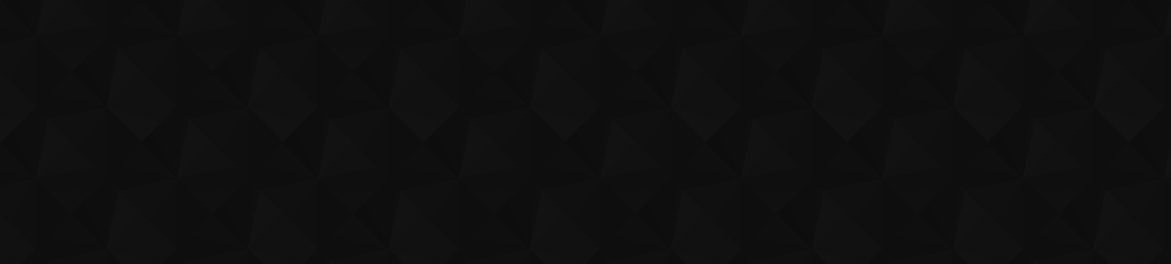
- 91
- 491 029
Tucker Balch
เข้าร่วมเมื่อ 13 ส.ค. 2010
วีดีโอ
model 2 6
มุมมอง 4576 ปีที่แล้ว
The reward includes a positive reward for moving horizontally close to the region above the landing zone.
Model 1 1
มุมมอง 1766 ปีที่แล้ว
At the beginning of training the learned model does not do very well.
Lucena/Nasdaq: Multi-Expert Factors Trading Model
มุมมอง 9957 ปีที่แล้ว
Tucker Balch of Lucena Research and Oliver Albers of Nasdaq introduce the technology forming MELS.
How Lucena builds successful strategies, Part III: Test and deployment
มุมมอง 6127 ปีที่แล้ว
Lucena's Chief Scientist Tucker Balch, PhD and Lead Quant Developer Taylor Korf discuss process Lucena follows to develop and deploy trading strategies.
How Lucena builds winning strategies: Part II, Backtesting
มุมมอง 6137 ปีที่แล้ว
Lucena's Chief Scientist Tucker Balch, and Lucena's top quants discuss our backtesting methodology.
TieBreaker & BlackDog: Two systematic trading strategies
มุมมอง 7558 ปีที่แล้ว
TieBreaker & BlackDog: Two systematic trading strategies
New Python-based 3D Multi-agent Simulator
มุมมอง 1.3K9 ปีที่แล้ว
New Python-based 3D Multi-agent Simulator
Lucena Webinar: GoldDigger II: Scaling Up Genetic Algorithm-based Strategies
มุมมอง 1.8K9 ปีที่แล้ว
Lucena Webinar: GoldDigger II: Scaling Up Genetic Algorithm-based Strategies
Lucena Webinar: Genetic Algorithm-based Event Discovery
มุมมอง 3.2K9 ปีที่แล้ว
Lucena Webinar: Genetic Algorithm-based Event Discovery
The 60/40 Portfolio is Dead: Why Dynamic Portfolios are Better
มุมมอง 2.9K10 ปีที่แล้ว
The 60/40 Portfolio is Dead: Why Dynamic Portfolios are Better
Computer labeled interactions between ants
มุมมอง 20210 ปีที่แล้ว
Computer labeled interactions between ants
Lucena Webinar: Best Strategies of 2013
มุมมอง 1.1K10 ปีที่แล้ว
Lucena Webinar: Best Strategies of 2013
40:17 i spat my food out
What is this toy called?
8 years later and watching this two days after a Presidential Debate with Trump in it. Time is a circle
Another 7 year old video courtesy of OMSCS
the audio is so bad, im having difficulties understanding the material..
Really wish Georgia tech would invest a tiny amount redoing these 7 year old videos with outdated requirements
i was 14 years old when this was posted lol
I was 20. Now I feel old 😆
ml4t gang wya ?
Ml4t gang wya ?
money man
connection issue sucks sorry.
subscribed !
For future OMSCS students this the the first little bit of this video: th-cam.com/video/2e2Yr-Bpo-w/w-d-xo.html
Yo, someone needs to re-record this lecture on a microphone instead of a potato. It's been six years, tf am I paying your salaries for? @instructors
These requirements require a law degree to understand...
🤣
The audio is horrible
58:54 for iterative vs final speed comparison
legend
here's ur crown
Aged like milk
That is indeed a giant log
` 3:15 - Lecture Starts 3:26 - Structure of orders.csv 5:00 - Dataframe: Prices 8:57 - Dataframe: Trades 15:20 - Dataframe: Holdings 21:10 - Dataframe: Value 23:02 - Dataframe: Portfolio Value 24:10 - Q&A Section 33:36 - Leverage 36:24 - Q&A Section 39:55 - Alternative Approach
This is an excellent explanation as to the risk-reward system that portfolio optimization realizes. The beauty is that what you do is manage the risk reward on the portfolio so that when it does get off of the tangent line, you rebalance back to the upper part of the line or on the line. That is the idea of when to rebalance. The idea is to maintain an optimized portfolio and if it shifts off of that upper part of the line, then the portfolio is out of balance and needs to have the right cocktail of efficient asset allocations.
This was a great lecture for helping build intuition about decision trees. Thank you Prof Balch! You're awesome!
this is challenging to watch. audo is poor and cannot discern what is on the presentation slides. just take this video down.
skill issue
#AMC #BBIG
Dyna at 42:00
king
Incredible explanation, so helpful!
I would really like to believe that GaTech grad students would be smarter than this bunch.
Mr. Abercrombie's redemption 33:42
2:15 - [START HERE] code structure and templates 4:45 - StrategyLearner API 6:15 - addEvidence() parameters and behavior 7:10 - testPolicy() parameters and behavior 9:30 - Evaluation rubric 12:25 - Implementation of StrategyLearner 17:00 - how to frame trading as a reinforcement learning (RL) problem 19:55 - What defines the State (in this problem)? 21:15 - What are the Actions? 27:35 - What is the Reward? 28:30 - Should the reward be delayed (long-term, i.e. cumulative return) or frequent (short-term, i.e. daily return)? 31:15 - Balch assumes we are not using the Transition Matrix (i.e. no Dyna-Q) 33:45 - How to represent the State? 38:15 - StrategyLearner addEvidence() pseudocode 44:20 - Q: How do we define convergence? 50:45 - testPolicy() pseudocode 52:15 - adding missing line in addEvidence() pseudocode 54:05 - discussion of short-term vs long-term rewards 58:00 - daily return rewards depend on state (long, short, none). If holdings are none, you should get no rewards 59:15 - should we use Dyna-Q? Dyna is not recommended, because we want to minimize runtime, but it should reduce the number of trades 1:00:30 - [END OF LECTURE]
link to the slides please
You are a great teacher Prof. You make it easy for us. Thank you!
0:00 - [START OF BACKTESTING LECTURE]. Goal of next project: create a classification model that decides between BUY, SELL, or DO NOTHING. 3:20 - How to backtest this strategy. 5:50 - Trading on adjusted close considerations 6:30 - Look back a constant (recent window) amount of time 7:00 - Roll-forward cross-validation 9:15 - Done w/ overview of project. How can backtesting be misleading? 10:15 - #1: In-sample backtesting 11:45 - How to avoid #1? 13:00 - #2: Survivor (survival / survivorship) bias 17:10 - Question 17:58 - How to avoid #2 19:20 - [Professor skips #3]. #4: Ignoring market impact. 24:00 - Question: What if you traded less (i.e. a lower volume of trading)? Answer: It might work, but a strategy may not be profitable below a certain volume. 26:10 - End of backtesting lecture. Start of Q&A. 26:20 -Question: Are there any legal issues with trading to affect a price? Answer: It depends. Pump-and-dump is illegal. 28:00 - [END OF BACKTESTING LECTURE] 29:30 - [START OF VECTORIZATION LECTURE] 31:50 - Description of Price / SMA ratio 32:20 - Description of Bollinger Bands Percent (BBP) 33:00 - Description of Relative Strength Index 34:35 - Description of trading strategy 35:20 - 'Indicator Details' 35:40 - 'Iteration: The Easy Way' 38:55 - Iteration continued: Calculate Relative Strength & RSI <-- this is hard to vectorize 39:45 - Iterative code to apply the indicators and make a trading decision 41:20 - Iteration is slow. What are the problems with this? 42:20 - Start of how to vectorize this code. Suggested strategy for converting iterative algorithm to vectorized code. Bottom line: work iteratively ;) 45:05 - Vectorize initializing arrays with zeroes 45:20 - Vectorize looping over symbol columns 46:08 - Vectorizing SMA loop 48:00 - Vectorizing Bollinger Bands loop 49:10 - Vectorizing Price / SMA 49:15 - Vectorizing RSI 50:10 - Vectorizing inner RSI loop 1 / 2 51:40 - Vectorizing inner RSI loop 2 / 2. How NumPy handles dividing by zero. 52:55 - Adding code to combine multiple vectors into one vector. df.cumsum() is key to handling sliding windows. 54:40 - Fully vectorizing RSI 55:00 - Simplifying trading strategy if-elif statements 55:45 - Problem with Price-SMA crossovers 57:10 - Vectorizing calculating results of entire strategy in one line only works because all arrays are the same size 57:40 - Dealing with NaNs in orders df 58:20 - Optimizing writing to orders file. Final result is 1000 x faster than iterative version. 59:30 - [END OF VIDEO]
Dude you're a legend!
02:00: lecture theme: how to build a decision tree 05:10: gap (skip) 05:40: decision tree example 08:09: tabular view of decision tree - use Numpy 2D array 9:00: if a factor value represents a leaf node, the associated SplitVal represents a y_pred value and not a split value 10:30: student question about binary tree efficiency 13:00: algorithm for building decision tree 15:00: decision tree algorithm (JR Quinlan) 16:30: IF base cases, RETURN row of data 17:00: description of recursive algorithm 22:40: student questions 24:50: how to determine the "best" feature 29:10: student questions • Check which axis your tree rows are being appended to each other 30:45: ndarray representation of decision tree 33:30: Use highest absolute value of correlation to select optimal 'splitting' factor • Numpy has a built-in correlation method • Split value should be the median value 35:30: gap (skip) 36:20: ndarray representation of decision tree (cont.) 39:00: when multiple factors have the same correlation value with y, use a deterministic approach to select the "best split factor" (e.g. the lowest indexed factor) rather than a random approach -- this will make results more reproduceable because we have to calculate correlation or entropy, Gini Impurity, etc. 43:30: which steps in JR Quinlan decision tree algorithm are the most computationally expensive? 44:30: A: determining the best feature to split on 45:30: Most time-intensive parts of the JR Quinlan Decision Tree algorithm are: 1. Determining the best feature to split on 2. Calculating the median for SplitVal • Random trees can help with this 46:30: Random Tree Algorithm (A Cutler) 50:30: You can create a random tree by randomly selecting features of the tree OR by creating a tree with randomly selected subsets of the training data 52:30: Strengths & weaknesses of Decision Tree learners • Cost of learning: decision trees more expensive than parametric or KNN • Cost of querying: Linear regression is fastest. But decision trees are faster than KNN • Decision Trees: No need to normalize data
11:00: Factors (X1, X2, …, XN) 15:00: describing decision tree structure 16:30: theme of lecture: how to use a decision tree if you have one 19:00: Decision tree API 22:00: introduce UCI wine dataset 24:00: Discussion of wine dataset 27:00: abstracted model dataset with X_n factors and y label 30:30: how to use a decision tree 32:00: gap while drawing decision tree (skip) 36:30: example decision tree with quiz 41:00: answer to quiz 44:00: in-sample vs out-of-sample testing --> overfitting • y_predict = model's predicted y value • y_test = true value (out-of-sample test) 48:00: implementing decision tree as ndarray 1:00:00 - Saving tree data to an ndarray - use relative indexes for children • Think about binary tree indexing parent & children nodes 1:01:40: Q&A • Fastest time-to-query: linear regression --> decision tree --> KNN • Max time-to-query for decision tree: log_2 (n) where n = number of nodes (assuming a balanced tree) • Decision trees are slow to learn/train --> it takes a lot of computational time to build a decision tree 1:07:00: end of lecture
very helpful for the midterm, thanks!
Extremely helpful
Thank you for your service my man
🫡
Blue m
Taking this course as my first semester course and you are hands down MY FAVORITE Professor! You making learning so fun and do a phenomenal job. Thank you so much!
3:30 - Key Requirements: Function should take in file name, return pandas dataframe indexed by dates, with values of portfolio on each date 6:20 - Format, contents of orders.csv 9:45 - Student question: shorting 10:20 - What kind of orders are these? --> "market-on-close" orders = adj close price for the day 13:10 - Expected input and required output 15:10 - How to get data for all dates between earliest date and most recent 16:35 - Step 1: Read in orders file --> prices dataframe 19:55 - Add rightmost 'cash' column 21:50 - What should the values of 'cash' be? $1.00 <-- Assume the value of one share of cash is one dollar 23:40 - Copy 'prices' dataframe to 'trades' dataframe 25:50 - How to build 'trades' dataframe? Initialize values for all columns right of dates to zeroes 26:20 - How to populate Trades: step through orders file row by row and record buys and sells. Record changes in cash column to reflect transactions 27:50 - How to calculate Cash values: +/- (Number shares in order * share price on order date) <-- (+/-) depending on buy or sell 36:00 - 'Holdings' dataframe records equities and cash amounts in portfolio on each date 38:00 - Create Holdings dataframe by copying Trades, initializing columns right of date with zeroes, and recording the initial cash value in the first row of the Cash column 39:00 - How to populate Holdings: For each day in Trades dataframe, add previous day plus current day. First day (row 0) is an edge case. Consider row -1 to be initialized with zeroes, plus initial cash value 41:20 - How to populate day 2 (row 1): For each day, take corresponding row in Trades dataframe, add it to previous day/row in Holdings dataframe, and that is the current row of Holdings 45:30 - The core of the project is accounting for the value of your equities and cash on each day 46:30 - 'Values' dataframe. Copy Holdings dataframe and initialize with zeroes. This dataframe represents the value in dollars of each asset 47:40 - Values = x * y. x = ? y = ? Remember: in pandas, multiplication is a cell-by-cell operation 48:30 - Answer: Values = Prices * Holdings 50:00 - 55:55 - Technical error (skip) 57:50 - The final output should be: day-by-day what is the total value of the portfolio? 58:10 - How to calculate portfolio value? Add Value dataframe columns (row-wise sum, axis=1). 58:35 - No matter what you buy or sell on day 1, the portfolio value at the close of day 1 should be the same as the initial Cash value 1:00:30 - Question: Can the cash column be anything other than $1.00? No, not for this assignment. It is always $1.00 1:01:50 - How do you go from the orders file to the Trades dataframe without a for loop? You have to do a for loop. Step 1 requires a for loop 1:02:40 - Secret #1 (irrelevant as of Spring 2021 -- skip) 1:05:40 - Leverage (irrelevant as of Fall 2021 -- skip)
Life saver
Thank you!
You are doing God's work.
DT Learner Algorithm Explanation starts at 14:30 RT Learner Algorithm Explanation starts at 47:00
New toy
That is a great video
1:57 - Overview 6:00 - Constructor 6:05 - num_states / num_actions / qtable structure 7:09 - alpha 7:35 - gamma / robot motivation negative reward 9:50 - rar 11:00 - radr 11:52 - dyna 12:20 - verbose 12:37 12:47 - query(s_prime, r) 16:20 - querysetstate(s) 17:24 - question 18:30 - question 19:30 - question 23:26 - question 25:42 - navigation problem 32:30 - transforming the problem 34:25 - test code 40:30 - dyna 49:40 - dyna bonus 51:20 - question 54:00 - questions
thank you, super handy!
Thank you for the excellent content.
Great great content, I'm watching this 6 years later in 2021 still great, thanks.
I love these recorded lectures. A real breath of fresh air after 7 classes of Udacity :)
Don't get your hopes up about the secrets at the end of the video. They don't apply to current semesters of the class (at least in Spring 2021)
outstanding explanation great start to learn this stuff
So energetic and gives a great explanation, fantastic!
How was the correlation calculated ? around 28:30
using an in built excel function
If you already have rough idea on how decision tree works, I suggest you to jump to part 2 where the algorithm on how to construct the decision tree is being explained, to save your time. th-cam.com/video/WVc3cjvDHhw/w-d-xo.html As the sound quality in this video is not great, it is hard to grasp exact words from the professor and I end up guessing what is it about from the whiteboard.
By far the most easily digestible presentation on portfolio optimization. Thanks!