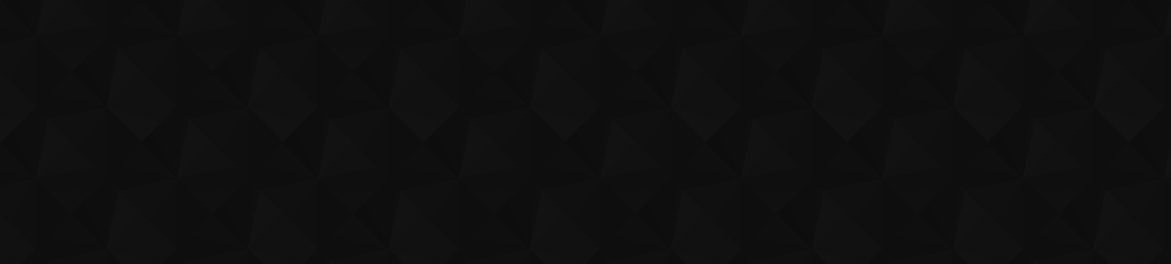
- 13
- 80 246
AI with Dr. Mo
เข้าร่วมเมื่อ 2 ต.ค. 2011
I am a data scientist who is responsible for creating predictive models and "putting them into production". I enjoy sharing my experience in predictive modeling and this channel is created to give examples on practical machine learning.
Testing Anomaly Detection Models
Anomaly or novelty detection is a way to automatically identify discrepancies in any data such as financial, medical, or manufacturing data. We have seen different ways of training (fitting) and predicting such anomalies however, testing the model accuracy is important and missing step that I am going to cover in this video. Feel free to comment your questions and also take a look at the following references,
Code:
github.com/mesmalif/MVP/blob/develop/anomaly_detection/unsupervised_testing/sales_anomalies.ipynb
💻 Store sales anomaly detection th-cam.com/video/WjpYqvMtYlQ/w-d-xo.html
💻 Anomaly detection with isolation forest th-cam.com/video/qNDcPUeCEPI/w-d-xo.html
💻 Anomaly detection with KNN th-cam.com/video/RwmttGrJs08/w-d-xo.html
Code:
github.com/mesmalif/MVP/blob/develop/anomaly_detection/unsupervised_testing/sales_anomalies.ipynb
💻 Store sales anomaly detection th-cam.com/video/WjpYqvMtYlQ/w-d-xo.html
💻 Anomaly detection with isolation forest th-cam.com/video/qNDcPUeCEPI/w-d-xo.html
💻 Anomaly detection with KNN th-cam.com/video/RwmttGrJs08/w-d-xo.html
มุมมอง: 1 532
วีดีโอ
How to learn time series in 5 minutes: P2-Univariate multi step out time series prediction
มุมมอง 1.1K2 ปีที่แล้ว
Many practical prediction problems have time component and the seasonality inside these dates has valuable information that cannot be neglected. Time series problems can be categorized into 4 groups, 1- Univariate (one feature to use in training) and single step (predicting just one point in the future) 2- Multivariate (multiple features to use in training) and single step (predicting just one ...
How to learn time series in 5 minutes: P1-Univariate single step out time series prediction
มุมมอง 5312 ปีที่แล้ว
Q: Why time series? A: Many practical prediction problems have time component and the seasonality inside these dates has valuable information that cannot be neglected. Time series problems can be categorized into 4 groups, 1- Univariate (one feature to use in training) and single step (predicting just one point in the future) 2- Multivariate (multiple features to use in training) and single ste...
How to find anomalies in store sales data and make it an AI/ML product
มุมมอง 2.1K2 ปีที่แล้ว
Anomaly detection is an interesting topic in machine learning where you can train models even without labels (unsupervised learning) to detect anomalies in the data. This would be great in auditing of finance, predictive maintenance etc. where management wants to see problems in current processes and correct them. Code: github.com/mesmalif/MVP/tree/develop/store_anomaly 🔴 Subscribe for more ML ...
How to create minimum viable product for machine learning projects - Weather prediction
มุมมอง 5702 ปีที่แล้ว
Creating MVP for ML projects is an interesting topic because of quick feedback it provides for engaged partners (managers, clients, etc.) and can catch problems early in the process of product development. These feedbacks can also be used in improving next versions of product. In this video, I will show how a sample project can be analysed and then converted to a MVPs. I have kept it simple to ...
Regression Analysis Part 1 of 2 (theory)
มุมมอง 2404 ปีที่แล้ว
Regression analysis is a way to examine the relationship of two or more variables (also called predictors or inputs) with one or more output variable (also call target(s)). It can predict continuous values such as temperature for tomorrow or number of visitors in a website. This would be extremely useful when you want to proactively plan for the coming days...
How to clean and prepare your data using Python
มุมมอง 3.6K4 ปีที่แล้ว
Collected data in real-world applications has missing values, anomalies or even data types could be wrong. Many believe that 70% or more of spent hours in machine learning projects belong to collecting, and cleaning of the data. In this video, we will talk about different steps that are common in data preparation. Also, the following links help you to navigate easier to the portion of the video...
Anomaly detection using iforest
มุมมอง 19K4 ปีที่แล้ว
Anomaly detection is an interesting topic that is gaining interest in different industries. Anomaly detection algorithms in health care can point to health issues of patients and in the financial world, they can flag frauds. Isolation forest algorithm first was introduced in 2008 and gained a lot of interest since then. git link: github.com/mesmalif/Practical_Machine_learning/tree/develop_pract...
Anomaly detection with KNN
มุมมอง 11K4 ปีที่แล้ว
How do you know something is not right or it is far from the normal situation? Mathematically, if we can measure the distance between the new observation and the rest of the dataset (observed earlier), we can judge the closeness of this new data point to the historical dataset. In many applications, if we have fair confidence in the normality of the historical dataset, the low distance would sh...
SESCAD Ground Grid Studies Part 3
มุมมอง 11K7 ปีที่แล้ว
The CDEGS software package (Current Distribution, Electromagnetic Fields, Grounding and Soil Structure Analysis) is a powerful set of integrated engineering software tools designed to accurately analyze problems involving grounding/earthing, electromagnetic fields, electromagnetic interference including AC/DC interference mitigation studies and various aspects of cathodic protection and anode b...
RESAP Ground GRID studies Part 2
มุมมอง 8K7 ปีที่แล้ว
The CDEGS software package (Current Distribution, Electromagnetic Fields, Grounding and Soil Structure Analysis) is a powerful set of integrated engineering software tools designed to accurately analyze problems involving grounding/earthing, electromagnetic fields, electromagnetic interference including AC/DC interference mitigation studies and various aspects of cathodic protection and anode b...
RESAP Grounding Grid Studies Part 1
มุมมอง 7K7 ปีที่แล้ว
The CDEGS software package (Current Distribution, Electromagnetic Fields, Grounding and Soil Structure Analysis) is a powerful set of integrated engineering software tools designed to accurately analyze problems involving grounding/earthing, electromagnetic fields, electromagnetic interference including AC/DC interference mitigation studies and various aspects of cathodic protection and anode b...
CDEGS Grounding Power Systems Tutorial-Part 1 Introduction
มุมมอง 15K7 ปีที่แล้ว
The CDEGS software package (Current Distribution, Electromagnetic Fields, Grounding and Soil Structure Analysis) is a powerful set of integrated engineering software tools designed to accurately analyze problems involving grounding/earthing, electromagnetic fields, electromagnetic interference including AC/DC interference mitigation studies and various aspects of cathodic protection and anode b...
Murphy Vista
hello, where i can find your dataset?? can you share?
Dr. Esmalifalak, thank you so much for your teaching in a short time. I wonder that there is any relationship between number of steps and lags in LSTM? What will happens if number of lags variables are far less than number of steps? Technically, it is possible, because the lstm looks for finding coefficients for a function makes between inputs and outputs. Is there any rule to limit number of time steps based on lag variables? for example, if the lag variables are 8, then number of time step (future step for prediction) must be less than 8. Thank you so much for your consideration in advance.
How to download
please contact the product customer service directly. Thanks
Hello dr. Mo
I want to hire you as my CDEGS tutor asap. Please reponse
I have very important questions regarding the CDEGS. Please reply if you are existed.
Dr. Moe, I have been looking for you regarding CDEGS If you received this message please reply.
Thank you Dr.Esmalifalak, I have a question regarding the sliding window approach that you used for time series data. Due to the sequence of output, there will be multiple predictions for a single timestep, resulting in overlapping predictions. I am curious about how you handled this while evaluation model ? And plotting the predictions result ? Thanks a lot !!
Thanks Gülşah for your comment. You can handle evaluation and plotting by averaging predictions (the most common way), selecting the most recent prediction, or modifying your evaluation metrics to account for overlaps. When plotting, you can either average predictions or use transparency to visualize overlaps. If you have enough time, it is always recommended to try different methods and see which one works better for your application.
thanks you profesor, just a question. Is possible deal with categorical variables? Is important the type of enconding to use (one hot or label enconding)? Thanks you in advance
Joshua, Thanks for your comment. Yes it is possible! You can use Extended Isolation Forest (EIF). Please take a look at this page for more info and a python example: capable-timimus-00a.notion.site/Isolation-Forest-in-Categorical-Values-b5534c14548b4ba881199477939044c2
Step stone of DS projects ... Plz make video on it to work with this step with customisable pipelines for different usecases .
Thanks, really helpful video
Thank you for the video. I found it very informative Can you please show how to run .py files for example where do we need to give filepath name and filter city name and can you also please show how the results looks like that are generated from .py file Thank you!
I have a question, this is an unsupervised model, right? is there a way to make the model predict a user input?
This is unsupervised anomaly detection method. It can be applied to user input data to detect anomalies or unusual patterns in user behavior over time. The basic idea is to use the algorithm to learn the normal patterns of user behavior based on the historical data, and then to use the model to identify any deviations from these patterns.
Great video!
😘 ᎮᏒᎧᎷᎧᏕᎷ
Thanks for the nice topic. I am wondering if we can do this considering the effect of seasonality? Like, lagging the sales values multiple times and creating new features and then training and testing the anomaly detector?
you can do that for sure then train/test your model with similar approach.
Excellent Dr.Mohammad. Are these types of algorithms (KNN) considered as weak algorithms in ensemble learning? Please make the similar video and post for other algorithms.
Thanks Dr. Esmalifalak. Your explanation is very useful. How does the accuracy of the program change by changing the step size and log? Will the changes be noticeable? Also, I would appreciate it if you could post a similar video about multi-variable.
Thanks Peyman. For the accuracy it is usually better to grid search different hyper-parameters such as number of lags. Trying different lags and testing the predictions (by walk forward method for example) would generally reveal the skill of different combinations of hyperparameters. I will have a video on the testing of time-series so stay tuned!
Thank you so much. This was really helpful👌
Thanks Dr Mo.
The greatest ml videos in TH-cam
Thanks Seyed!
@@AIwithDrMo Dr Mo I am looking for you regarding the CDEGS. Please reply
Timecodes 0:00 - Intro 0:19 - Problem Definition 2:14 - Importing Data 4:46 - Changing data types - to_datetime 5:48 - Changing data types - LabelEncoder 8:28 - Reindexing - set_index 9:47 - Converting time series to conventional ML problem by shifting dataframe 18:55 - Model training 23:28 - Model evaluation 28:00 - Creating python files for MVP 29:32 - train.py 36:51 - predict.py
Hi Dr. Esmalifalak, I'm a huge fan of all your videos, they've helped me with getting through university and get a career, can you please upload more videos, what data visualization tool do you use?
You can help me with a master’s thesis for my software part (coding) in Python?
Please fill out the following form for any specific questions, forms.gle/Jz4pkrNSGUqGhPug9
@@AIwithDrMo I can connect with you by email?
You can help me with a master’s thesis for my software part (coding) in Python?
Please fill out the following form for any specific questions, forms.gle/Jz4pkrNSGUqGhPug9
@@AIwithDrMo I can connect with you by email?
thank sir
can you please provide the code.
github.com/mesmalif/Practical_Machine_learning/tree/develop_practical_ML
Just amazing
excellent!!
Thanks mate.
Sir where is part 2 of this section
It is hard to find such good explanations on Isolation Forest. Keep up the good work!
very helpful video; I want to ask one question about time series part; you have entered n_neighbours=5 why 5? What about if it is 2 or 3 or 4? If I use time series anomaly detection part for 4 - 5 sensors column data; what should I choose for n_neighbours parameter? again 5?
Thanks Ugur. n_neighbours depends on your application and we usually try different ones to see if the outputs makes sense for this specific project or not.
A very well-structured but simple way of explanation. Can we also have a look at measuring the efficacy of the model?
Thanks for the comment. Isolation Forest is an effective anomaly detection method that can handle high-dimensional data and has several advantages over other methods. Its efficacy depends on the specific characteristics of the data and hyperparameters used. For example, the performance of the algorithm can be affected by the choice of subsampling ratio, the number of trees in the forest, and the choice of distance metric used to evaluate the splits.
thank you sir.
Please make another video on, Anomaly detection One-class SVM for Novelty detection
Thx, I will apply it~~
Hi, good job, I have a question, how we can resample according to the year?
I usually use 12 months resampling like "resample('12M')"
good video. suggest to turn up the volume. good content nonetheless. thanks
Hi, thanks I found it really helpful, but I have a question about the Contamination parameter, how we can choose a suitable value for this parameter?
glad you liked it. Contamination should be tested for your application. You can start with small numbers ( like 2%) and look at the results. If algorithm catches things that are normal to you, you may decrease the threshold otherwise keep increasing it ... You will find something reasonable for the data set you are working with.
@@AIwithDrMo Thanks a lot for your explanation.
I really love your video. could i ask if there is part 2 of 2 for this section? thank you very much!
thanks for this video! its not easy to find high quality content like this! keep it up!
Thanks for the clarification ! after applying iforest , how can I evaluate the cluster's result ? do you have specific method used for evaluation this type of unsupervised learning? I'd really appreciate that.
I usually prefer to have a small labeled dataset (from client etc.) and validate my results with those labels.
Hi! Thanks for the great tutorial. But I have a question, is it possible that isolation forest output different result? I have used isolation forest on my dataset, but the output results are a bit different than previous results everytime (I haven't changed any parameter in the model and the dataset I used is the same).
Thanks Johnson. Isolation forest randomly splits the datasets so there is no guarantee to have exactly the same results each time but, if you do it enough times and average out the results, it should converge to one solution (with reasonable data sets of course).
@@AIwithDrMo Thank you! Dr. Mohammad
Hello Dr. Mohammad, Is the algorithm effective with the real time streaming data? I have sensor data of around more than 100 sensors, should I need to find the important variables before feeding into the model or should I pass all the variables and let the algorithm decide by itself? Multicollinearity exist in the data .
Hi Aradhna, Isolation forest is one of the fast algorithms in anomaly detection and people use it with large datasets like financial datasets. For sensor data you don't have to process very high frequency data. You may need to find the right sampling rate (for example temperature usually is not changing sooner that 10-20 sec so sampling every second is not necessary ). If your window is 1 minute, you should not have noticeable problem in a regular application. I usually start will all of the data and the drop/minimize if I have to...
You solved a big problem for me,thank you
I am glad that helped you.
Thank you Mohammad
I am glad that you liked it.
Great explanation...
Thanks Reza. I'm glad you liked it.
Hello! Just a question. Is this an algorithm a classic isolation forest or an extended isolation forest (I saw you named the object with the predictions eif)? Is there any way to implement an extended isolation forest? Basically the difference between EIF and IF is that the EIF takes random intercept and slope and does the split based on the trend line. Thank you for the video!
Hi Vladimir This is classic isolation forest and as you mentioned, EIF can also be used similarly.