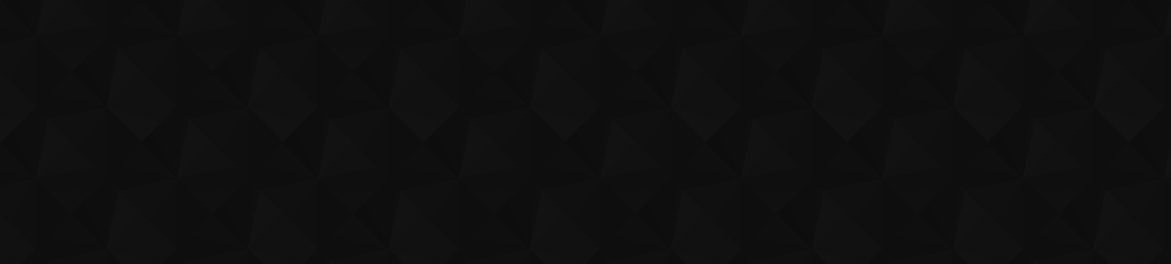
- 4
- 60 337
Kaiwen Wang
เข้าร่วมเมื่อ 3 ต.ค. 2011
Computer Science PhD at Cornell
ex-Facebook Engineer
ex-Facebook Engineer
Conditional Language Policy for Steerable Alignment
📝 "Conditional Language Policy: A General Framework for Steerable Multi-Objective Finetuning", to appear at EMNLP 2024.
X 🔗: x.com/kaiwenw_ai/status/1855304823760970056
Full Paper: arxiv.org/abs/2407.15762
Abstract: Reward-based finetuning is crucial for aligning language policies with intended behaviors (e.g., creativity and safety). A key challenge is to develop steerable language models that trade-off multiple (conflicting) objectives in a flexible and efficient manner. This paper presents Conditional Language Policy (CLP), a general framework for finetuning language models on multiple objectives. Building on techniques from multi-task training and parameter-efficient finetuning, CLP learn steerable models that effectively trade-off conflicting objectives at inference time. Notably, this does not require training or maintaining multiple models to achieve different trade-offs between the objectives. Through extensive experiments and ablations on two summarization datasets, we show that CLP learns steerable language models that outperform and Pareto-dominate the existing approaches for multi-objective finetuning.
Keywords: Reinforcement Learning, Multi-Objective Finetuning, Multi-task Learning, Parameter Efficient Training
X 🔗: x.com/kaiwenw_ai/status/1855304823760970056
Full Paper: arxiv.org/abs/2407.15762
Abstract: Reward-based finetuning is crucial for aligning language policies with intended behaviors (e.g., creativity and safety). A key challenge is to develop steerable language models that trade-off multiple (conflicting) objectives in a flexible and efficient manner. This paper presents Conditional Language Policy (CLP), a general framework for finetuning language models on multiple objectives. Building on techniques from multi-task training and parameter-efficient finetuning, CLP learn steerable models that effectively trade-off conflicting objectives at inference time. Notably, this does not require training or maintaining multiple models to achieve different trade-offs between the objectives. Through extensive experiments and ablations on two summarization datasets, we show that CLP learns steerable language models that outperform and Pareto-dominate the existing approaches for multi-objective finetuning.
Keywords: Reinforcement Learning, Multi-Objective Finetuning, Multi-task Learning, Parameter Efficient Training
มุมมอง: 104
วีดีโอ
Day of a Computer Science PhD at Cornell
มุมมอง 47K3 ปีที่แล้ว
A day in the life of a Computer Science PhD at Cornell University (Ithaca Campus). Music Adventures - A Himitsu Destination - MBB Sparks - Chaël Rainbow - JayJen
Construction of suffix arrays
มุมมอง 12K6 ปีที่แล้ว
A tutorial on the efficient construction of suffix arrays in O(n log(n))
Mazify! CMU 15-112 Term Project
มุมมอง 1.1K8 ปีที่แล้ว
Mazify! 15-112 Term Project for Fall 2016 at Carnegie Mellon University. Written in Python3, with Tkinter and Pillow, using techniques such as recursive backtracking, k-means segmentation and graph theory. Github: github.com/kaiwenw/Mazify-