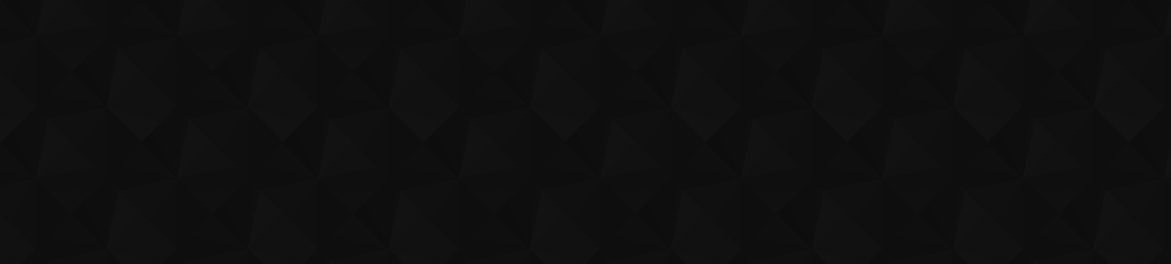
- 220
- 92 282
Hany Farid, Professor at UC Berkeley
United States
เข้าร่วมเมื่อ 17 ส.ค. 2018
Deepfakes
The technology that can distort and manipulate digital media is developing at break-neck speeds, and it is imperative that the technology that can detect such alterations develop just as quickly. The field of photo forensics seeks to restore some trust to photography.
At their foundation, photo forensic techniques rely on understanding and modeling the imaging pipeline, from the interaction of light with the physical 3-D world, the refraction of light as it passes through the camera lenses, the transformation of light to electrical signals in the camera sensor, and, finally, the conversion of electrical signals into a digital image file. This set of lectures focuses on the first part of the imaging pipeline, so-called physics-based techniques.
At their foundation, photo forensic techniques rely on understanding and modeling the imaging pipeline, from the interaction of light with the physical 3-D world, the refraction of light as it passes through the camera lenses, the transformation of light to electrical signals in the camera sensor, and, finally, the conversion of electrical signals into a digital image file. This set of lectures focuses on the first part of the imaging pipeline, so-called physics-based techniques.
มุมมอง: 770
วีดีโอ
Ballistic Motion
มุมมอง 16510 หลายเดือนก่อน
The technology that can distort and manipulate digital media is developing at break-neck speeds, and it is imperative that the technology that can detect such alterations develop just as quickly. The field of photo forensics seeks to restore some trust to photography. At their foundation, photo forensic techniques rely on understanding and modeling the imaging pipeline, from the interaction of ...
3D Modelling
มุมมอง 15910 หลายเดือนก่อน
The technology that can distort and manipulate digital media is developing at break-neck speeds, and it is imperative that the technology that can detect such alterations develop just as quickly. The field of photo forensics seeks to restore some trust to photography. At their foundation, photo forensic techniques rely on understanding and modeling the imaging pipeline, from the interaction of ...
Specularities
มุมมอง 7710 หลายเดือนก่อน
The technology that can distort and manipulate digital media is developing at break-neck speeds, and it is imperative that the technology that can detect such alterations develop just as quickly. The field of photo forensics seeks to restore some trust to photography. At their foundation, photo forensic techniques rely on understanding and modeling the imaging pipeline, from the interaction of ...
Lighting
มุมมอง 10610 หลายเดือนก่อน
The technology that can distort and manipulate digital media is developing at break-neck speeds, and it is imperative that the technology that can detect such alterations develop just as quickly. The field of photo forensics seeks to restore some trust to photography. At their foundation, photo forensic techniques rely on understanding and modeling the imaging pipeline, from the interaction of ...
Human Abilities and Limits
มุมมอง 10410 หลายเดือนก่อน
The technology that can distort and manipulate digital media is developing at break-neck speeds, and it is imperative that the technology that can detect such alterations develop just as quickly. The field of photo forensics seeks to restore some trust to photography. At their foundation, photo forensic techniques rely on understanding and modeling the imaging pipeline, from the interaction of ...
Lens Flare
มุมมอง 9810 หลายเดือนก่อน
The technology that can distort and manipulate digital media is developing at break-neck speeds, and it is imperative that the technology that can detect such alterations develop just as quickly. The field of photo forensics seeks to restore some trust to photography. At their foundation, photo forensic techniques rely on understanding and modeling the imaging pipeline, from the interaction of ...
Shadows
มุมมอง 14710 หลายเดือนก่อน
The technology that can distort and manipulate digital media is developing at break-neck speeds, and it is imperative that the technology that can detect such alterations develop just as quickly. The field of photo forensics seeks to restore some trust to photography. At their foundation, photo forensic techniques rely on understanding and modeling the imaging pipeline, from the interaction of ...
Vanishing Points and Lines
มุมมอง 33511 หลายเดือนก่อน
The technology that can distort and manipulate digital media is developing at break-neck speeds, and it is imperative that the technology that can detect such alterations develop just as quickly. The field of photo forensics seeks to restore some trust to photography. At their foundation, photo forensic techniques rely on understanding and modeling the imaging pipeline, from the interaction of ...
Reflections
มุมมอง 14311 หลายเดือนก่อน
The technology that can distort and manipulate digital media is developing at break-neck speeds, and it is imperative that the technology that can detect such alterations develop just as quickly. The field of photo forensics seeks to restore some trust to photography. At their foundation, photo forensic techniques rely on understanding and modeling the imaging pipeline, from the interaction of ...
Introduction
มุมมอง 1.2K11 หลายเดือนก่อน
The technology that can distort and manipulate digital media is developing at break-neck speeds, and it is imperative that the technology that can detect such alterations develop just as quickly. The field of photo forensics seeks to restore some trust to photography. At their foundation, photo forensic techniques rely on understanding and modeling the imaging pipeline, from the interaction of ...
Closing: parting thoughts
มุมมอง 160ปีที่แล้ว
Learn Computer Vision: These lectures introduce the theoretical and practical aspects of computer vision from the basics of the image formation process in digital cameras, through basic image processing, space/frequency representations, and techniques for image analysis, recognition, and understanding.
Image understanding: unsupervised learning: tSNE: implementation
มุมมอง 211ปีที่แล้ว
Learn Computer Vision: These lectures introduce the theoretical and practical aspects of computer vision from the basics of the image formation process in digital cameras, through basic image processing, space/frequency representations, and techniques for image analysis, recognition, and understanding.
Image understanding: unsupervised learning: t-distributed stochastic neighbor embedding (tSNE)
มุมมอง 181ปีที่แล้ว
Learn Computer Vision: These lectures introduce the theoretical and practical aspects of computer vision from the basics of the image formation process in digital cameras, through basic image processing, space/frequency representations, and techniques for image analysis, recognition, and understanding.
Image understanding: unsupervised learning: principal component analysis (PCA): eigenfaces
มุมมอง 151ปีที่แล้ว
Learn Computer Vision: These lectures introduce the theoretical and practical aspects of computer vision from the basics of the image formation process in digital cameras, through basic image processing, space/frequency representations, and techniques for image analysis, recognition, and understanding.
Image understanding: unsupervised learning: principal component analysis (PCA): computation
มุมมอง 106ปีที่แล้ว
Image understanding: unsupervised learning: principal component analysis (PCA): computation
Image understanding: unsupervised learning: principal component analysis (PCA): implementation
มุมมอง 121ปีที่แล้ว
Image understanding: unsupervised learning: principal component analysis (PCA): implementation
Image understanding: unsupervised learning: principal component analysis (PCA): eigenvectors
มุมมอง 126ปีที่แล้ว
Image understanding: unsupervised learning: principal component analysis (PCA): eigenvectors
Image understanding: unsupervised learning: principal component analysis (PCA): covariance matrix
มุมมอง 193ปีที่แล้ว
Image understanding: unsupervised learning: principal component analysis (PCA): covariance matrix
Image understanding: unsupervised learning: principal component analysis (PCA): canonical basis
มุมมอง 119ปีที่แล้ว
Image understanding: unsupervised learning: principal component analysis (PCA): canonical basis
Image understanding: unsupervised learning: expectation/maximization: EM implementation
มุมมอง 78ปีที่แล้ว
Image understanding: unsupervised learning: expectation/maximization: EM implementation
Image understanding: unsupervised learning: expectation/maximization: M-step
มุมมอง 75ปีที่แล้ว
Image understanding: unsupervised learning: expectation/maximization: M-step
Image understanding: unsupervised learning: expectation/maximization: E-step
มุมมอง 83ปีที่แล้ว
Image understanding: unsupervised learning: expectation/maximization: E-step
Image understanding: unsupervised learning: expectation/maximization: EM
มุมมอง 89ปีที่แล้ว
Image understanding: unsupervised learning: expectation/maximization: EM
Image understanding: unsupervised learning: clustering: k-means implementation
มุมมอง 100ปีที่แล้ว
Image understanding: unsupervised learning: clustering: k-means implementation
Image understanding: unsupervised learning: clustering: k-means
มุมมอง 144ปีที่แล้ว
Image understanding: unsupervised learning: clustering: k-means
Image understanding: supervised learning: classification: ANN: convolutional
มุมมอง 103ปีที่แล้ว
Image understanding: supervised learning: classification: ANN: convolutional
Image understanding: supervised learning: classification: ANN: backpropagation
มุมมอง 86ปีที่แล้ว
Image understanding: supervised learning: classification: ANN: backpropagation
Image understanding: supervised learning: classification: ANN: universal approximation theorem
มุมมอง 77ปีที่แล้ว
Image understanding: supervised learning: classification: ANN: universal approximation theorem
Image understanding: supervised learning: classification: artificial neural networks: xor + hidden
มุมมอง 73ปีที่แล้ว
Image understanding: supervised learning: classification: artificial neural networks: xor hidden
Thank You for your Hard Work sir!!
I feel so grateful to learn from you sir!! Thank you✨
Thank You Sir!!
Thank You sir!!
very clear explanation, thank you!
Excellent Rendering. Great Video man :) <3
Thanks for the video
This is a very interesting lecture, but I would like to point out that the first example being used is not showing Jennifer Garner, its showing Jennifer Lawrence.
Thank you, very clear and interesting explanation.
This was awesome. Thank you from a Computer Vision student in the UK!
1:54 -- what last section?
See here for the full syllabus: farid.berkeley.edu/learnComputerVision/
Fantastic, thank you!!!
Holy shit why doesnt this have more views
can anyone give me the correct step for the laplacian pyramid?? 1. Take the image fi from stage i. 2. filter the image fi with a low pass filter and thus create the image Li 3. Downsample the image li and thus create the image fi+1 4. Calculate the difference image hi=fi-li 5. cache hi 6. Consolidate all images hi 7. Repeat the above step n times.
Here is some Python code for computing a Laplacian pyramid: # Laplacian pyramid import matplotlib.pyplot as plt import numpy as np import cv2 from scipy.signal import sepfir2d im = plt.imread( 'mandrill.png' ) # load image h = [1/16,4/16,6/16,4/16,1/16]; # blur filter N = 4 # number of pyramid levels # Gaussian pyramid G = [] G.append(im) # first pyramid level for k in range(1,N): # pyramid levels im2 = np.zeros( im.shape ) for z in range(3): im2[:,:,z] = sepfir2d( im[:,:,z], h, h ) # blur each color channel im2 = im2[0:-1:2, 0:-1:2,:] # down-sample im = im2 G.append(im2) # Laplacian pyramid L = [] for k in range(0,N-1): # pyramid levels l1 = G[k] l2 = G[k+1] l2 = cv2.resize(l2, (0,0), fx=2, fy=2) # up-sample D = l1 - l2 D = D - np.min(D) # scale in [0,1] D = D / np.max(D) # for display purposes L.append(D) L.append(G[N-1]) # display pyramid fig, ax = plt.subplots(nrows=1, ncols=N, figsize=(15, 7), dpi=72, sharex=True, sharey=True) for k in range(N-1,-1,-1): ax[k].imshow(L[k])
@@hanyfarid5019 Thank you so much for the reply would you please give me the correct algorithm step for this one?
such a wonderful explanation! thank you!
Can I get a lecture on SIFT?
I don't have lecture on SIFT, but this is a lecture on the related HOG features: th-cam.com/video/RaaGoB8XnxM/w-d-xo.html
I love how easy this is to understand. I guess there are multiple ways to accomplish a CE. In my text, its explained using XOR with NOR circuits to accomplish the same thing. Or, am I misunderstanding?
You are correct. There are several different ways to create a CE circuit. The way I show is perhaps the most straight-forward, but definitely not the most efficient.
P=NP : ^ )
Much much better than the channels with millions of subscribers. I love it...❤❤
Why didnt I find this channel earlier...
Dear Professor Hany Farid, Thank you for sharing this fantastic course on image filtering! I find your explanations to be extremely clear and engaging. I have a quick question regarding linear time-invariant functions at around the 3:29 and 3:57 minute marks. The formulas shown use h[-3-k] and h[-4-k]. I was wondering if this might be a typo, as I would have expected h[-1-k] and h[0-k] to be used instead, or if I am missing something.
I thought the same thing
A viewer noticed that there is a bug at the 03:43 mark (nice catch) but I accidentally deleted their comment (sorry viewer). The code at this mark should read: M1z@M1z.T (not M1z@M2z.T)
Obvious typo on line 9 at 3:43: should be M1z@M1z.T + ...
Good catch. The code at this mark should read: M1z@M1z.T (not M1z@M2z.T)
Thanks Professor for your knowledge
Very clear explanation, thank you
This is the most comprehensive CV course I have ever seen. I was always looking for something like this but I always found general datascience courses. Thank you so much.
you is a legend
amazing point of view for explaining number of the features we want for a model won't change the cost function.
Wonderful lesson! Thank you
Amazing! Thank you
thank you for these lecture series, they are interesting
Can't be simpler. Best explanation
Thank you
Wow the legend that you are. Thank you!!!
Wow such a clear and concise video! And you make sure to review material that student should know! Amazing
Awesome explanation!! As on all the videos. Thanks for the uploads! 🙌🙌
Thank professor its really helpful
Thank you for this real world problem exercise ! I guess any rigid motion may be explained as a "combination" that ?
Why didn't anyone comment on a really good explanation?
how do you generalize this model to a dataset that "don't pass through the origin" ? Do we need to "center" the data before using it ?
Yes, the data can be centered (i.e., zero meaned) by subtracting the mean of each component.
@@hanyfarid5019 thanks, really appreciated. your video are really cool !!
Loved it!!
Great work sir. I never see any one in you tube covers in this much depth❤😊your students will be lucky to have you
Thank you professor for your effort this was really well explained, looking forward to learn more from this playlist
at 6:21, may be the summation of impulses representation is not right if the unit impulse signal is considered to be centered.
In this formulation, we assume the unit impulse falls on an integer sampling lattice.
You are great at teaching. ❤
VV Good
awesome video thanks!
how comes this channel has only 500 subs very valuable stuff here
Hi Prof. Farid, At 3:50, since the "*" notation stands for convolution, shouldn't the filter be [1, -1]? Assuming right is the positive x direction The following is some python output: a = [0, 1, 0, 0] b = scipy.signal.convolve(a, [1, -1], mode='same') print(a) print(b) [0, 1, 0, 0] [ 0 1 -1 0] Similarly in 13:27, I think the d filter should be reversed.
This depends on the specific implementation of convolution. Some implementations yield the results you see here in which the filter is flipped, while others don't flip the filter. Your code here is a good way to determine how to specify your filter values.
Your videos are great! Thank you for sharing!