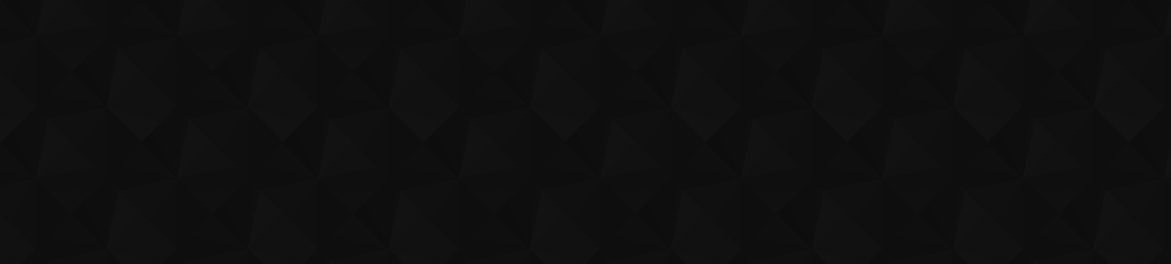
- 101
- 419 107
Berkeley AI
เข้าร่วมเมื่อ 21 ม.ค. 2015
Spring 2016 Section 12 (Neural Networks) Solutions
Spring 2016 Section 12 (Neural Networks) Solutions
มุมมอง: 3 063
วีดีโอ
Spring 2016 Section 11 (Neural Networks) Overview
มุมมอง 2.6K8 ปีที่แล้ว
Spring 2016 Section 11 (Neural Networks) Overview
Spring 2016 Section 11 (Perceptrons + Neural Networks) Solutions
มุมมอง 2.4K8 ปีที่แล้ว
Spring 2016 Section 11 (Perceptrons Neural Networks) Solutions
Spring 2016 Section 10 (Naive Bayes + Perceptrons) Solutions
มุมมอง 2.9K8 ปีที่แล้ว
Spring 2016 Section 10 (Naive Bayes Perceptrons) Solutions
Spring 2016 Section 10 (Naive Bayes + Perceptrons) Overview
มุมมอง 2.8K8 ปีที่แล้ว
Spring 2016 Section 10 (Naive Bayes Perceptrons) Overview
Spring 2016 Section 9 (HMMs + Particle Filters) Solutions
มุมมอง 4.1K8 ปีที่แล้ว
Spring 2016 Section 9 (HMMs Particle Filters) Solutions
Spring 2016 Section 9 (HMMs + Particle Filters) Overview
มุมมอง 6K8 ปีที่แล้ว
Spring 2016 Section 9 (HMMs Particle Filters) Overview
Spring 2016 Section 8 (Sampling + VPI) Solutions
มุมมอง 1.9K8 ปีที่แล้ว
Spring 2016 Section 8 (Sampling VPI) Solutions
Spring 2016 Section 8 (Sampling + VPI) Overview
มุมมอง 2.3K8 ปีที่แล้ว
Spring 2016 Section 8 (Sampling VPI) Overview
Spring 2016 Section 7 (Bayes Nets + Variable Elimination) Solutions
มุมมอง 7K8 ปีที่แล้ว
Spring 2016 Section 7 (Bayes Nets Variable Elimination) Solutions
Spring 2016 Section 7 (Bayes Nets + Variable Elimination) Overview
มุมมอง 12K8 ปีที่แล้ว
Spring 2016 Section 7 (Bayes Nets Variable Elimination) Overview
Spring 2016 Section 6 (RL + Probability) Solutions
มุมมอง 2.3K8 ปีที่แล้ว
Spring 2016 Section 6 (RL Probability) Solutions
Spring 2016 Section 6 (RL + Probability) Overview
มุมมอง 2.4K8 ปีที่แล้ว
Spring 2016 Section 6 (RL Probability) Overview
Spring 2016 Section 5 (MDPs + RL) Solutions
มุมมอง 6K8 ปีที่แล้ว
Spring 2016 Section 5 (MDPs RL) Solutions
Spring 2016 Section 5 (MDPs + RL) Overview
มุมมอง 3.2K8 ปีที่แล้ว
Spring 2016 Section 5 (MDPs RL) Overview
Spring 2016 Section 4 (Games + MDPs) Solutions
มุมมอง 2.7K8 ปีที่แล้ว
Spring 2016 Section 4 (Games MDPs) Solutions
Spring 2016 Section 4 (Games + MDPs) Overview
มุมมอง 2.5K8 ปีที่แล้ว
Spring 2016 Section 4 (Games MDPs) Overview
Spring 2016 Section 3 (CSPs + Games) Overview
มุมมอง 2.2K8 ปีที่แล้ว
Spring 2016 Section 3 (CSPs Games) Overview
Spring 2016 Section 3 (CSPs + Games) Solutions
มุมมอง 2.3K8 ปีที่แล้ว
Spring 2016 Section 3 (CSPs Games) Solutions
Spring 2016 Section 2 (Graph Search + CSPs) Solutions
มุมมอง 2.2K8 ปีที่แล้ว
Spring 2016 Section 2 (Graph Search CSPs) Solutions
Spring 2016 Section 2 (Graph search + CSPs) Overview
มุมมอง 2.2K8 ปีที่แล้ว
Spring 2016 Section 2 (Graph search CSPs) Overview
Spring 2016 Section 1 (Search) Solutions
มุมมอง 2.3K8 ปีที่แล้ว
Spring 2016 Section 1 (Search) Solutions