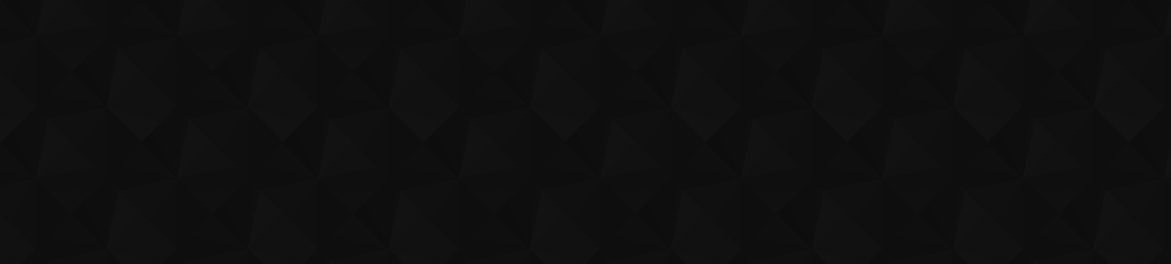
- 32
- 2 520
Maninda Edirisooriya
เข้าร่วมเมื่อ 27 ก.ย. 2013
Learn about Artificial Intelligence till you no longer need to learn anything, up until AI becomes a sufficiently advanced learner where you are nowhere near its capabilities of learning ;)
วีดีโอ
ML Strategy for Deep Learning
มุมมอง 20หลายเดือนก่อน
Machine Learning project strategy for optimizing an objective including Orthogonalization, Single Number Evaluation Metrics, Optimizing and Satisficing Metrics, Training, Dev (Validation) and Test Sets, changing the Metric Function for unexpected scenarios, Accuracy Level Benchmarks including Bayes Optimal Error, Human-level Performance, Variance and Avoidable Bias, and optimization decisions b...
Optimizing Deep Learning
มุมมอง 662 หลายเดือนก่อน
Effect of scaling features, problems of Vanishing Gradient and Exploding Gradient problems, the techniques to solve the problems including, properly initializing weights (Xavieror He initialization), Gradient Clipping, and using proper Activation Functions (e.g.: ReLU), Batch Normalization, Layer Normalization, alternative deep learning architectures (e.g.: LSTM or GRU for RNNs) and deep learni...
Introduction to Deep Learning
มุมมอง 772 หลายเดือนก่อน
Learn about Natural Neurons, Neural Networks, Artificial Neural Networks (ANNs), mathematical approximation of ANNs, Activation Functions, Neural Network Types, Single Layer Perceptron, Multi-Layer Perceptron, advantages of Deep Learning, Feature Hierarchies, trend of Deep Learning, and Deep Learning applications.
Bayesian Models
มุมมอง 763 หลายเดือนก่อน
Learn about Bayesian thinking, Bayes Theorem, Prior, Likelihood, Posterior, Conditional Independence, Naive Bayes, Naive Bayes Classifier Regularization, Laplace Smoothing, Lidstone Smoothing, Bayesian Networks (Bayesian Belief Networks), Bayesian Linear Regression, and Bayesian Optimization.
Support Vector Machines (SVM)
มุมมอง 1244 หลายเดือนก่อน
Learn about Support Vector Machines, Support Vectors, Hard SVM, Soft SVM, Primal Problem, Dual Problem, Regularization, Kernel Trick, Kennel Function, Gaussian Function/Radial Basis Function (RBF), Linear Kernel, Polynomial Kernel, and Support Vector Regression (SVR).
Model Testing and Evaluation
มุมมอง 444 หลายเดือนก่อน
Learn Data Balancing, Hyperparameter Tuning with Grid Search and Random Search, ML model evaluation, ML Performance Metrics, measure of performance of Classification Models, Confusion metrics, Accuracy, Precision, Recall (Sensitivity), Specificity, F-Measures, F1 score, ROC curves, AUC and Cross Validation.
Decision Trees and Ensemble Methods
มุมมอง 445 หลายเดือนก่อน
Learn Decision Trees (DT), Building Decision Trees, Optimizing Decision Trees, CART Algorithm, Gini Index, Entropy, Decision Tree Classification Geometry, Converting Continuous Features to Categorical, Bias-Variance Metrics of Decision Tree, Decision Tree Regularization, Ensemble Methods, Bootstrapping, Bagging, Boosting, Random Forrest, and XGBoost.
Gradient Descent
มุมมอง 735 หลายเดือนก่อน
Learn about the Gradient Descent algorithm for the example of Linear Regression, its parameter initialization, update rule, derivation and introduction to Batch Gradient Descent, Stochastic Gradient Descent and Mini-Batch Gradient Descent optimization.
Linear Regression
มุมมอง 545 หลายเดือนก่อน
Learn about Linear Regression, Mean Squared Error (MSE), Linear Regression, R-squired, Multiple Linear Regression, Simple Linear Regression, Linearity assumptions, Homoscedasticity, Multicollinearity, Variance Inflation Factor (VIF), Training and testing, Residual Sum of Squares, Total Sum of Squares, Polynomial Regression and Multivariate Polynomial Regression.
Introduction to Statistical & Machine Learning
มุมมอง 1076 หลายเดือนก่อน
Introduction to Statistical & Machine Learning
Awosome
Good Series... Thank you for this wonderful lecture