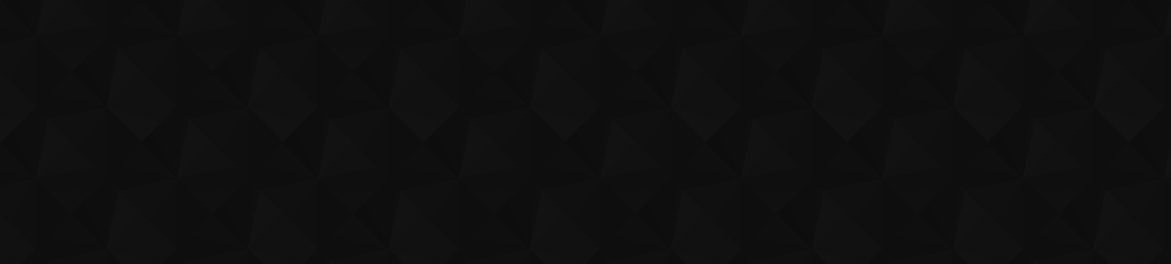
- 58
- 11 021
Sabudh Foundation
India
เข้าร่วมเมื่อ 5 ก.ค. 2018
PyTorch for Beginners - Insights by Research Assistant at IIIT Delhi, Jivjot Singh!
Ready to dive into the world of PyTorch and enhance your data science skills? Join Jivjot Singh for an engaging webinar where he’ll guide you through the essentials of PyTorch. Whether you’re new to machine learning or looking to solidify your foundation, this session is perfect for you.
Key Topics:
Introduction to PyTorch
Evolution of Deep Learning
Understanding basics of Tensors
PyTorch to build deep learning projects
Code walkthrough showcasing the abilities of autograd and optimizers
Key Topics:
Introduction to PyTorch
Evolution of Deep Learning
Understanding basics of Tensors
PyTorch to build deep learning projects
Code walkthrough showcasing the abilities of autograd and optimizers
มุมมอง: 64
วีดีโอ
Sabudh’s Data Science Internship Testimonial by Kumari Ratan Priya
มุมมอง 483 หลายเดือนก่อน
Meet Kumari Ratan Priya, a shining star from Sabudh's Data Science Batch, who recently completed her 6-month internship with us! Her journey is a testament to the incredible growth possible with Sabudh's immersive learning experience. Why Sabudh? ✅ Expert Guidance from Industry Leaders ✅ Hands-On Projects & Assignments ✅ Clear Career Trajectory Post-Program A big shoutout to Kumari Ratan Priya ...
Sabudh’s Data Science Internship Testimonial by Nikhil Sharma
มุมมอง 18310 หลายเดือนก่อน
Curious about the Sabudh Data Science Internship experience? Hear directly from Mr. Nikhil Sharma, one of our talented interns, as he share insights, challenges, and exciting moments during his internship journey. Nikhil's journey is a testament to the incredible growth possible with Sabudh's immersive learning experience. 📊 From Little Knowledge to Data Science Maestro! 🔍 Applications in Every...
Testimonial: How I started my Journey in the field of Data Science | Sabudh Foundation
มุมมอง 133ปีที่แล้ว
At Sabudh Foundation, we believe in nurturing the potential of young minds and providing them with the tools they need to succeed in the ever-evolving world of technology and innovation. In this video, we showcase the inspiring internship experiences of our learners, who have had the privilege of being mentored by industry experts and gaining hands-on experience in their respective fields. Dr. ...
Transforming Career from Computer Science Teacher to a skilled Data Scientist!" | Sabudh Foundation
มุมมอง 110ปีที่แล้ว
Welcome to the Sabudh Foundation TH-cam channel! In this video, we're excited to bring you real-life stories of transformation through our internship programs. 🌟 Internships are more than just a stepping stone; they are the foundation upon which young minds build their futures. Join us as we hear from our talented interns who share their incredible journeys, highlighting the challenges they fac...
Sabudh Data Science Pro 2020 Webinar with Mr. Marko Grobelnik
มุมมอง 963 ปีที่แล้ว
Sabudh Data Science Pro 2020 Webinar with Mr. Marko Grobelnik
It is possible to get the python scripts
Thanks for sharing. Nice testimonial.
Thanks for sharing, Nice testimonial.
Thanks for sharing. Nice testimonial.
Thanks for sharing
Great session and practical insight into this world by Dr Satnam. Can we get those references and specially the mind map of learning path( if it posted in Sabudh repo somewhere?)
Good sir
A very informative session!!
A very informative session!!
Thank you Sabudh for quality content.
Excellent talk. Thank you.
The QnA session was wonderful.
Status paga
Could you please tell how to grab an internship in data science in sabudh foundation
Hi Ayush. Apply at sabudh.org. our next batch starts in January so apply soon.
What is the distribution of random vector d? Is that always fixed? Or time-varying?
Wonderful Talk! Thanks! I have a question from slide 6, do you have any mathematical explanation/framework of the terminologies ''prediction'' and ''trust'' in this context?
Now i see why they always recommending #haxtechie01 on IG you are the main boss thanks for the successful Unlock
Thank you for doing such a great seminar!
Thanks for sharing the recording here.
Big thank you Sarab sir for conducting these webinars. These are eye opening use cases and make us aware about the depth of great work going around on this subject. Would love to listen to your journey as well as you have experienced alot of these advances in this technological domain. Many thanks!
Challenges for ML at scale: 1. Fairness Survey hindi -> data biased -> algo inherent bias -> discriminated outputs May'19 GDPR by EU. We don't know if those bias variables exists in dataset. California vs NY gender vs car color - correlated. Race-income Race-education How algo can be less discriminatory. Ethical data modelling. Use less demographic variables Ai fairness 360 degrees toolkit detects bias variables 2. Reliability How robust are the results. DL ability to predict with higher accuracy has risen 2012 onwards Model shd be generalized enough Training algo robustness Adversarial impact /attacks Panda image + noise=junk Have enough training representation, so as to easily side line adversarial data. More unstructured data today. Comf matrix Softmax func 3. Explainability Explainable AI ML is not a blackbox, we shd be able to explain the reason for outcome. Answerable to auditor. FAT US millitary research wing XAI explainable AI Writting letters -> automatic classification based on pincodes 85-90% accurate Friend past 5 yrs pics, can we predict what next? Hill station predicted Sea beach actual How? Which variable is impacting model predictions? LIME LOCAL INTERPRETABILITY LOCO leave one covarient out ELI5 Explain like I am 5 follows LIME SHAP sharply Debugging model predictions becomes easy. Helps in accountability of our model. Investment -> take decisions -> a risk Can you pls share email resources?? Accuracy Fairness training set Reliability attacks Explainability key decision makers Textual data Is GDPR truly achievable? Not fully. What is sensitive info and what is not? Behavioral data? Customer centric data? Personally identifiable data? Information asymmetry Drivers info Mostly male drivers Gender for female can be biased Use less survey driven data Survey vs actual huge gap