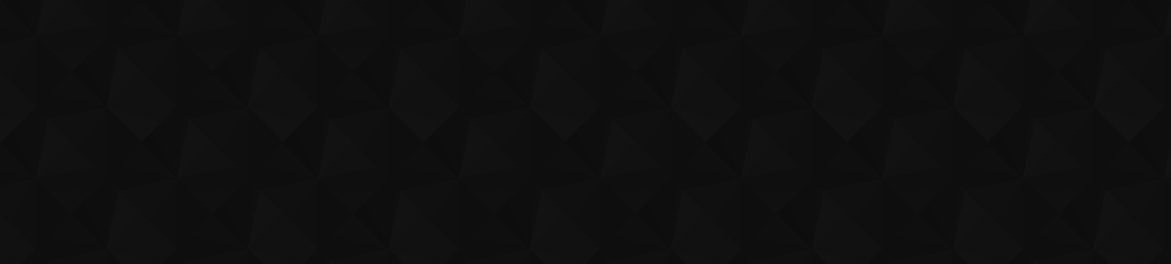
- 238
- 294 835
Tamer Elsayed
เข้าร่วมเมื่อ 20 ก.พ. 2011
This is an educational channel that offers learning material by Dr. Tamer Elsayed (Associate Professor of Computer Science at Qatar University).
Lecture 25 | Transformers for Images | CMPS 497 Deep Learning | Fall 2024
Lecture 25 of CMPS 497 Deep Learning, Fall 2024 undergraduate course at Qatar University by Dr. Tamer Elsayed (qufaculty.qu.edu.qa/telsayed/).
Slides are available at: www.dropbox.com/scl/fo/46brluxg9nt94r4ejq8gh/AD4R3owcIQgCKmcZTnG3Ox0?rlkey=nkjlb4fqxy0pgzinypcyjwblb&st=blze7083&dl=0
Slides are available at: www.dropbox.com/scl/fo/46brluxg9nt94r4ejq8gh/AD4R3owcIQgCKmcZTnG3Ox0?rlkey=nkjlb4fqxy0pgzinypcyjwblb&st=blze7083&dl=0
มุมมอง: 712
วีดีโอ
Lecture 24 | CNNs III | CMPS 497 Deep Learning | Fall 2024
มุมมอง 462หลายเดือนก่อน
Lecture 24 of CMPS 497 Deep Learning, Fall 2024 undergraduate course at Qatar University by Dr. Tamer Elsayed (qufaculty.qu.edu.qa/telsayed/). Slides are available at: www.dropbox.com/scl/fo/46brluxg9nt94r4ejq8gh/AD4R3owcIQgCKmcZTnG3Ox0?rlkey=nkjlb4fqxy0pgzinypcyjwblb&st=blze7083&dl=0
Lecture 23 | CNNs II | CMPS 497 Deep Learning | Fall 2024
มุมมอง 559หลายเดือนก่อน
Lecture 23 of CMPS 497 Deep Learning, Fall 2024 undergraduate course at Qatar University by Dr. Tamer Elsayed (qufaculty.qu.edu.qa/telsayed/). Slides are available at: www.dropbox.com/scl/fo/46brluxg9nt94r4ejq8gh/AD4R3owcIQgCKmcZTnG3Ox0?rlkey=nkjlb4fqxy0pgzinypcyjwblb&st=blze7083&dl=0
Lecture 22(b) | CNNs I | CMPS 497 Deep Learning | Fall 2024
มุมมอง 876หลายเดือนก่อน
Lecture 22(b) of CMPS 497 Deep Learning, Fall 2024 undergraduate course at Qatar University by Dr. Tamer Elsayed (qufaculty.qu.edu.qa/telsayed/). Slides are available at: www.dropbox.com/scl/fo/46brluxg9nt94r4ejq8gh/AD4R3owcIQgCKmcZTnG3Ox0?rlkey=nkjlb4fqxy0pgzinypcyjwblb&st=blze7083&dl=0
Lecture 22(a) | Transformers V (LLMs & Encoder-Decoder Models) | CMPS 497 Deep Learning | Fall 2024
มุมมอง 652หลายเดือนก่อน
Lecture 22(a) of CMPS 497 Deep Learning, Fall 2024 undergraduate course at Qatar University by Dr. Tamer Elsayed (qufaculty.qu.edu.qa/telsayed/). Slides are available at: www.dropbox.com/scl/fo/46brluxg9nt94r4ejq8gh/AD4R3owcIQgCKmcZTnG3Ox0?rlkey=nkjlb4fqxy0pgzinypcyjwblb&st=blze7083&dl=0
Lecture 21 | Transformers IV (Encoder- and Decoder-only Models) | CMPS 497 Deep Learning | Fall 2024
มุมมอง 793หลายเดือนก่อน
Lecture 21 of CMPS 497 Deep Learning, Fall 2024 undergraduate course at Qatar University by Dr. Tamer Elsayed (qufaculty.qu.edu.qa/telsayed/). Slides are available at: www.dropbox.com/scl/fo/46brluxg9nt94r4ejq8gh/AD4R3owcIQgCKmcZTnG3Ox0?rlkey=nkjlb4fqxy0pgzinypcyjwblb&st=blze7083&dl=0
Lecture 20 | Transformers III (Transformer Layer)| CMPS 497 Deep Learning | Fall 2024
มุมมอง 8132 หลายเดือนก่อน
Lecture 20 of CMPS 497 Deep Learning, Fall 2024 undergraduate course at Qatar University by Dr. Tamer Elsayed (qufaculty.qu.edu.qa/telsayed/). Slides are available at: www.dropbox.com/scl/fo/46brluxg9nt94r4ejq8gh/AD4R3owcIQgCKmcZTnG3Ox0?rlkey=nkjlb4fqxy0pgzinypcyjwblb&st=blze7083&dl=0
Lecture 19 | Transformers II (Part 2/2 of Self Attention) | CMPS 497 Deep Learning | Fall 2024
มุมมอง 6342 หลายเดือนก่อน
Lecture 19 of CMPS 497 Deep Learning, Fall 2024 undergraduate course at Qatar University by Dr. Tamer Elsayed (qufaculty.qu.edu.qa/telsayed/). Slides are available at: www.dropbox.com/scl/fo/46brluxg9nt94r4ejq8gh/AD4R3owcIQgCKmcZTnG3Ox0?rlkey=nkjlb4fqxy0pgzinypcyjwblb&st=blze7083&dl=0
Lecture 18(b) | Transformers I (Part 1/2 of Self Attention) | CMPS 497 Deep Learning | Fall 2024
มุมมอง 1.3K2 หลายเดือนก่อน
Part (b) of Lecture 18 of CMPS 497 Deep Learning, Fall 2024 undergraduate course at Qatar University by Dr. Tamer Elsayed (qufaculty.qu.edu.qa/telsayed/). Slides are available at: www.dropbox.com/scl/fo/46brluxg9nt94r4ejq8gh/AD4R3owcIQgCKmcZTnG3Ox0?rlkey=nkjlb4fqxy0pgzinypcyjwblb&st=blze7083&dl=0
Lecture 18(a) | Regularization III | CMPS 497 Deep Learning | Fall 2024
มุมมอง 3382 หลายเดือนก่อน
Part (a) of Lecture 18 of CMPS 497 Deep Learning, Fall 2024 undergraduate course at Qatar University by Dr. Tamer Elsayed (qufaculty.qu.edu.qa/telsayed/). Slides are available at: www.dropbox.com/scl/fo/46brluxg9nt94r4ejq8gh/AD4R3owcIQgCKmcZTnG3Ox0?rlkey=nkjlb4fqxy0pgzinypcyjwblb&st=blze7083&dl=0
Intro to Project | CMPS 497 Deep Learning | Fall 2024
มุมมอง 3912 หลายเดือนก่อน
Introduction to the project of CMPS 497 Deep Learning, Fall 2024 undergraduate course at Qatar University by Dr. Tamer Elsayed (qufaculty.qu.edu.qa/telsayed/). PyTorch Resources for Beginers: -Tutorials: pytorch.org/tutorials/beginner/basics/intro.html -Videos: th-cam.com/play/PL_lsbAsL_o2CTlGHgMxNrKhzP97BaG9ZN.html Paper: Conundrums in Cross-Prompt Automated Essay Scoring: Making Sense of the ...
Lecture 17 | Regularization II | CMPS 497 Deep Learning | Fall 2024
มุมมอง 6682 หลายเดือนก่อน
Lecture 17 of CMPS 497 Deep Learning, Fall 2024 undergraduate course at Qatar University by Dr. Tamer Elsayed (qufaculty.qu.edu.qa/telsayed/). Slides are available at: www.dropbox.com/scl/fo/46brluxg9nt94r4ejq8gh/AD4R3owcIQgCKmcZTnG3Ox0?rlkey=nkjlb4fqxy0pgzinypcyjwblb&st=blze7083&dl=0
Lecture 16 | Measuring Performance II, Regularization I | CMPS 497 Deep Learning | Fall 2024
มุมมอง 5632 หลายเดือนก่อน
Lecture 16 of CMPS 497 Deep Learning, Fall 2024 undergraduate course at Qatar University by Dr. Tamer Elsayed (qufaculty.qu.edu.qa/telsayed/). Slides are available at: www.dropbox.com/scl/fo/46brluxg9nt94r4ejq8gh/AD4R3owcIQgCKmcZTnG3Ox0?rlkey=nkjlb4fqxy0pgzinypcyjwblb&st=blze7083&dl=0
Gaza: A Flood of Messages | CMUQ | Oct 2024
มุมมอง 6962 หลายเดือนก่อน
No one can deny that we are living in a world that has critically changed since the dawn of October the 7th last year with what's happening in Gaza. Those major events have left us (and still!) with a myriad of messages. What can we learn at the personal level? What are the conceptual lessons we need to recognize? How should we change as students and professors? Can we really make a difference?...
Lecture 15 | Measuring Performance I | CMPS 497 Deep Learning | Fall 2024
มุมมอง 5462 หลายเดือนก่อน
Lecture 15 of CMPS 497 Deep Learning, Fall 2024 undergraduate course at Qatar University by Dr. Tamer Elsayed (qufaculty.qu.edu.qa/telsayed/). Slides are available at: www.dropbox.com/scl/fo/46brluxg9nt94r4ejq8gh/AD4R3owcIQgCKmcZTnG3Ox0?rlkey=nkjlb4fqxy0pgzinypcyjwblb&st=blze7083&dl=0
Lecture 14 | Model Initialization | CMPS 497 Deep Learning | Fall 2024
มุมมอง 6182 หลายเดือนก่อน
Lecture 14 | Model Initialization | CMPS 497 Deep Learning | Fall 2024
Lecture 13 | Backpropagation II | CMPS 497 Deep Learning | Fall 2024
มุมมอง 6672 หลายเดือนก่อน
Lecture 13 | Backpropagation II | CMPS 497 Deep Learning | Fall 2024
محاضرة قصيرة - "تحقق" - مؤتمر مجمع الملك سلمان للغة العربية - 7 أكتوبر 2024
มุมมอง 1913 หลายเดือนก่อน
محاضرة قصيرة - "تحقق" - مؤتمر مجمع الملك سلمان للغة العربية - 7 أكتوبر 2024
Lecture 12 | Backpropagation I | CMPS 497 Deep Learning | Fall 2024
มุมมอง 1.1K3 หลายเดือนก่อน
Lecture 12 | Backpropagation I | CMPS 497 Deep Learning | Fall 2024
Lecture 11 | Fitting Models II | CMPS 497 Deep Learning | Fall 2024
มุมมอง 5293 หลายเดือนก่อน
Lecture 11 | Fitting Models II | CMPS 497 Deep Learning | Fall 2024
Lecture 10 | Fitting Models I | CMPS 497 Deep Learning | Fall 2024
มุมมอง 7803 หลายเดือนก่อน
Lecture 10 | Fitting Models I | CMPS 497 Deep Learning | Fall 2024
Lecture 9 | Loss Functions III | CMPS 497 Deep Learning | Fall 2024
มุมมอง 5963 หลายเดือนก่อน
Lecture 9 | Loss Functions III | CMPS 497 Deep Learning | Fall 2024
Lecture 8 | Loss Functions II | CMPS 497 Deep Learning | Fall 2024
มุมมอง 8513 หลายเดือนก่อน
Lecture 8 | Loss Functions II | CMPS 497 Deep Learning | Fall 2024
Lecture 7 | Loss Functions I | CMPS 497 Deep Learning | Fall 2024
มุมมอง 8593 หลายเดือนก่อน
Lecture 7 | Loss Functions I | CMPS 497 Deep Learning | Fall 2024
Lecture 6 | Deep Neural Networks | CMPS 497 Deep Learning | Fall 2024
มุมมอง 1.3K4 หลายเดือนก่อน
Lecture 6 | Deep Neural Networks | CMPS 497 Deep Learning | Fall 2024
Lecture 5 | Shallow Neural Networks II | CMPS 497 Deep Learning | Fall 2024
มุมมอง 9814 หลายเดือนก่อน
Lecture 5 | Shallow Neural Networks II | CMPS 497 Deep Learning | Fall 2024
Lecture 4 | Shallow Neural Networks I | CMPS 497 Deep Learning | Fall 2024
มุมมอง 1.3K4 หลายเดือนก่อน
Lecture 4 | Shallow Neural Networks I | CMPS 497 Deep Learning | Fall 2024
Lecture 3 | Supervised Learning | CMPS 497 Deep Learning | Fall 2024
มุมมอง 1.5K4 หลายเดือนก่อน
Lecture 3 | Supervised Learning | CMPS 497 Deep Learning | Fall 2024
Lecture 2 | Introduction | CMPS 497 Deep Learning | Fall 2024
มุมมอง 3.1K4 หลายเดือนก่อน
Lecture 2 | Introduction | CMPS 497 Deep Learning | Fall 2024
Lecture 1 | Welcome to DL | CMPS497 Deep Learning | Fall 2024
มุมมอง 10K4 หลายเดือนก่อน
Lecture 1 | Welcome to DL | CMPS497 Deep Learning | Fall 2024
I just find it counter-intutive (it could be just me) when describing the encoder-decoder portion by using the previously Q (search bar)/K (searched items)/V (searched results). When doing translation from English to French, it is like quering in English to find which French words to use? If I put the 'above analogy' among Q, K, V aside and purely looking at it from the Matrix perspective, I think I can see why. First, we need the 'attention" between the English and French words. To get the French text, we apply the 'attention' matrix with the English text (input - V) to perform the 'transformation' to generate the output French. To fulfill the matrix dimension across, we need to position French in Q, English in V and K. Oh, if I transpose the "English to French;' Attention matrix, input the French instead, will I get the English? I guess so but likely improper translation. Sorry about the long text. BTW, very good lecture, many thanks
I was looking for a deep dive to deep learning till I found this on the udlbook website: Video lectures for chapters 1-12 from Tamer Elsayed of Qatar University. I think it's a great combo!
You are an excellent lecturer
Thank you 😊
Jazakallah Khair. I'm a student at AUS (in the UAE) going through the book. Delighted to find these great video lectures!
جزانا وإياكم. الحمد لله 😊
Very helpful
Great! Thank you
Rational mean .do the right thing
From my heart Thank you so much ❤❤❤
بارك الله فيك
مليون لايك يادكتور اسلوبك اكثر من رائع الله يوفقك
الحمد لله. بارك الله فيك
I know my suppose is not practical but wouldn't it be better if words are tokenized into +2d tensor instead of vector
I didn't get what you meant.
@tamer_elsayed in tokenization step (suppose words as tokens) are converted into vectors that in the next steps would be modified in heavy computation steps including billions of parameters but if in the beginning that vector wasn't just 1d vector but multi dimension tensor to bear more information -i know it will distort the pipeline of transformer- but would it reduce the burden of computations in the following step because the changes would be fewer later.
@@tamer_elsayed i know my question is vague but it is originated from nothing more than a gut feeling that the realm of natural language is better represented as graphs of densely connected nodes instead of isolated vectors connected to each other via a process that mimics covariance matrix calculation . I mean the heavy calculations required by transformers are originated from over simplication of 1st step to represent words as vectors and perhaps tokenizing words into more complex form in the beginning would counterintuitively simplify heavy computations later on. Sorry in advance for my silly question
Text is naturally a 1D sequence, but theoretically it can be more. You just need to establish an intuition.
Text as a graph of words is an idea that is used in many problems (e.g., keyword extraction), and it would be interesting to see it here. Remember that the attention mechanism tries to construct a kind of bipartite graph already.
اعتزل شرحك سيئ
شكرا لك. هل شاهدت المحاضرات الخمس السابقة؟
ليه الشرح بالانجليزي ليه ليه نفسي افهم؟! مفيش مشكلة بالنسبة للكتاب ولا المشكله بالمصطلحات تكون باللغة الام لهذا العلم، لكن الشرح يكون بالانجليزية والله هذي انا ما فهمتها
الدراسة بجامعة قطر باللغة الإنجليزية. هذا هو السبب ببساطة.
@tamer_elsayed الله يكون بعوننا بس، بدل ما تكون الجامعات هي اخذه على عاتقها نقل العلوم الى لغتنا، لا بدل من ذالك تحاول تبقيها كما هي بلغتها. على العموم مشكور جدا على نشرك لهذي المحاضرات، واشكرك على الاهتمام بالتعليقات والرد عليها
@@studentforever7665 بارك الله فيك
Excellent lectures
Thank you 😊
where can I find theory question with answer for this course
The textbook has questions for each chapter and some of them are answered.
ربنا يبارك في حضرتك ويجعل شرحك في ميزان حسناتك 💗💗💗
آميين يا رب العالمين. بارك الله فيك.
بارك الله فيك، ما اجمل أن يجتمع الدين مع العلم
كم أسعدني تعليقك! جزاك الله خيرا.
رفع الله قدركم. The intentions for learning is fabulous. May Allah reward you for them.
آميين. بارك الله فيك
Wow, what an amazing course Prof. Elsayed. Are you planning on continuing with the stable diffusion chapter of Prince's book? That would be awesome :)
Thanks a lot. Unfortunately, no, at least for now.
Thank You Sir! for providing such a valueable Lectures ....Your each & very word open a new way towards the life .. Thanks again
You are welcome 😊
Assalamu alaykum. Sir the example in the first poll quiz to find out whether a news article is political, economical or sports -- it is multiclass classification but why do we consider it univariate? Like in the previous examples of image segmentation into foreground and background, we considered each pixel value and then found two possible outputs out of them so it was multivariate binary classification problem, but in this news article problem, don't we consider all words indicating it multivariate ? Please can you guide. Jazakumullahu khayran
It's univariate because we have just *one overall output*, which is the class for the entire news article, not for each word.
Thank you teacher. I got it now.
reinforcement learning ( I will be happy to watch this course from you professor)
I'm not teaching it.
This is the first course I completed from start to finish over a period of 3 months time in last 12 years. Thank you so so much Sir. I just hope and wish we can get your next sem course on DS and Information Retrieval :)
That's great! Thanks a lot for following and watching every single lecture! I really hope it was enjoyable and beneficial. Let's hope for the best for DS and IR 🙂
May God reward you, sir. From start till the end your lecture was very engaging and full of knowledge. Thank you for teaching us about having good intentions and collective goals as commanded to us in our religion.May Allah Subhanahu wa Ta'ala grant barakah in your knowledge & allow us to learn from you. Aameen
Thanks a lot for your words. May ALLAH accept.
Thanks prof Tamer for this amazing playlist❤ Will you discuss the Residual Networks?
You are welcome. Unfortunately, no, due to tight time.
such wonderful lectures professor thank you for sharing them
You are very welcome
absolutely exceptional.
Thank you 😊
First viewer.As usual excellent tutorial
Thank you 😊
awsome lecture.Best among youtube lectures
Thanks a lot ☺️
Thank you sir for sharing free such a valuable tutorials
You are welcome
Can you upload the slides Sir from Transformers (b) onwards ?
Done.
@@tamer_elsayed Thank you so much sir
Thank you so so much sir. Every student on this planet deserve a teacher like you. This course motivated me to pursue my Masters from Spring 2025.
You are welcome 😊. Very pleased to hear that my course motivated you to pursue MSc 👍
جزاك الله عنا خير الجزاء يا دكتور
جزانا وإياك
Sir, a few thoughts: 1. Regarding multi-head attention, we use (D/H) and then concatenate all the outputs to get back the (D x N ) matrix at the end. This process seems perfect, but putting computational efficiency aside, if I use the same (D x N ) matrix size for all {Q, K, V}, can we expect the network to generate a better understanding of the text? This would mean performing transformations within the same vector space, whereas in the actual architecture, we lower the dimensionality to (D/H), which could result in some loss of information. This trade-off is made for computational efficiency and model performance. Is my understanding correct, or am I missing something obvious? 2. Regarding positional encoding, in the book (page 213, section 12.3.1), it says (and I quote): "The positional embedding vector may be added to the network inputs or at every network layer. Sometimes it is added to (X) in the computation of the queries and keys but not to the values." What’s the intuition behind adding positional encoding to queries and keys but not to values? If possible, could you share your thoughts on this in a future lectures? It would be very helpful. Thank you once again. Looking forward for the next class this week :)
1. There are several points here: a. With multi-head, we are not in the same vector space; each head works in a different space, even if we don't lower the dimension. b. With your suggestion, we will increase the number of parameters by a factor of H. c. Not sure if that would result in a better representation. 2. a. It's added to X when computing keys and queries, not to keys and queries directly. b. Not sure why not values too. I need to check the original paper. You are welcome 😊
@@tamer_elsayed Thank you so much sir 🙏
@@suman14san you are welcome
أرجو إضافه تكلمه للكورس advance IR ، حيث أن المحاضر يملك تجربه طويله في هذا المجال ونرغب بخبرته
أتمنى أن يتاح لي الجهد والوقت لذلك بإذن الله.
يا ريت تضيف تمارين لتثبيت أو إختبار المعلومه
التدريبات العملية المصاحبة للمادة موجودة بالفعل هنا: github.com/telsayed/IR-in-Arabic
Thank you sir!
You are welcome
Sir, Can you upload the Transformer lectures ? I guess I told this 19 times in last 19 lectures, but thank you so much once again for sharing your knowledge to the whole world. 🙏🙏
I just uploaded the slides covered so far. You are most welcome :)
would you suggest me which book i follow?
Artificial Intelligence: A Modern Approach
Very well illustrated lecture. Your students are lucky with you as a very professional professor. I am graduated from 2 years and I which I were one of your students. You helped me alot to understand concepts were very complicated. Very excited to the next lecture and very excited to hands on projects. Good luck!
Thanks a lot for the nice words, and happy to hear that my lectures were useful to you (الحمد لله). The next lecture has been posted now 🙂
show of good pedagogical skills, great lecture.
Thank you 😊
"سير، قم بتقديم تطبيقًا حقيقيًا لمساق هذا حتى أستطيع إنشاء شيء يمكنه مساعدة إخوتي وأخواتي في مواجهة أعداء الإسلام"
التركيز هنا على فهم الأساسيات بعمق لتطبيقها في أي مجال.
Great lecture,Previously I have followed cs224n,But this is among the best
Thank you 😊
sir wonderful lecture
Thank you 😊
Thank you!
You are welcome
Sir please share the papers and data
I have added the resources and paper to the description of the video.
Thank you so much, Sir! Could you please share the resources you mentioned on PyTorch, research papers, and other resources you mentioned as you share in the class? This is the first time I've been able to follow a course from the beginning and keep up while reading the UDL book. Teachers like you are truly special. I’ve started envisioning the day I can meet you in person and thank you directly.
I have added the resources and paper to the description of the video. Thanks for your words. I also wish we meet one day (God Willing).
@@tamer_elsayed Thank you so much Sir
You are welcome @@suman14san
Great work.
Thank you 😊
نفع الله بكم ..وجعل كلماتكم في ميزان حسناتك ان شاء الله
آميين يارب. بارك الله فيك.
الله يقويك دكتور.....كلام صادق وواضح أنه من القلب....أسأل الله أن يجعله في ميزان حسناتك.....اللهم استعملنا ولا تستبدلنا
آميين يارب العالمين. بارك الله فيك.
Thank u sir for this amazing clear tutorial. I have one question. He initialization is applicable for Relu activation.What about other activation functions? Please advise.
He initialization is suitable for ReLU. You might try other initialization techniques, such as glorot or xavier, for other activation functions.
In the regularization term, we should not add the bias terms ? sum_of_phi_square should have only weights in the model and not the bias terms ? @1:00:03 And once again, thank you so so much Sir for the lecture, every friday, I just wait for the next lecture, :)
Theoretically, it's all parameters, but usually, it's just the weights only (as biases are much fewer). And thanks a lot for your continuous following -- it's really rewarding to see someone is benefiting (thanks God) 😊