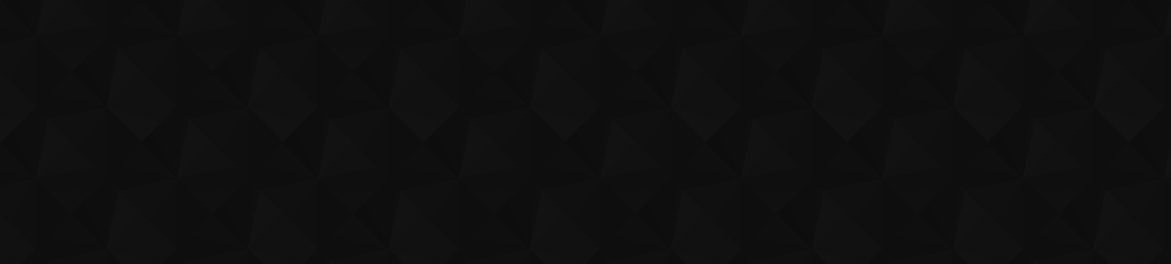
- 70
- 240 275
Zico Kolter
เข้าร่วมเมื่อ 7 ส.ค. 2012
Lecture 25: Computational Game Theory
Lecture 25: Computational Game Theory
This is a lecture video for the Carnegie Mellon course: 'Graduate Artificial Intelligence', Spring 2014.
Information about the course is available at www.cs.cmu.edu/~zkolter/course/15-780-s14/
This is a lecture video for the Carnegie Mellon course: 'Graduate Artificial Intelligence', Spring 2014.
Information about the course is available at www.cs.cmu.edu/~zkolter/course/15-780-s14/
มุมมอง: 3 173
วีดีโอ
Lecture 18: Computer vision 1
มุมมอง 6K10 ปีที่แล้ว
Lecture 18: Computer vision 1 This is a lecture video for the Carnegie Mellon course: 'Graduate Artificial Intelligence', Spring 2014. Information about the course is available at www.cs.cmu.edu/~zkolter/course/15-780-s14/
Lecture 23: Natural language processing
มุมมอง 4.9K10 ปีที่แล้ว
Lecture 23: Natural language processing This is a lecture video for the Carnegie Mellon course: 'Graduate Artificial Intelligence', Spring 2014. Information about the course is available at www.cs.cmu.edu/~zkolter/course/15-780-s14/
Lecture 20: Scheduling
มุมมอง 1.1K10 ปีที่แล้ว
Lecture 20: Scheduling This is a lecture video for the Carnegie Mellon course: 'Graduate Artificial Intelligence', Spring 2014. Information about the course is available at www.cs.cmu.edu/~zkolter/course/15-780-s14/
Lecture 22: Robotics 2
มุมมอง 83610 ปีที่แล้ว
Lecture 22: Robotics 2 This is a lecture video for the Carnegie Mellon course: 'Graduate Artificial Intelligence', Spring 2014. Information about the course is available at www.cs.cmu.edu/~zkolter/course/15-780-s14/
Lecture 21: Robotics 1
มุมมอง 1.4K10 ปีที่แล้ว
Lecture 21: Robotics 1 This is a lecture video for the Carnegie Mellon course: 'Graduate Artificial Intelligence', Spring 2014. Information about the course is available at www.cs.cmu.edu/~zkolter/course/15-780-s14/
Lecture 19: Computer vision 2
มุมมอง 1.7K10 ปีที่แล้ว
Lecture 19: Computer vision 2 This is a lecture video for the Carnegie Mellon course: 'Graduate Artificial Intelligence', Spring 2014. Information about the course is available at www.cs.cmu.edu/~zkolter/course/15-780-s14/
Lecture 17: Reinforcement learning
มุมมอง 2.2K10 ปีที่แล้ว
Lecture 17: Reinforcement learning This is a lecture video for the Carnegie Mellon course: 'Graduate Artificial Intelligence', Spring 2014. Information about the course is available at www.cs.cmu.edu/~zkolter/course/15-780-s14/
Lecture 15: Probabilistic inference
มุมมอง 1.2K10 ปีที่แล้ว
Lecture 15: Probabilistic inference This is a lecture video for the Carnegie Mellon course: 'Graduate Artificial Intelligence', Spring 2014. Information about the course is available at www.cs.cmu.edu/~zkolter/course/15-780-s14/
Lecture 16: Planning under uncertainty
มุมมอง 1.4K10 ปีที่แล้ว
Lecture 16: Planning under uncertainty This is a lecture video for the Carnegie Mellon course: 'Graduate Artificial Intelligence', Spring 2014. Information about the course is available at www.cs.cmu.edu/~zkolter/course/15-780-s14/
Lecture 13: Planning 2
มุมมอง 56910 ปีที่แล้ว
Lecture 13: Planning 2 This is a lecture video for the Carnegie Mellon course: 'Graduate Artificial Intelligence', Spring 2014. Information about the course is available at www.cs.cmu.edu/~zkolter/course/15-780-s14/
Lecture 14: Probabilistic modeling
มุมมอง 1.2K10 ปีที่แล้ว
Lecture 14: Probabilistic modeling This is a lecture video for the Carnegie Mellon course: 'Graduate Artificial Intelligence', Spring 2014. Information about the course is available at www.cs.cmu.edu/~zkolter/course/15-780-s14/
Lecture 11: Machine learning 2
มุมมอง 82610 ปีที่แล้ว
Lecture 11: Machine learning 2 This is a lecture video for the Carnegie Mellon course: 'Graduate Artificial Intelligence', Spring 2014. Information about the course is available at www.cs.cmu.edu/~zkolter/course/15-780-s14/
Lecture 10: Machine Learning 1
มุมมอง 1.3K10 ปีที่แล้ว
Lecture 10: Machine Learning 1 This is a lecture video for the Carnegie Mellon course: 'Graduate Artificial Intelligence', Spring 2014. Information about the course is available at www.cs.cmu.edu/~zkolter/course/15-780-s14/
Lecture 8: Constraint satisfaction
มุมมอง 88610 ปีที่แล้ว
Lecture 8: Constraint satisfaction This is a lecture video for the Carnegie Mellon course: 'Graduate Artificial Intelligence', Spring 2014. Information about the course is available at www.cs.cmu.edu/~zkolter/course/15-780-s14/
Lecture 2: Intelligent agents and paradigms for AI
มุมมอง 4.5K10 ปีที่แล้ว
Lecture 2: Intelligent agents and paradigms for AI
Lecture 24: Future Directions in Smart Grid Research
มุมมอง 1.3K10 ปีที่แล้ว
Lecture 24: Future Directions in Smart Grid Research
Lecture 22: LQ Stochastic Control, MDPs
มุมมอง 1.3K10 ปีที่แล้ว
Lecture 22: LQ Stochastic Control, MDPs
Lecture 20: Stochastic systems, PID control
มุมมอง 1.3K11 ปีที่แล้ว
Lecture 20: Stochastic systems, PID control
Lecture 18: Control examples, dynamical systems
มุมมอง 1.4K11 ปีที่แล้ว
Lecture 18: Control examples, dynamical systems
What of we took H=1 do we compute a lot of inuts so we have a smooth control?
Awesome video sir! Thank you!
The claim that the separation principle does not hold for POMDPs (15:00 - 16:00 in the video) is false. The belief state (conditional distribution of the present state based on the history of measurements) is a sufficient statistic. As pointed out in the video, you can update the belief in a recursive fashion (a generalized version of the Kalman filter). The principle of optimality (Bellman equation) still holds and it's possible to show that you can write it in terms of the belief state rather than the full measurement history. Consequently, the optimal action is a function of the belief. This is not an approximation. The separation principle holds for POMDPs. What does not hold in the POMDP case is certainty equivalence. The optimal POMDP policy will in general be different from the optimal MDP policy applied to the MMSE estimate of the state (the expected value of the belief). It's only in the LQG case that this works. The reason people say "POMDPs are hard" has nothing to do with the separation principle. There are two reasons: 1) Doing belief propagation in continuous state/action/measurement space is intractable. You would have to store the belief as a conditional distribution, which is easy to do in the LQG case where you know the belief will be Gaussian, but in general you would have to store some function that does not have a closed-form expression. Likewise, you can do dynamic programming, but again you have to store the value function, which is easy in the LQG case where you know it will be quadratic, but is difficult in general. There are various approaches for approximating the belief propagation and dynamic programming steps, which is what is done in practice to overcome these difficulties. 2) You can find the exact POMDP policy if the state, action, and observation spaces are finite and discrete. Then, your belief and optimal policy can be stored as matrices and implemented as lookup tables. Unfortunately, this is only practically useful for very modest POMDPs due to the curse of dimensionality.
wow thanks for the insight.
Starts at 3:30
hi , what is the difference between UC and Day- ahead? please
Great Work Sir Thanks Lot of Sir
thank you very much!!!, this will be helpful for my future master degree thesis in MPC
Thanks for the lecture. Please you have code MatLab or Simulink file examples
BORING!
Power flow begins 31:26
Is there a set of notes? Thanks
You are favourite
Great lecture. I have one persisting question though. How do you understand a model with many free parameters?
Very illuminating lecture. You put things in very nice mathematical ideas like convex functions and have made an important point about the linear and non-linear regression using features.
How did you plot those cool rotating 3D scatter plots?
I tried to convince myself that if f(x) = Ax then the Jacobian of f(x) is just A 59:00 by using the first example. I did it out on paper and it didn't work out so I tried it using MATLAB and got the same answer. Take a look imgur.com/a/Nlyf1BZ please let me know what I did wrong. I found an A matrix that works but it is not the Jacobian of f(x)… imgur.com/a/aDt668A
your lecture videos are great by the way :) thanks for sharing
please suggest a book you are following
32:55 How is fixing a function actually better than erasing it entirely? They both deliver us globally non optimal solution
for the quiz, machine learning because the robot has to be capable to learn the preferred coffee quantity for each one in the office, I like a big and full cup, no sugar. good robot. thanks Zico.
convex optimization at 50:20
THANKS!!!
Matrix Calculus start at 48:50
thx, very helpful
REALLY HELPFUL
Nice! Can you comment on how you made the 3D animated (rotating) graph?
This was really helpful.
Greetings, are there any plans to upload the Spring 2016 iteration of this course please, as you cover Deep Learning in it. Thank you for sharing these lecture video
Thank you! very helpful
Hello, Zico, I'm working on robot scheduling problem for automated wet station in semiconductor process. I want to learn how to use MILP concept to solve this problem, I've known all the constraints, but I don't know how to start with the algorithm. So how can I start to learn the MILP codeing for the very beginning?
Sunh Hao Hi sunh were you able to get any information on MILP??
The instruction is great Can't wait to watch more videos about control from this instructor Great job
Agreed
Hey, quick question about the objective function for the Sudoku. Are we actually optimizing with respect to this objective function or is it just used to "sort" the branches? If we are optimizing with respect to it, how do we do it? (As the function isn't linear).
They don't care about the value of the objective function. They just keep branching, then relaxing to solve the linear programming (LP), and go down the first branches that are feasible, then branch again + relax again + LP again until all binary variables are integral. The use of an arbitrary objective function is that the linear programming of the relaxed problem can be solved. A nice objective function makes LP solve faster.
Hello Mr.Kolter, Could you please advice me for good book talk about power flow?
Thanks. In many ways an excellent video, however in the beginning you talk of the magnet as if it has one pole. Later at about 43 min you in fact refer to a "one pole" magnet! AC is produced because when the field from the North pole cuts the conductor, current flows in one direction and in the opposite when the South pole comes around (load connected). The min number of poles in the generator is 2 and it must rotate at 3600 rpm for 60 Hz. You mention this as an unreasonable speed, but in Europe the vast majority of thermal sets run at 3000 rpm and I expect many thermal set in the US run at 3600. Hydro sets are typically multi-pole and so run at much slower speeds. However the stators are wound so that the rotating field of the rotor is still in synch with the rotating field produced by the current flowing in the stator coils. Thanks for the info regarding how the generator voltage must be increased to deliver reactive energy to inductive loads, very useful explanation.
Thank you !!!! Really need this for my thesis!
I am eager to know what program you are using to view the waveform in your computer.?
QUCS
Thanks for the upload. I was reading up on kernel methods but struggling to understand parts of it, but this really helped clear a lot of my doubts regarding the topic.
it starts at @54:51. Great teaching.
the teacher is handsome and hot
Someone please give me the Power Presentation link.
Thanks for the lecture. It is not true, however, that MDPs only work for finite actions and states. Have a look at policy search RL methods, and stuff like Fitted Q-Iteration.
I don't think so, continuous MDPs can only be solved approximately. While the state variables can be continuous, the actions are almost always discretized for applying methods like Fitted Q-iteration and some function approximation is always incorporated to beat the curse of dimensionality in continuous state spaces.
Hi, can you help me, I have fitted a non linear model, but now I need to make a test of significance for every parameter of my non linear model, How should I proceed??, I estimate my parameters with non linear least squares
very useful thanks.
brutta linguaccia!!!!!!!!
I got a head-start into Computer Vision through the last two lectures.Very intuitive way of teaching and definitely not sleep-inducing. Thanks a lot for posting these!
Nice lecture.
if there anyone listening u talking, they probable all dead,u kill them
Lecture begins at 5:10.
Great Record. It helps me a lot to get the basic idea of non-linear regression. Thx