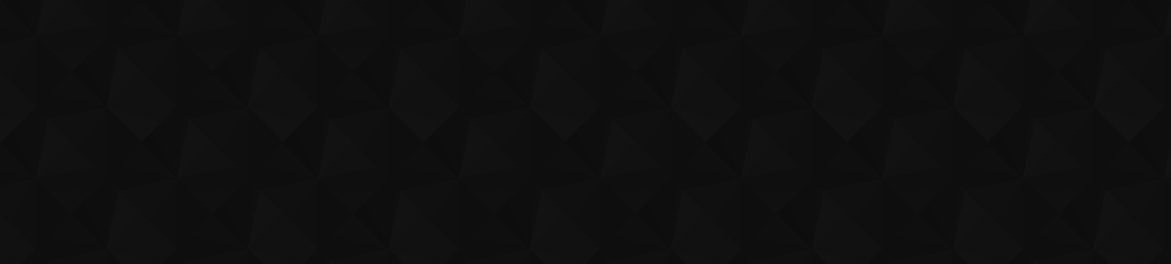
- 52
- 59 003
Optimized AI Conference
เข้าร่วมเมื่อ 3 ก.ค. 2021
The Optimized AI Conference (OAI) is practitioner first machine learning conference that experts and researchers from the companies share their best practices for generating revenue with AI.
If you are responsible for generating revenue with AI both on the practical and leadership level, there will be content that you would enjoy.
If you are responsible for generating revenue with AI both on the practical and leadership level, there will be content that you would enjoy.
How ChatGPT can change your life - Joel Grus
Joel compared the paradigm shift brought about by ChatGPT to earlier advancements in NLP, noting its broader societal impact. The talk touched on the unique capabilities of ChatGPT in understanding and generating responses, distinguishing it from traditional search engines.
✅ Connect with Joel: www.linkedin.com/in/joelgrus/
✅ Connect with Southern Data Science Conference on LinkedIn - www.linkedin.com/company/southerndatascienceconference/
✅ Connect with Southern Data Science Conference on Twitter:
southerndsc
✅ Connect with Joel: www.linkedin.com/in/joelgrus/
✅ Connect with Southern Data Science Conference on LinkedIn - www.linkedin.com/company/southerndatascienceconference/
✅ Connect with Southern Data Science Conference on Twitter:
southerndsc
มุมมอง: 503
วีดีโอ
Applied ML with KerasCV & KerasNLP - Shilpa Kancharla
มุมมอง 211ปีที่แล้ว
Shilpa highlights the challenges of keeping up with the ever-evolving landscape of machine learning and AI, particularly in training state-of-the-art models. She emphasizes the significance of KerasCV and KerasNLP in simplifying complex tasks, such as computer vision and natural language processing, by reducing computational costs. ✅ Connect with Shilpa: www.linkedin.com/in/shilpa-kancharla-301...
Reinforcement Learning for Music Recommendations - Surya Kanoria
มุมมอง 320ปีที่แล้ว
In this insightful presentation, Surya Kanoria, a Senior Machine Learning Engineer at Spotify, delves into the fascinating realm of reinforcement learning for music recommendations, focusing specifically on automatic playlist generation. Surya highlights the importance of providing users with a unique, personalized listening experience, considering both foreground and background music consumpti...
Emerging Architectures for LLM Applications - Raja Iqbal
มุมมอง 268ปีที่แล้ว
Raja explores three key approaches: training a model from scratch, fine-tuning a foundation model with custom data, and the innovative concept of "in-context learning." He emphasizes the significance of data engineering in using vector databases, indexing, and semantic caching to enhance LLM applications. The talk covers common design patterns, challenges in real-world scenarios, and the import...
Evolution of Ads Ranking at Pinterest - Aayush Mudgal
มุมมอง 291ปีที่แล้ว
Aayush discusses the transition from linear models to gradient-boosted decision trees, outlining the limitations and challenges faced during this phase. The talk further explores the adoption of neural network-based approaches, emphasizing the need to replace nonlinear transformations. Ayush introduces Pinterest's AutoML architecture, a deep multitask model that streamlines feature engineering ...
An approach to ML Project architecture in the age of generative LLMs - Marcelle Bonterre
มุมมอง 82ปีที่แล้ว
Marcelle Bonterre delves into various optimization techniques, including pruning, quantization, TorchScript, FX system, module fusion, and more. Marcelle highlights the availability of tools like TorchPrep, AWS Neuron, Intel Neuro Compressor, OpenVino, nn Compression, and others that automate and streamline the optimization process. He also touches on the benefits of using PyTorch's better tran...
Relevance in the Age of Generative Search - Trey Grainger
มุมมอง 159ปีที่แล้ว
The talk covers the nuances of generative search, discussing its potential to create new information dynamically, but also addressing the pitfalls of biased results and hallucinations. Trey emphasizes the importance of hybrid approaches that combine traditional search techniques with emerging vector database technologies. ✅ Connect with Trey: www.linkedin.com/in/treygrainger/ ✅ Connect with Sou...
Building AI Co-Pilot for Effective Marketing using Multimodal Knowledge-infused Learning
มุมมอง 90ปีที่แล้ว
Ugur introduces the knowledge-infused learning approach, showcasing the role of knowledge graphs in mitigating content ambiguity and ensuring coherent contextual understanding. He explores ongoing projects aimed at providing marketers with AI tools that integrate social and behavioral insights for optimal content creation. Join Ugur Kursuncu on this enlightening journey into the future of socia...
Paradigm Shifts and Enterprise Concerns Around Large Language Models - Reshma Lal Jagadheesh
มุมมอง 168ปีที่แล้ว
Reshma begins by providing an overview of traditional chatbot architectures and then highlights the revolutionary changes brought about by LLMs. She discusses how LLMs have the potential to handle natural language understanding, dialog management, and natural language generation in one unified system, transforming the way virtual assistants and chatbots operate. Throughout the presentation, Res...
LLM and Vector Databases: Concepts, Architectures, and Examples - Sam Partee
มุมมอง 760ปีที่แล้ว
In this engaging presentation, Sam Partee, a Principal Applied AI Engineer at Redis, dives into the world of Language Model (LLM) and Vector Databases. He discusses various concepts, architectures, and provides practical examples to help the audience better understand these complex topics. ✅ Connect with Sam: www.linkedin.com/in/sampartee/ ✅ Connect with Southern Data Science Conference on Link...
Build vs. Buy: What's Best for your Business - Alex Vayner
มุมมอง 139ปีที่แล้ว
Alex Varner, Managing Partner at Martingale Insights, delves into the critical decision-making process of whether to build or buy solutions for your business. Drawing from his extensive background in mathematics, computer science, and consulting across various industries, Alex provides a structured approach to help organizations navigate this fundamental choice. Throughout the talk, Alex addres...
Beyond Labels: Leveraging Deep Learning and LLMs for Content Metadata - Jaya Kawale
มุมมอง 192ปีที่แล้ว
Jaya Kawale explores how machine learning plays a fundamental role in enhancing three key pillars of Tubi: audience, content, and ads. She discusses how machine learning helps in delivering personalized content recommendations, understanding the intricacies of different genres, and optimizing ad placements for an enhanced user experience. The presentation also introduces the concept of "Genre S...
Suraj Subramanian: Distributed Training in PyTorch - Paradigms for Large-Scale Model Training
มุมมอง 219ปีที่แล้ว
Subramanian's talk promises to serve as a cornerstone for anyone interested in the field of machine learning, offering invaluable insights and a deeper understanding of the principles that drive the development of cutting-edge AI models. Whether you're a researcher, developer, or enthusiast, this presentation at the SDSC Conference is a golden opportunity to gain a solid grasp of the critical e...
Memory Matters: Improving Chatbots with Long-Term Memory at Pinecone - Roie Schwaber-Cohen
มุมมอง 154ปีที่แล้ว
Discover the importance of effective chunking and embedding methods in chatbot design and learn how these techniques can optimize responses and enhance user experiences. Roie also discusses the critical issue of hallucination and shares valuable insights into fixing and mitigating this challenge. Join us for an enlightening session with Roie Schwaber-Cohen, a Staff Developer Advocate at Pinecon...
Why VCs Say No and How to Get a Yes - Garnet Heraman
มุมมอง 115ปีที่แล้ว
Garnet S. Heraman, an esteemed Venture Capitalist at Aperture VC, offers invaluable insights into the world of startup investments and entrepreneurship. The talk also delves into the challenges that startups often face, such as overhyping their ventures and mismanaging their finances. Heraman provides practical advice on how to avoid these pitfalls and maintain a lean, customer-focused approach...
Generating Interest in Gen AI: Debunking the myth with Real World Business Examples - Laura Edell
มุมมอง 107ปีที่แล้ว
Generating Interest in Gen AI: Debunking the myth with Real World Business Examples - Laura Edell
LLMs for Everything and Everyone! - Sebastian Raschka - Lightning AI
มุมมอง 7Kปีที่แล้ว
LLMs for Everything and Everyone! - Sebastian Raschka - Lightning AI
Lessons Learned Applying Large Language Models in Healthcare - David Talby
มุมมอง 178ปีที่แล้ว
Lessons Learned Applying Large Language Models in Healthcare - David Talby
Embracing SQL in Data Science Workflows - Aaron Richter
มุมมอง 2532 ปีที่แล้ว
Embracing SQL in Data Science Workflows - Aaron Richter
From Model to Microservice - MLOps at Scale - Joshua Goldstein
มุมมอง 2942 ปีที่แล้ว
From Model to Microservice - MLOps at Scale - Joshua Goldstein
Real Time ML: Challenges and Solutions - Chip Huyen
มุมมอง 1.5K2 ปีที่แล้ว
Real Time ML: Challenges and Solutions - Chip Huyen
Monetizing Data Science - Justin Dale Collins
มุมมอง 1152 ปีที่แล้ว
Monetizing Data Science - Justin Dale Collins
How Feature Stores save you from the Mundane - Rosie Min
มุมมอง 1962 ปีที่แล้ว
How Feature Stores save you from the Mundane - Rosie Min
Machine Learning at Tubi: Powering Free Movies, TV and News for All - Jaya Kawale
มุมมอง 2862 ปีที่แล้ว
Machine Learning at Tubi: Powering Free Movies, TV and News for All - Jaya Kawale
Keras: Deep Learning Made Easier - Divya Sreepathihalli
มุมมอง 3.1K2 ปีที่แล้ว
Keras: Deep Learning Made Easier - Divya Sreepathihalli
Building a Security Analytics Platform using Federated Machine Learning
มุมมอง 712 ปีที่แล้ว
Building a Security Analytics Platform using Federated Machine Learning
Towards Responsible AI in Production for Consumer Product Organizations
มุมมอง 6242 ปีที่แล้ว
Towards Responsible AI in Production for Consumer Product Organizations
Vector Search and a Conversation with your users - Marcus Eagan
มุมมอง 3532 ปีที่แล้ว
Vector Search and a Conversation with your users - Marcus Eagan
Nicely Explained!
SingleStore DB has hybrid search capabilities.
sickkkkk
I'm also nice to my chat gpt sessions, but equally abusive when I fail to prompt it well
no questions usually mean - you know what.. examples of the slides are terribly biased, not sure how that can be effective campaign or product
what exactly is a parameter in llm? what do you mean when you say this model has 1 billion parameters?
Parameters are numerical values that basically translate into model performance/behaviour, learned during the training process. Those are needed to better capture the patterns and relationships in the data. More = higher complexity = ability to perform more complex tasks. As a rough estimate, for every 1 billion data points, you can expect approximately 10 billion parameters. Where data point is a unit of information that is used to train a model. Let's say we want to train a model to understand positive and negative reviews, so data point will be: one review (tasty pizza and great service) + sentiment (positive). Example: weights. LLM is a neural network - a lot of connections. How to figure out the influence of one neuron on another? - weights. He mentioned another parameter called "temperature" - controls the randomness of the generative process. Temperature is usually used for the entire model but for some cases, multiple temperature values could be used. You can adjust "biases" to eliminate (probably partially if data set is like that) offensive or harmful model responses.
@@kutervthank you for the insanely detailed explanation, lets say you wanted to train a model on reviews since you mentioned it. How many parameters do you need or does this somehow get set by the system while training
Nice
nothing new, just recycled existing information on this topic, very disappointing ...
Absolutely amazing! Thank you so much for this.
🎯 Key Takeaways for quick navigation: 00:29 🤖 Sebastian Raschka is the lead AI educator for Lightning AI and a former professor of Statistics. 00:43 🤔 LLMs (Large Language Models) promise to make us more productive by speeding up tasks like writing emails and coding. 01:52 🦾 Sebastian discusses the motivation behind caring about LLMs, including staying updated with news and the potential for increased productivity. 04:09 🎛️ LLMs like ChatGPT go through three main stages: pre-training, supervised fine-tuning, and alignment. 07:37 💡 The pre-training and fine-tuning stages involve next word prediction tasks, but the format of the data sets and instructions differ. 10:09 🤖 LLMs (Large Language Models) go through a three-stage process: pre-training, fine-tuning, and alignment. 11:31 🦾 Reinforcement Learning from Human Feedback (RLHF) involves sampling prompts, having humans rank the responses, training a reward model, and refining the model using proximal policy optimization. 13:21 💡 When it comes to using LLMs, there are five main ways: everyday tasks, pre-training and prompting for specific domains, fine-tuning on specific data, fine-tuning and prompting for code-related tasks, and training custom models. 16:36 👥 Bloomberg pre-trained their own LLM on general data and finance-specific data to generate a proprietary database format and finance news headlines. 18:13 🐍 Meta AI (formerly Facebook) trained the Code LLM by pre-training a model, further training it on general code data, fine-tuning it on python code, and iterating through various fine-tuning stages to create models like Code Llama. 19:07 👥 Open source LLMs provide access to log probabilities, allowing for analyzing the confidence of the model and ranking responses. 21:24 🤖 Open source LLMs provide privacy and control as the data never has to leave the computer and can be modified as desired. 23:19 👍 Open source LLMs offer full customizability and allow for experimentation and modifications to the model. 24:30 💡 Open source LLMs do not change unless desired, which can be an advantage or disadvantage depending on the need for updates. 25:40 🔌 Running open source LLMs requires access to hardware and some additional work in terms of cloning repositories and downloading weights. 30:02 👥 Open source models like Free Willy Falcon, Bikuna, PhysioStable, Code Llama, and Lit GPT offer freely available weights and a hackable, customizable codebase. 31:27 🏢 Fine-tuning smaller models can achieve better performance on specific tasks than using larger general models like GPT-3 or GPT-4. 32:49 💡 Fine-tuning models are task-specific and useful for solving specific business problems, while prompting models are more general-purpose. 34:40 ⏱️ Parameter-efficient fine-tuning techniques like adapters and low-rank adaptation (Laura) can save a significant amount of time compared to full fine-tuning. 37:12 🌟 Future trends for LLMs include the mixture of experts approach, multi-modal models, and LLMs for specific domains like protein-related tasks. 39:33 🎯 Non-transformer large language models like RWKV, hyena hierarchy, and retentive Network offer alternative approaches to language modeling that are worth keeping an eye on. 39:49 🔄 There are alternatives to reinforcement learning-based fine-tuning, such as relabeling data in hindsight and direct policy optimization, which show promising results and may simplify the process. 41:22 🌐 The performance of fine-tuned models can vary based on the specificity of the domain, with the potential to outperform larger pre-trained models in smaller, more specific domains. 41:09 📚 Sebastian Raschka stays updated on new research papers and frequently discusses them on his blog. He is also involved in developing the open-source repository Electricity for loading and customizing LLMs. 41:40 🎙️ There is no size limit for the domain when it comes to outperforming pre-trained models with fine-tuned models. The performance can vary based on the specific task and the domain covered. Made with HARPA AI
1 minus cosine similarity? Why?
You're calculating the distance between the vectors. when normalized, 1-distance = how similiar the vectors are.
Good but, how does it compare against the other vector DBs?
Can we search through the documents "which targets specific information"? Suppose, I want to search for the articles which talk about "young males in their 20s". Now, that doesn't necessarily look for that phrase because it is unlikely that it will be the case. Then how would I search like that? Should I pass metadata? How to pass additional information that can be used to filter the documents for the retrival?
Vector addition is high level term?
Thanks for a presentation
Come on people, his jokes weren't that bad.
yea they were
Great speaking skills by Sam. Vector databases are going to partner with LLMs beautifully.
Great explanation
ai datab bases
I can't find the blog
@5:00, bottom of the slide
Is there a possibility if we can get the presentation deck?
Ol
Thanks for sharing this ! This presentation was very valuable. This 3rd generation view(past, present, future) raises a lot of questions in my mind. • What business areas(industry, agriculture, constructions, small business) we could apply the machine learning techniques to improve productivity ? • How to turn a vast amount of tabular data available into good resources(good raw material) for theses models? • How to acquire better quality, standardized and comparable data (e.g. sensors, transfer learning from dl models etc) ? Thank you all for sharing with us and congratulations Mr. Tunguz for this presentation.
Good Talk
One of the most undervalued projects! Happy to had/have the chance to know about it before it skyrockets!
Like bro!)
I loved this technical presentation! I've been using Presearch for a long time and have two nodes. The engine is already running very well! I invite everyone to try it.
Outstanding yes, thanks for your talk
Nice job Trey! Looking forward to seeing the rollout of additional node roles in the near future!
Outstanding presentation... Thanks Trey for an explanation that even a novice such as me can understand! : ) 💪💪💪
Amazing work Trey you are the best for Presearch, here I am migrating a node from RN jaja. Keep rocking