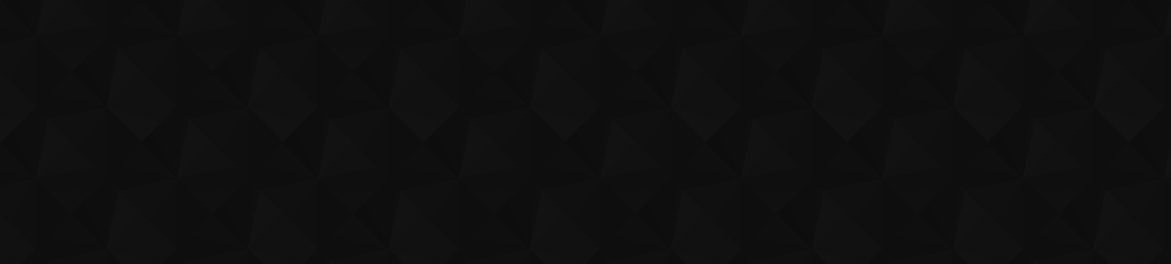
- 7
- 28 615
Davis Winston Rempe
เข้าร่วมเมื่อ 14 ก.พ. 2018
Trace and Pace: Controllable Pedestrian Animation via Guided Trajectory Diffusion (CVPR 2023)
Project page: research.nvidia.com/labs/toronto-ai/trace-pace/
Abstract: We introduce a method for generating realistic pedestrian trajectories and full-body animations that can be controlled to meet user-defined goals. We draw on recent advances in guided diffusion modeling to achieve test-time controllability of trajectories normally only associated with rule-based systems. Our guided diffusion model allows users to constrain trajectories through target waypoints, speed, and specified social groups while accounting for the surrounding environment context. This trajectory diffusion model is integrated with a novel physics-based humanoid controller to form a closed-loop, full-body pedestrian animation system capable of placing large crowds in a simulated environment with varying terrains. We further propose utilizing the value function learned during RL training of the animation controller to guide diffusion to produce trajectories better suited for particular scenarios such as collision avoidance and traversing uneven terrain.
Abstract: We introduce a method for generating realistic pedestrian trajectories and full-body animations that can be controlled to meet user-defined goals. We draw on recent advances in guided diffusion modeling to achieve test-time controllability of trajectories normally only associated with rule-based systems. Our guided diffusion model allows users to constrain trajectories through target waypoints, speed, and specified social groups while accounting for the surrounding environment context. This trajectory diffusion model is integrated with a novel physics-based humanoid controller to form a closed-loop, full-body pedestrian animation system capable of placing large crowds in a simulated environment with varying terrains. We further propose utilizing the value function learned during RL training of the animation controller to guide diffusion to produce trajectories better suited for particular scenarios such as collision avoidance and traversing uneven terrain.
มุมมอง: 1 941
วีดีโอ
STRIVE: Generating Useful Accident-Prone Driving Scenarios via a Learned Traffic Prior (CVPR 2022)
มุมมอง 1.9K2 ปีที่แล้ว
Project page: nv-tlabs.github.io/STRIVE/ Abstract: Evaluating and improving planning for autonomous vehicles requires scalable generation of long-tail traffic scenarios. To be useful, these scenarios must be realistic and challenging, but not impossible to drive through safely. In this work, we introduce STRIVE, a method to automatically generate challenging scenarios that cause a given planner...
HuMoR: 3D Human Motion Model for Robust Pose Estimation (ICCV 2021)
มุมมอง 14K3 ปีที่แล้ว
Project Page: geometry.stanford.edu/projects/humor/ Abstract: We introduce HuMoR: a 3D Human Motion Model for Robust Estimation of temporal pose and shape. Though substantial progress has been made in estimating 3D human motion and shape from dynamic observations, recovering plausible pose sequences in the presence of noise and occlusions remains a challenge. For this purpose, we propose an exp...
CaSPR: Learning Canonical Spatiotemporal Point Cloud Representations (NeurIPS 2020)
มุมมอง 9294 ปีที่แล้ว
Presented at Advances in Neural Information Processing Systems (NeurIPS) 2020 [Spotlight]. Project Page: geometry.stanford.edu/projects/caspr/ Abstract: We propose CaSPR, a method to learn object-centric Canonical Spatiotemporal Point Cloud Representations of dynamically moving or evolving objects. Our goal is to enable information aggregation over time and the interrogation of object state at ...
Contact and Human Dynamics from Monocular Video (ECCV 2020)
มุมมอง 10K4 ปีที่แล้ว
Presented at the European Conference on Computer Vision (ECCV) 2020. Project page: geometry.stanford.edu/projects/human-dynamics-eccv-2020 Abstract: Existing deep models predict 2D and 3D kinematic poses from video that are approximately accurate, but contain visible errors that violate physical constraints, such as feet penetrating the ground and bodies leaning at extreme angles. In this paper...
Predicting the Physical Dynamics of Unseen 3D Objects (WACV 2020)
มุมมอง 4895 ปีที่แล้ว
Presented at WACV 2020. Abstract: Machines that can predict the effect of physical interactions on the dynamics of previously unseen object instances are important for creating better robots and interactive virtual worlds. In this work, we focus on predicting the dynamics of 3D objects on a plane that have just been subjected to an impulsive force. In particular, we predict the changes in state...
Learning Generalizable Final-State Dynamics of 3D Rigid Objects
มุมมอง 1455 ปีที่แล้ว
Presented at CVPR 2019 Workshop on 3D Scene Understanding for Vision, Graphics, and Robotics. Humans have a remarkable ability to predict the effect of physical interactions on the dynamics of objects. Endowing machines with this ability would allow important applications in areas like robotics and autonomous vehicles. In this work, we focus on predicting the dynamics of 3D rigid objects, in pa...