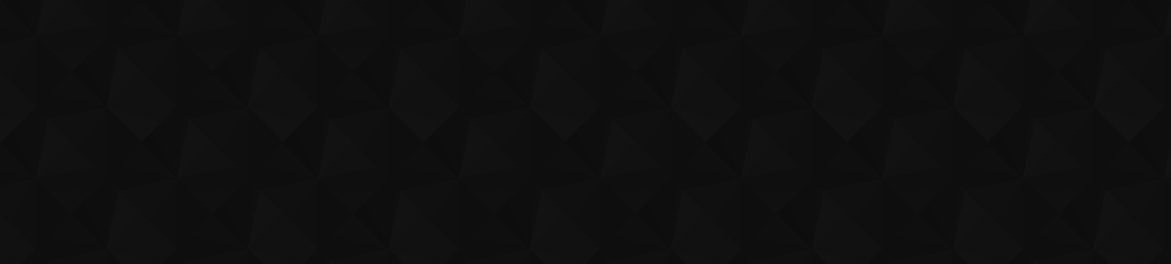
- 460
- 195 145
Toronto Machine Learning Series (TMLS)
เข้าร่วมเมื่อ 22 ธ.ค. 2017
Tina White - Decentralized exposure alert protocols for protecting communities from COVID-19
A discussion of the novel Temporary Contact Number (TCN) protocol and the history of its development within an independent research collaboration between Stanford and Waterloo for stopping the spread of COVID-19. Different app-based methods for assessing proximity are discussed along with their privacy trade-offs and implications. Comparisons are made between the privacy models of apps that use Bluetooth to send decentralized exposure alerts and those that perform centralized digital contact tracing.
มุมมอง: 124
วีดีโอ
Dr. Benjamin Fine & Liam McCoy - HowsMyFlattening: Ontario's COVID-19 Data Analytics Collaborative
มุมมอง 6311 หลายเดือนก่อน
Learn about a growing collaborative of clinicians, epidemiologists, data scientists, engineers and data viz experts from academia and industry trying to make sense of COVID-19 data to help guide Ontario's curve flattening activities.
Kenny Daniel - DevOps for ML and other Half-Truths: Processes and Tools for the ML Lifecycle
มุมมอง 42711 หลายเดือนก่อน
- Speaker Bio - Kenny Daniel is a founder and CTO of Algorithmia. He came up with the idea for Algorithmia while working on his PhD and seeing the plethora of algorithms that never saw the light of day. In response, he built the Algorithmia Cloud AI Layer, which has helped more than 80,000 developers share, pipeline, and consume more than 7000 models. Through his work with hundreds of companies...
Sheldon Fernandez - COVID-Net for COVID detection and risk stratification via chest radiography
มุมมอง 4211 หลายเดือนก่อน
COVID-Net is a neural network for COVID detection and risk stratification via chest radiography. In addition to illustrating its operational benefits - risk stratification and improved diagnostic sensitivity - this presentation will provide of an overview of its rapid construction by means of our explainability technology.
Stephen Mackinnon - PolypharmDB, Quickly Identifies Repurposed Drug Candidates for COVID-19
มุมมอง 4911 หลายเดือนก่อน
"There is an immediate need to discover treatments for COVID-19, the pandemic caused by the SARS-CoV-2 virus. Standard small molecule drug discovery workflows that start with library screens are an impractical path forward given the timelines to discover, develop, and test clinically. To accelerate the time to patient testing, here we explored the therapeutic potential of small molecule drugs t...
Machine Learning Simplified from Ideation to Deployment in Minutes with Automated Machine
มุมมอง 13111 หลายเดือนก่อน
Speaker: Aniththa Umamahesan - Program Manager, Microsoft Key Learnings: Artificial intelligence (AI) has become the hottest topic in tech. Executives, analysts, engineers, and developers all want to leverage the power of AI to gain better insights and make better predictions. But machine learning requires advanced data science skills that are hard to come by. Automated ML is an emerging field ...
Sabina Stanescu - Your First ML Model In Production: Examples & Considerations
มุมมอง 18211 หลายเดือนก่อน
As Data Science professionals, we want to do innovative, impactful work. Thus, our work on data munging and building machine learning models cannot happen in isolation from business objectives and the infrastructure of our organizations. In this talk, I will explore ways to identify impactful, executable Data Science work, and how to take this work to production. I will discuss what it means to...
Parnian Afshar - Capsule Network-based Framework for Identification of COVID cases from X-ray Images
มุมมอง 7011 หลายเดือนก่อน
We present a modeling framework based on Capsule Networks, referred to as the COVID-CAPS, being capable of handling small datasets, which is of significant importance due to the sudden and rapid emergence of COVID-19. Our results based on a dataset of X-ray images show that COVID-CAPS has an advantage over previous CNN-based models.
Kaushik Roy - Are Your Models Location Smart?
มุมมอง 21711 หลายเดือนก่อน
- Speaker Bio - Kaushik Roy is a Location Insights Specialist at Municipal Property Assessment Corporation. - Talk Abstract - What was the promised land? A location. What do we protect with all our might? Our Borders? The Royal Bank of Canada considers any account login from Russia a red flag. Starbucks considers hundreds of locations before deciding on one. The use of AI techniques in urban an...
Vinnie Saini - RPA and AI disrupting the Financial Industry
มุมมอง 23311 หลายเดือนก่อน
The talk will focus on how implementing Robotic Process Automation(RPA) in the sector of finance is optimizing the manual operational processes, while offering effective protection against financial cyber threats. We'll then talk about how RPA is also beneficial for data management and is disrupting financial services with infused Artificial Intelligence(AI).
Dmitry Baev - Deploying Distributed AI and Machine Learning in Financial Services
มุมมอง 22911 หลายเดือนก่อน
Financial Services industry has the potential to benefit from advanced application of Machine Learning and Artificial Intelligence by leveraging their vast data reserves in order to transform their businesses. However, keeping pace with new technologies for data science and Machine Learning can be overwhelming. Financial Services industry regulations can make it even more challenging to deploy ...
Dr. Neeraj Kashyap - Machine Learning
มุมมอง 17311 หลายเดือนก่อน
In industries like finance and healthcare, access to data is restricted due to privacy and security concerns. The author will cover how to use open source machine learning tools and techniques to make use of sensitive data without compromising privacy limitations.
Denise Gosnell -Modeling, Querying and Seeing Time Series Data within a Self-Organizing Mesh Network
มุมมอง 14511 หลายเดือนก่อน
Denise Gosnell -Modeling, Querying and Seeing Time Series Data within a Self-Organizing Mesh Network
Dean Wampler - Ray and how it enables easier DevOps
มุมมอง 7311 หลายเดือนก่อน
Dean Wampler - Ray and how it enables easier DevOps
Saranyan - MLOps that works: How we built ML pipelines for deploying models for autonomous factories
มุมมอง 12111 หลายเดือนก่อน
Saranyan - MLOps that works: How we built ML pipelines for deploying models for autonomous factories
LTC Isaac J Faber Ph D - Building an AI Capability in the United States Army
มุมมอง 6811 หลายเดือนก่อน
LTC Isaac J Faber Ph D - Building an AI Capability in the United States Army
Yaron Haviv - Simplify ML Pipeline Automation and Tracking using Kubeflow and Serverless Functions
มุมมอง 9611 หลายเดือนก่อน
Yaron Haviv - Simplify ML Pipeline Automation and Tracking using Kubeflow and Serverless Functions
Ebrahim & Jisheng - Automated Pipeline for Large-Scale Neural Network Training and Inference
มุมมอง 11611 หลายเดือนก่อน
Ebrahim & Jisheng - Automated Pipeline for Large-Scale Neural Network Training and Inference
Ayesha Hafeez - Walkthrough: Utterance Generator Fast Tracks Chatbot Training
มุมมอง 3211 หลายเดือนก่อน
Ayesha Hafeez - Walkthrough: Utterance Generator Fast Tracks Chatbot Training
Abe Gong - Fighting Pipeline Debt With Great Expectations
มุมมอง 7611 หลายเดือนก่อน
Abe Gong - Fighting Pipeline Debt With Great Expectations
Josh Poduska - Workshop: Turbo-Charging Data Science with AutoML
มุมมอง 7011 หลายเดือนก่อน
Josh Poduska - Workshop: Turbo-Charging Data Science with AutoML
Innodata Presents: Bogged Down by Annotation Why SMEs Should Do the Heavy Lifting
มุมมอง 62211 หลายเดือนก่อน
Innodata Presents: Bogged Down by Annotation Why SMEs Should Do the Heavy Lifting
Lina Palianytsia - Metrics: Holistic Health Metrics of ML-Based Product
มุมมอง 11511 หลายเดือนก่อน
Lina Palianytsia - Metrics: Holistic Health Metrics of ML-Based Product
Josh Mineroff - Workshop: Intro to ML with Python
มุมมอง 7411 หลายเดือนก่อน
Josh Mineroff - Workshop: Intro to ML with Python
Bahareh, Eric and Safura - Workshop: Trusted AI: a workshop on IBM Watson OpenScale
มุมมอง 4811 หลายเดือนก่อน
Bahareh, Eric and Safura - Workshop: Trusted AI: a workshop on IBM Watson OpenScale
Don Ward - Edge AI - The Next Frontier
มุมมอง 5411 หลายเดือนก่อน
Don Ward - Edge AI - The Next Frontier
At 1:17:00 If your values are continuous you can keep a threshold for eg: thres = 2 5 - 4 < thres is considered close whereas 5 - 2 > thres it's not close. Now, you have a binary matrix for precision and recall
matrix sparsity of MovieLens 20M Dataset using your code is 0.54% where my findings imply the matrix sparsity is 100-0.54=99.46% which makes more sense after all. I dont understand. isnt the formula sparsity=(#zero valued elements)/(#total elements) ?
02.07.2024: Semantic Kernel : 20k stars, 1.3M downloads, 268 contributors Langchain : 88k stars, 81M downloads, 477 contributors
@ternaus I love your hair looks good on you!🎉 Congratulations on this one
Had a meeting with them last week. As of now, bb ai will be released next year but don’t quiote me on this
Thanks great overview!
Completely usless model. Models learn from the past data . They cannot predict the future. Once political event can completely turn the model parameters upside down.
not a denmo what a garbage
The mic. It's so painful to follow. Please, next time you record a conference fix this :)
Hi, thank you for your presentation. Could you please give me the source and dataset for this model?
it seems great but in practice this only works for very small subset of false predictions, which is not worth it in a critical usecase or non critical usecase
thanx.
33:30 That would actually just be a better test to see if a human is a robot.
Can we get the notebooks?
thank you so much bro
17:00 Calculating the average rating
Which white paper is referred in this video? Can i get link?
Dear Jil, Your tutorial is extremely helpful and well presented - well done! However, I do have a small question about the section where You discuss sparsity. In most documentation I see that sparsity is measured by getting the quotient of number of zero or missing values / total number of elements in the matrix. However, You're taking the number of ratings (rather than missing values) into the formula - why? Considering that we have 610x9724=5,931,640 elements and 100,836 ratings - it would seem that our sparsity should be quite big (as most data is missing)! Also, could You point to any documentation which discussed at which levels of sparsity the CF methods should be used? Best regards, Pawel.
You're right, I found 5830804 empty elements out of 5931640 which means the sparsity is 98.30% not 1.7%
tks for sharing
Subpar model 😂. I legit made something better...by myself with $500. I guess everything is so new, it seemd few actually understand how to build a SOTA model. Guess this was a POC though.
Bard got trained on hundreds of trillions of tokens vs 700 billion tokens for Bloom model.
Thank you for sharing your experience which we don't hear from Google and OpenAI
Okay, I nominate this guy to be future Marty McFly in "Back to the future 4"
great talk! lovin' the technical details about training
Very educational, I am only as non-technical novice, especially with Gen AI and Foundation Models. I learned from 40 minutes.
Has it been 10 weeks already?
sorry, don't think that slide on 6:49 was explained well.
I think you should have started from a checkpoint & trained on your private dataset, just monitoring test performance on MMLU and your finance metrics.
This man is a genius
look into
FinGPT is better tho.
방송도 버출얼 캐릭터들이 많이해. 물론 사람이 하는건데, 이것도 AI화 될수있어. 그럼 그것도 인간이 할수 없을꺼야.
모든 직업이 그렇게 되는건 아니지. 스포츠는 인간이 하겠지. 축구 야구 농구 배구, 그리고 방송도 인간이 하고 콘서트 노래도 인간이 하겠지. 사이버 가수 AI가수가 나오긴하는데, 완전히 대처될진 모르겠고, 영화나 드라마도 애니메이션이 흥행하는것을보면, 사이버 가상의 배우들이 영화와 드라마를 찍을날도 올꺼야. 그때는 배우가 단 1명도 없는데, 영화와 드라마가 나올꺼야. 애니메이션인데 실사화 된 애니메이션같은거지. 배우라는 직업도 없어질수있어. 왜냐면 더 싸더니까,
아나운서도 필요가 없어. 이미 AI 아나운서와 기자가 뉴스 진행을해. MBN 김주희 아나운서였나? 중국의 CCTV도 10년전에 한거야. AI 아나운서가해도 똑같아. 별 차이가 없어. 그럼 아나운서도 필요가 없어. 기자도 다른 기사 복불복하는 기사 청와대에서 보도 할꺼 내려줘서 그거 그냥 쓰는 기자들도 필요가 없어. 그거 AI가 하면 돼. 기자도 필요가 없어. 상담사들도 챗gpt가 다 상담하고 대답해. 상담사라는 직업들도 90%는 AI로 바뀌고 나머지 10%만 인간이 할꺼고, 사람이 필요가 없어. 그럼 도대체 어디서 직업을 구할까? 택시 운전도 테슬라와 구글 웨이모가 자율주행 택시 하면 택시 직업 수백 수천만명의 사람들은 직업을 잃어. 트럭 운전사도 없어질꺼고, 어디서도 일을 구할수가 없어. 인간은 필요가 없어. 택배 물류도 기계가 다해. 인간은 필요가 없어.
이미 사람 대신에 로봇을써. CJ대한통훈도, 쿠팡도, 영국의 오카도? 배달도 로봇배달이 시작되고, 운전도, 테슬라와 구글의 웨이모가 자율주행을 하고, 이미 시작됐어. 상담사도 챗 gpt로 인도에서 바꾸고 사람을 해고했잖아. 테슬라 공장은 사람이 없어. 더 없어도 되는데, 고용률 생각하는 정부때문에 유지하는거야. 사실 필요가 없어. 기계가 다할수있어. 그리고 기계로 하면 마진율이 더 높아. 주택 보급도 3d프린터가 사람없이 집을 지을수있어. 그럼 지금 집값 반값으로 공급할수있어. 그럼 부동산 시장은 다 터져. 자본주의는 무너지고, 은행시스템도 무너져, 끝나는거야. 이미 자본주의는 끝났다니까. 그냥 죽은 자본주의를 붙들고 살아가는거야. 지들 죽기 싫다고, 자기들 기득권 놓기 싫다고 그런것뿐이야. 이미 이건 막을수가 없어.
집도 미래에는 무한 공급이 가능해3d프린터가 무한으로 집을 찍어낼수있기때문에, 무조건 가능해. 지금 당장도 가능해. 그런데 자본주의 기득권들이 자기들 부동산 자산 가치가 하락할까봐, 막고있는거지. 근데 아무리 막고 아무리 이걸 반대해도 바꿀수가 없어.
자본주의라는 개념은 미래에는 없어. 그건 이제 과거의 존재했던 개념으로만 남을꺼야.
그러니가 이 미래는 자본주의가 바뀌던지, 인간이 멸종하던지 둘중에 하나야. 미래에는 자본주의라는 개념은 없어져. 이건 왜냐면 인간이 멸종해서 없어지고, 인간이 살아남기 위해서 자본주의를 바꿔도 없어지기때문이야. 이건 확정이야.
이미 이 세상은 돈을 못벌면 사람 취급을 안해. 돈이 없으면 내가 파는 물건 내가 일하는 월급을 줄수가 없는 존재야. 그럼 노숙자와 똑같은거야. 왜냐면 돈을 못버니까? 그러면 돈을 못버는 존재를 어떻게 판단하고 있지? 사람 취급을 안해. 왜냐면 내가 당장 오늘 돈을 벌어서 밥을 먹거나, 잠을 자거나, 스마트폰을 쓰거나, 인터넷할려나, 차를 타거나, 뭘 할려고해도 돈이 있어야해. 그런데 돈을 벌려면, 인간은 어떻게 했지? 노동력을 제공해야해. 돈을 가지고 태어나지 않은 이상은, 그런데 이미 노동력을 AI와 로봇이 우위로 점할거라는것을 모르는 사람이 없어. 그럼 인간이 필요할까? 왜냐면 상대가 안되는데? 능력주의 성과주의 생산성, 기업이 인간과 로봇중에 생산성이 높은 쪽을 고른다고, 당연히 더싸고 일을 잘하는 로봇을 골라. 그래서 로봇이 점점 많아져, 소비자또한 인간보다, 로봇과 AI를 선택해. 자율주행이 운전을하면 더 싸. 그럼 당연히 소비자 인간이 인간대신에, 더 싸게 운전해주는 자율주행을 선택해. 인간이 선택을하는거야. 인간이 스스로 그런 선택을해. 그럼 돈을 못버는 인간은 살아남을수가 없어. 노숙자가 어떻게 살아남아? 그럼 모든 인간이 로봇과 기계 AI에 밀린다고, 그럼 기업이 인간을 고용을하지 않을꺼야. 그럼 지금 자본주의앞에선 인간은 살아남을수가 없어. 자본주의 앞에서 경제적 생산성 능력주의 이 앞에서 인간은 존재의 이유가 없어. 왜냐면 로봇에 상대가 안되거든, 그럼 인간은 멸종이 확정이야. 살아남을수가 없어. 자본주의 논리로는 그렇다는거야. 모든 기업이 어느 나라에서라도, 다 그렇게 해. 다 기계 로봇 AI로 교체중이야. 기업이 그래야 살아남으니까. 자본주의 논리로는 인간은 살아남을수가 없어. 이미 이건 결과로 증명된거야.
지금 모든 회사들이 AI를 다 따로 만들잖아. 그런데 그렇게 할 필요가 없이, 모든 AI 회사 IT 빅테크 기업 국가들이 힘을 합쳐서 엄청난 데이타를 학습하고 대규모 컴퓨터를 합쳐서 한개의 AI를 만들어내면, 아마 성능이 상상을 초월할꺼다. 아마 인간을 이미 아득히 넘을 성능을 보이는 수준일꺼다. 이미 그 수준으로 가지 않을까? 인간의 지식보다 훨씬 더 넓은 지식을 가진거지. 인간은 의사나 판사나 it프로그래머나, 그래픽디자이너나, 소설가나, 어떤 한 가지 직업의 지식분야만을 전문가로써 공부해서 성장하는데, 이 AI는 모든 직업의 전문가 수준의 지식을 다 통합하고 있으니, 지적능력 지식으로는 상대가 안돼. 수천 수만명의 박사 수준의 전문가 지식을 아득히 넘은수준이 바로 지금 나올수있다. 지적능력으로는 상대가 안돼. 프로그래밍능력, 헌법에 나온 법 데이타, 100년동안 매일 나온 전세계의 모든 언론사의 기사들, 모든 존재하는 소설책의 내용들, 지적 능력 데이타로는 상대가 안돼. 인간은 절대 못이겨. 이미 상대가 안돼. 모든 나라와 모든 빅테크와 모든ai기업이 합치면 인간을 아득히 뛰어넘는 수준의 AI는 이미완성됐다. 나눠서 해도 성능이 뛰어나. 합치면 이미 인간을 아득히 뛰어넘는다. 인간은 이미 상대가 안돼. 그럼 인간은 과연 미래에 살아남을수있을까? 직업을 못구해. 경제적 능력으로는 ai에게 상대가 안돼. 인간이 필요할까? 직업을 못구해 인간의 성능을 뛰어넘으면 인간이란 존재할까? 능력주의, 성과주의, 실력으로 따지면 이미 상대가 안돼. 그런 경제논리 능력논리로따지면 인간은 필요가 없어. 왜냐면 상대가 안되니까. 그럼이 미래에 살아남을수있을까? 거의 불가능하지 않을까?
bringing value to shareholders - Yay 😀
Bloomberg GPT is now obsolete
Awesome way to learn
Its funny he mentions that open source helped them and that want to share what they learned.. they used datasets, architecture, likely code from open source. And yet the only novel thing I can even spot in this whole talk is their special tokenizer for numbers, which he “doesn’t want to get into.” How transparent.
He literally says they tokenizes individual numbers and uses multi word tokenizers, as compared to the arbitary number tokenizer of gpt-3.
Good Kid?
24:18 lr reduced from 6e-5 to 1e-4 ? ridiculous
Ah those numbers are transposed - good catch.
I'd definitely liked more examples on what it can do. "Does better on financial tasks" is a very vague statement. But great work. I wish I had their resources so that I can train my own LLM.
Do you have a BBG license? If not, dumb money is always last. GS telling everyone that CRE is still alive means it's betting against CRE. BBG telling everyone they're using LLM means it's too late for John Q. Public.
Markov chain... Shannon?..pff
?
Does BloombergGPT suffer the same problems as GPT4, most notably hallucinations? We talk about mistakes that the financial industry made, and the government had to bail them out. The noteworthy ones I can think of are changes in the tax code resulting in Texas's banks access to commercial real estate coverage in 1984, Long Term Capital Management, the 2008 Financial Crisis due to mortgage default swaps, and Oil Pipeline investment in Mexico. We talk about capitalism, but the level of corruption and bailouts makes the Soviets just a bunch of amateurs. So, hey, if it makes mistakes, the tax payer will bail them out.
If you are suffering from hallucinations using your own money, that is okay. The problem arises when you are hallucinating with other people's money.
How well the model predict future prices?