: www.coursera.org Learn Machine Learning for free.
3.4.2 Cost Function (Regularization) by Andrew Ng
มุมมอง 2.4K7 ปีที่แล้ว
Solving the Problem of Overfitting Machine Learning - Stanford University | Coursera by Andrew Ng Please visit Coursera site: www.coursera.org Learn Machine Learning for free.
3.4.1 The Problem of Overfitting by Andrew Ng
มุมมอง 8K7 ปีที่แล้ว
Solving the Problem of Overfitting Machine Learning - Stanford University | Coursera by Andrew Ng Please visit Coursera site: www.coursera.org Learn Machine Learning for free.
3.3.1 Multiclass Classification One vs all by Andrew Ng
มุมมอง 53K7 ปีที่แล้ว
3.3.1 Multiclass Classification One vs all by Andrew Ng
3.2.3 Advanced Optimization by Andrew Ng
มุมมอง 2.1K7 ปีที่แล้ว
3.2.3 Advanced Optimization by Andrew Ng
3.2.2 Simplified Cost Function and Gradient Descent by Andrew Ng
มุมมอง 2.6K7 ปีที่แล้ว
3.2.2 Simplified Cost Function and Gradient Descent by Andrew Ng
3.1.2 Hypothesis Representation by Andrew Ng
มุมมอง 2.6K7 ปีที่แล้ว
3.1.2 Hypothesis Representation by Andrew Ng
2.2.2 Normal Equation Noninvertability by Andrew Ng
มุมมอง 1.1K7 ปีที่แล้ว
2.2.2 Normal Equation Noninvertability by Andrew Ng
2.1.5 Features and Polynomial Regression by Andrew Ng
มุมมอง 4.6K7 ปีที่แล้ว
2.1.5 Features and Polynomial Regression by Andrew Ng
2.1.4 Gradient Descent in Practice II Learning Rate by Andrew Ng
มุมมอง 8K7 ปีที่แล้ว
2.1.4 Gradient Descent in Practice II Learning Rate by Andrew Ng
2.1.3 Gradient Descent in Practice I Feature Scaling by Andrew Ng
มุมมอง 5K7 ปีที่แล้ว
2.1.3 Gradient Descent in Practice I Feature Scaling by Andrew Ng
2.1.2 Gradient Descent for Multiple Variables by Andrew Ng
มุมมอง 7K7 ปีที่แล้ว
2.1.2 Gradient Descent for Multiple Variables by Andrew Ng
Its helped me - thanks
Octave is even more higher level and mathematically oriented than python meaning such ideas can be expressed much more concisely it's a bit like a free Matlab or Wolfram
This is implementation of Linear regression from scratch in NumPy only. In-depth explanation of key concepts like Cost Function and Gradient Descent th-cam.com/video/wxCQxZKo4hU/w-d-xo.html
That's a great.❤
I cannot follow the accent unfortunately and even the CC is not working
why you shorten your last name? I want cite you!
This is very good! But where did you get Andrew's presentation?
First comment enjoy
I wonder how the complexity of the model might affect the overfitting (or underfitting?)
the higher order/degree of the model equation is, higher the chances of overfitting..
Where can I watch this old course? Thanks
first
ㅤ
Great video!
Thank you so much. It’s been very useful. 🙏👏
Hello, Do you know if I could listen to the sound of the MANIAC somewhere on the internet ? I'm a sound editor working on an audio documentary about Mathematics and litterature, and I need to recreate the sound of the MANIAC. Thanks for your answer. Helena
The great video, thank you so much professor
so nicely explained. thank you!
instead of using superscript and subscript terms , had it been explained like start with the gist of what this algorithm does and then using math plus superscript , would help holding the audience and also motivating the audience to continue watching
Nicely done it sir...what about one vs one
I did not get one thing...Suppose for a classification we get the max probability..then we wd be classifying only one class separately and rest 2 as another...but how are we classifying all 3 separately??
well done Andrew
Well explained but Why is it called cost function? And taking 1/2 is not clear. Why and why not take square root?
Cost function is also called as Loss function, both are synonyms. Division by m or 2m is interchangeable. What we are really concerned about it a model which is producing least error , not the value of the loss function directly. Cost functions can be of 3-types , among them is a regression, which again has 3 types , that is Mean Error , Mean squared error , mean absolute error . Why so many ? Cause a data set may have negative/positive errors , taking mean directly may cancel out +/- errors , and taking a square directly can be a bit troublesome if you have some outliers . In these videos , Andrew can be seen using all three in regression based . Note :it's not the only required param for concluding a model isn't good.
A quadratic polynomial is much easier to work with than a square root, and as it will have a minimum at the same point, we can work with the quadratic polynomial. The 1/2 is there so that the derivative of the cost function will be weighed with 1.
Everybody in this ML field directs to use python, you are the first one who referred to octave. Why is this so?
I know right
Any video for multiclass entropy and entropy. Please show calculations sample. Thanks.
Don't you have legal issues for copying content from coursera
I am pretty sure coursera copied from his content.
he is coursera
@@GelsYT hahaha true..
Thanks Andrew Yang, I'll definitely vote for you.
wrong dude, the other guy wants to give you UBI. This guy wants to give you OVA
audio quality is shit
Thank you for great explanation Sir!
thankyou sir
Just wanted to say these videos are amazing! thank you!
There is an error of the example Andrew used here to demonstrate Normal equation. The X is the 4 by 5 matrix which makes the system underdetermined, which also result in the inverse of X's transpose multiplying X having no inverse. So the Normal equation cannot be calculable.
X is 4x5 and X(transpose) is 5x4. Therefore, X(transpose)*X = 5x4 * 4x5 which results in a 5x5 matrix, which has an inverse.
@@bonipomei Very late reply but being a square matrix doesn't mean it has inverse.
@@brownishcookie yes especially if its determinant is 0
Great video, can you make a video on Stemming with Multiclass Classification?
Get this man a good Camera and Mic.
Sending you the bill...
@@namangupta8609 Did you receive the bill? Or you’ll be the only youtuber watching this video
Great video
What does theta represent?
learning rate
@@ofathy1981 alpha is the learning rate in gradient descent .... theta is a parameter like weights in NN
@@ofathy1981 Theta does not represent the learning rate, instead it represents the parameters of the model (e.g. the weights). So P(y | x; θ) translates to English as "The probability of *y* given *x* , parameterized by *θ* ".
That the parameters of logistic classifier which is trained separately for each case